https://github.com/bartosz25/beam-learning
Real-time processing is most of the time somehow related to stateful processing. Either we need to solve some sessionization problem, count the number of visitors per minute etc. Not surprisingly Apache Beam comes with the API adapted to put in place the solutions to them.
Data Engineering Design Patterns
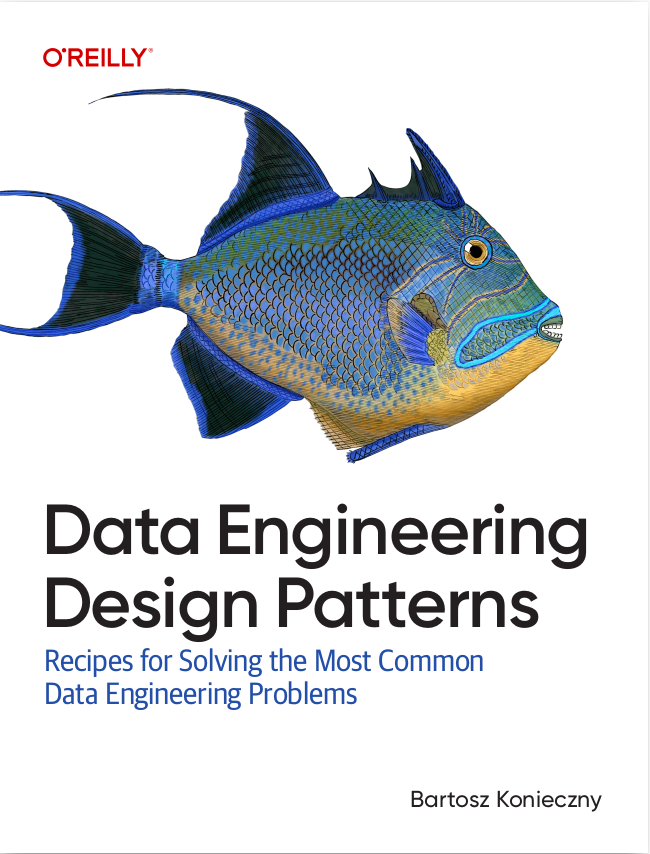
Looking for a book that defines and solves most common data engineering problems? I wrote
one on that topic! You can read it online
on the O'Reilly platform,
or get a print copy on Amazon.
I also help solve your data engineering problems 👉 contact@waitingforcode.com 📩
This post introduces the idea of stateful processing in Apache Beam. In the first section we'll discover the main points about this category of processing implemented in the framework. The second part focuses on the code and presents the objects involved in stateful applications. The last section gives some examples of stateful processing.
State in Apache Beam
The state in Apache Beam represents a situation occurred between 2 different points in the time. It can for instance group all events produced between this interval, aggregate, reduce them etc. Thus, the state is mutable since it evolves with time and new incoming elements. Technically speaking, Beam's stateful code allows each processing function to access persistent mutable state for each processed entry.
State storage
I've spent some time on looking for how the state storage is currently implemented in Apache Beam. The answer can be found in the org.apache.beam.sdk.state package and the implementations of the interfaces representing data structures accepted in stateful transform: MapState, SetState or ValueState.
The default implementation used by direct runner stores the state in memory. However, as Kenneth Knowles presented it during the Stateful processing of massive out of order streams with Apache Beam talk, Apache Beam is used to describe the computation pipeline and the state storage details rely on the runner executing the code.
For instance the state implementation in Spark relies on in-memory storage (stateTable attribute in org.apache.beam.runners.spark.stateful.SparkStateInternals). The objects are represented as an array of bytes.
Though it's quite possible that the state is stored in less volatile place, as for instance a key-value data store or simply on disk. It's up to runner to decide about the used strategy.
We can develop that short description and characterize the state with the following properties:
- state table - the mutable Beam's state can be thought as a table where the columns contain the state for each particular processing time. Since the state is bounded to the window, this particular processing time represents the window duration. The abstraction representing each sub-state is called state cell. For instance the state composed of state variable called page measuring the average time passed on this page by all users, could look like in the following table:
page passed time window1 index.html 200 window2 index.html 20 windown index.html 120 - mixed types - the state can contain different types of objects: scalar values, collections or maps. So grouped state is not limited to a single value. It can have multiple columns as in the table below where a column listing the visitors per page withing given window was added:
page passed time visits window1 index.html 200 ['visitor1', 'visitor2'] window2 index.html 20 ['visitor2'] windown index.html 120 ['visitor1', 'visitor2', ...] - grouped by key - the state works per key, i.e. it groups all events related to a particular key within a single state. In the previous example we can find the grouping per "page" property. Here we organize the state per user id key:
window state window1 user passed time pages user1 200 ['index.html', 'contact.html'] user2 120 ['cart.html', 'contact.html'] user3 50 ['index.html'] window2 user passed time pages user1 50 ['contact_form.html'] user2 120 ['search.html', 'products.html'] user3 50 ['contact.html'] - bounded to window - since the state cell is bounded to the window, it expires with the window ends. It's clearly illustrated in the table from the previous point where the list of visited pages is not persisted between 2 windows. However, if we're working with unbounded data and global window it may happen that the state will never expire without the use of timers (covered in of next posts)
- order not guaranteed - an important point to keep in mind is that, as for distributed processing in general, the order of processing is not guaranteed. So if our state is not commutative or associative, we'd not rely on arrival order and be able to handle out-of-order events
- no global and mutable state - the state applies only to keyed distributed collections and it makes an insight of another Beam's property. This framework doesn't support a global and shared mutable state that could be used for not-keyed datasets. In fact, this kind of state would be very difficult to manage in distributed environment
- shuffle - and obviously, as every key-based operation, the stateful one also can do an additional shuffle to collocate the keys on the same worker node. It brings another noticeable point - more keys are statefuly processed, more space Beam will need to store the state
State API
The state definition consists on the use of @StateId annotation on a private and final field being the instance of org.apache.beam.sdk.state.StateSpec<StateT extends State>. The state is identified by the name that must be unique through the transform. The state must be final because it can't be modified after the declaration. Similarly to its private access - the state must remain local to the transform. Regarding to the unique name, it's required to an efficient identification. This requirement to declare the state makes the processing fail fast, i.e. we don't even need to start the pipeline to see that it's incorrectly defined.
The identification of state by name is used later, when the state is attached to the processing (@ProcessElement-annotated) method. The same @StateId annotation is used to specify the state in this context as an additional argument. The arguments of @ProcessElement method are later analyzed by DoFnSignatures#analyzeExtraParameter(ErrorReporter methodErrors, FnAnalysisContext fnContext, MethodAnalysisContext methodContext, TypeDescriptor<? extends DoFn<?, ?>> fnClass, ParameterDescription param, TypeDescriptor<?> inputT, TypeDescriptor<?> outputT).
The type of state passed in processing method is not StateSpec but org.apache.beam.sdk.state.State. The state can be one of the following types:
- collection of values - the values computed for each key can be stored in a collection-like state as for instance: BagState<T>, MapState<K, V>, SetState<T>
- scalar value - for each processing key a single value is stored in the state. It's represented by ValueState<T> implementations.
- combine state - combines multiple input values with the help of accumulator. So generated intermediate outputs are later merged to produce the final output represented by a single value.
- grouping state - same as combine except it doesn't use accumulators and thus groups the values at once.
State examples
The points described above are presented in the following tests:
private static final Instant NOW = new Instant(0); private static final Instant SEC_1_DURATION = NOW.plus(Duration.standardSeconds(1)); private static final Instant SEC_2_DURATION = NOW.plus(Duration.standardSeconds(2)); private static final Instant SEC_5_DURATION = NOW.plus(Duration.standardSeconds(5)); private static final Instant SEC_6_DURATION = NOW.plus(Duration.standardSeconds(6)); @Test public void should_fail_on_applying_stateful_transform_for_not_key_value_pairs() { Pipeline pipeline = BeamFunctions.createPipeline("Shared state example"); Coder<String> utfCoder = StringUtf8Coder.of(); TestStream<String> words = TestStream.create(utfCoder).addElements( TimestampedValue.of("cat", SEC_1_DURATION), TimestampedValue.of("hat", SEC_1_DURATION) ) .advanceWatermarkToInfinity(); Duration windowDuration = Duration.standardSeconds(5); Window<String> window = Window.<String>into(FixedWindows.of(windowDuration)); assertThatThrownBy(() -> { pipeline.apply(words).apply(window).apply(ParDo.of(new MinLengthTextSharedStateProcessing(2))); pipeline.run().waitUntilFinish(); }).isInstanceOf(IllegalArgumentException.class) .hasMessage("ParDo requires its input to use KvCoder in order to use state and timers."); } @Test public void should_correctly_increment_counter_for_each_encountered_item() { Pipeline pipeline = BeamFunctions.createPipeline("Counter example"); Coder<String> utf8Coder = StringUtf8Coder.of(); Coder<Integer> varIntCoder = VarIntCoder.of(); KvCoder<String, Integer> keyValueCoder = KvCoder.of(utf8Coder, varIntCoder); TestStream<KV<String, Integer>> words = TestStream.create(keyValueCoder).addElements( TimestampedValue.of(KV.of("a", 1), SEC_1_DURATION), TimestampedValue.of(KV.of("a", 2), SEC_1_DURATION), TimestampedValue.of(KV.of("b", 5), SEC_1_DURATION), TimestampedValue.of(KV.of("a", 6), SEC_1_DURATION), TimestampedValue.of(KV.of("c", 2), SEC_1_DURATION), TimestampedValue.of(KV.of("a", 7), SEC_1_DURATION), TimestampedValue.of(KV.of("a", 3), SEC_1_DURATION), TimestampedValue.of(KV.of("a", 9), SEC_1_DURATION), TimestampedValue.of(KV.of("d", 2), SEC_1_DURATION), TimestampedValue.of(KV.of("a", 1), SEC_1_DURATION), TimestampedValue.of(KV.of("a", 2), SEC_1_DURATION) ) .advanceWatermarkToInfinity(); Duration windowDuration = Duration.standardSeconds(15); Window<KV<String, Integer>> window = Window.<KV<String, Integer>>into(FixedWindows.of(windowDuration)) .triggering(AfterPane.elementCountAtLeast(20)) .withAllowedLateness(windowDuration, Window.ClosingBehavior.FIRE_ALWAYS) .accumulatingFiredPanes(); PCollection<String> results = pipeline.apply(words).apply(window) .apply(ParDo.of(new DoFn<KV<String, Integer>, String>() { private static final String COUNTER_NAME = "counter"; // Its definition is required, otherwise the following exception is thrown: // parameter of type MapState<String, Integer> at index 1: reference to undeclared StateId: "counter" @StateId(COUNTER_NAME) private final StateSpec<MapState<String, Integer>> mapState = StateSpecs.map(); @ProcessElement public void process(ProcessContext processContext, @StateId(COUNTER_NAME) MapState<String, Integer> letterCounterState) { KV<String, Integer> element = processContext.element(); ReadableState<Integer> letterSumState = letterCounterState.get(element.getKey()); int currentSum = letterSumState.read() != null ? letterSumState.read() : 0; int letterSum = currentSum + element.getValue(); letterCounterState.put(element.getKey(), letterSum); processContext.output(element.getKey()+"="+letterSum); } })); IntervalWindow window1 = new IntervalWindow(NOW, NOW.plus(windowDuration)); // It also shows that state is not adapted to be returned every time because instead of having a, b,c and d // with final values, we have intermediary results PAssert.that(results).inFinalPane(window1).containsInAnyOrder("a=31", "a=29", "a=28", "a=19", "a=16", "a=9", "a=3", "b=5", "a=1", "c=2", "d=2"); pipeline.run().waitUntilFinish(); } @Test public void should_show_the_use_of_combine_state() { Pipeline pipeline = BeamFunctions.createPipeline("Combined state example"); Coder<String> utf8Coder = StringUtf8Coder.of(); KvCoder<String, String> keyValueCoder = KvCoder.of(utf8Coder, utf8Coder); TestStream<KV<String, String>> words = TestStream.create(keyValueCoder).addElements( TimestampedValue.of(KV.of("10:00", "/index.html"), SEC_1_DURATION), TimestampedValue.of(KV.of("10:01", "/cart.html"), SEC_2_DURATION), TimestampedValue.of(KV.of("10:01", "/cancel_order.html"), SEC_1_DURATION), TimestampedValue.of(KV.of("10:05", "/delivery.html"), SEC_5_DURATION), TimestampedValue.of(KV.of("10:02", "/index.html"), SEC_2_DURATION), TimestampedValue.of(KV.of("10:06", "/cart.html"), SEC_6_DURATION), TimestampedValue.of(KV.of("10:01", "/login.html"), SEC_6_DURATION), TimestampedValue.of(KV.of("10:07", "/payment.html"), SEC_6_DURATION.plus(Duration.standardSeconds(1))), TimestampedValue.of(KV.of("10:08", "/order_confirmation.html"), SEC_6_DURATION.plus(Duration.standardSeconds(2))) ) .advanceWatermarkToInfinity(); Duration windowDuration = Duration.standardSeconds(5); Window<KV<String, String>> window = Window.<KV<String, String>>into(FixedWindows.of(windowDuration)) .triggering(AfterPane.elementCountAtLeast(20)) .withAllowedLateness(windowDuration, Window.ClosingBehavior.FIRE_ALWAYS) .accumulatingFiredPanes(); PCollection<KV<String, Integer>> results = pipeline.apply(words).apply(window).apply(ParDo.of(new SessionTransform())); IntervalWindow window1 = new IntervalWindow(NOW, NOW.plus(windowDuration)); // This assert shows that the stateful processing doesn't work well when we output the computed values every time // The output for 10:01 is returned twice PAssert.that(results).inFinalPane(window1).containsInAnyOrder(KV.of("10:00", 1), KV.of("10:01", 2), KV.of("10:02", 1), KV.of("10:01", 1)); // The presence of 10:01 with different value than in previous window proves that the state is bounded // to the window duration IntervalWindow window2 = new IntervalWindow(NOW.plus(windowDuration), NOW.plus(windowDuration).plus(windowDuration)); PAssert.that(results).inFinalPane(window2).containsInAnyOrder(KV.of("10:05", 1), KV.of("10:06", 1), KV.of("10:07", 1), KV.of("10:08", 1), KV.of("10:01", 1)); pipeline.run().waitUntilFinish(); } @Test public void should_accumulate_encountered_words_with_bagstate() { Pipeline pipeline = BeamFunctions.createPipeline("Bag state example"); Coder<String> utf8Coder = StringUtf8Coder.of(); KvCoder<String, String> keyValueCoder = KvCoder.of(utf8Coder, utf8Coder); TestStream<KV<String, String>> words = TestStream.create(keyValueCoder).addElements( TimestampedValue.of(KV.of("p", "paradigm"), SEC_1_DURATION), TimestampedValue.of(KV.of("p", "programming"), SEC_1_DURATION) ) .advanceWatermarkToInfinity(); Duration windowDuration = Duration.standardSeconds(15); Window<KV<String, String>> window = Window.<KV<String, String>>into(FixedWindows.of(windowDuration)) .triggering(AfterPane.elementCountAtLeast(20)) .withAllowedLateness(windowDuration, Window.ClosingBehavior.FIRE_ALWAYS) .accumulatingFiredPanes(); PCollection<String> results = pipeline.apply(words).apply(window) .apply(ParDo.of(new DoFn<KV<String, String>, String>() { private static final String ACCUMULATOR_NAME = "accumulator"; @StateId(ACCUMULATOR_NAME) private final StateSpec<BagState<String>> accumulatorStateSpec = StateSpecs.bag(); @ProcessElement public void processElement(ProcessContext processContext, @StateId(ACCUMULATOR_NAME) BagState<String> wordsAccumulator) { KV<String, String> letterWordPair = processContext.element(); wordsAccumulator.add(letterWordPair.getValue()); processContext.output(Joiner.on(",").join(wordsAccumulator.read())); } })); IntervalWindow window1 = new IntervalWindow(NOW, NOW.plus(windowDuration)); // Since the ordering is not deterministic, we check against 2 possible outputs PAssert.that(results).inFinalPane(window1).satisfies((SerializableFunction<Iterable<String>, Void>) input -> { List<String> expectedWords = Arrays.asList("paradigm", "paradigm,programming", "programming", "programming,paradigm"); int matchedWords = 0; for (String generatedWord : input) { if (expectedWords.contains(generatedWord)) { matchedWords++; } } Assert.assertEquals(matchedWords, 2); return null; }); pipeline.run().waitUntilFinish(); } @Test public void should_show_that_stateful_processing_order_is_also_not_deterministic() { List<KV<String, String>> clubsPerCountry = Arrays.asList( KV.of("Germany", "VfB Stuttgart"), KV.of("Germany", "Bayern Munich"), KV.of("Germany", "FC Koln")); for (int i = 0; i < 10; i++) { Pipeline pipeline = BeamFunctions.createPipeline("Not deterministic stateful processing"); pipeline.apply(Create.of(clubsPerCountry)) .apply(ParDo.of(new DoFn<KV<String, String>, String>() { private static final String ACCUMULATOR_NAME = "accumulator"; @StateId(ACCUMULATOR_NAME) private final StateSpec<BagState<String>> accumulatorStateSpec = StateSpecs.bag(); @ProcessElement public void processElement(ProcessContext processContext, @StateId(ACCUMULATOR_NAME) BagState<String> clubsAccumulator) { clubsAccumulator.add(processContext.element().getValue()); String clubs = Joiner.on("-").join(clubsAccumulator.read()); StringsAccumulator.CLUBS.add(clubs); processContext.output(clubs); } })); pipeline.run().waitUntilFinish(); } // We deal with 3 keys. If the processing order would be guaranteed, we'd always retrieve the same pairs. // It means we'd have the size of accumulated entries equal to 3. But since the processing order is not // deterministic, obviously we have more items than that. assertThat(StringsAccumulator.CLUBS.getEntries().size()).isGreaterThan(3); } @Test public void should_show_that_stateful_processing_is_key_based() { List<KV<String, String>> clubsPerCountry = Arrays.asList( KV.of("Germany", "VfB Stuttgart"), KV.of("Germany", "Bayern Munich"), KV.of("Germany", "FC Koln"), KV.of("Holland", "Ajax Amsterdam"), KV.of("Holland", "Sparta Rotterdam"), KV.of("Spain", "FC Barcelona"), KV.of("Spain", "Real Madrid")); Pipeline pipeline = BeamFunctions.createPipeline("Not deterministic stateful processing"); PCollection<String> results = pipeline.apply(Create.of(clubsPerCountry)) .apply(ParDo.of(new DoFn<KV<String, String>, String>() { private static final String COUNTER_NAME = "occurrences_counter"; @StateId(COUNTER_NAME) private final StateSpec<ValueState<Integer>> counter = StateSpecs.value(VarIntCoder.of()); @ProcessElement public void processElement(ProcessContext processContext, @StateId(COUNTER_NAME) ValueState<Integer> counterState) { int currentValue = Optional.ofNullable(counterState.read()).orElse(0); int incrementedCounter = currentValue + 1; counterState.write(incrementedCounter); processContext.output(processContext.element().getKey()+"="+incrementedCounter); } })); // If the state cell wasn't be key-based, the number of accumulated entries would probably be incremental, // i.e. 1, 2, 3, 4, 5, 6, 7 PAssert.that(results).containsInAnyOrder("Germany=1", "Germany=2", "Germany=3", "Holland=1", "Holland=2", "Spain=1", "Spain=2"); pipeline.run().waitUntilFinish(); } @Test public void should_fail_when_two_states_with_the_same_ids_are_defined() { Pipeline pipeline = BeamFunctions.createPipeline("Two states with the same name"); List<KV<String, String>> clubsPerCountry = Arrays.asList( KV.of("Germany", "VfB Stuttgart"), KV.of("Germany", "Bayern Munich"), KV.of("Germany", "FC Koln")); assertThatThrownBy(() -> { pipeline.apply(Create.of(clubsPerCountry)) .apply(ParDo.of(new DoFn<KV<String, String>, String>() { private static final String ACCUMULATOR_NAME = "accumulator"; @StateId(ACCUMULATOR_NAME) private final StateSpec<BagState<String>> accumulatorStateSpec = StateSpecs.bag(); @StateId(ACCUMULATOR_NAME) private final StateSpec<BagState<String>> accumulatorStateSpecDuplicated = StateSpecs.bag(); @ProcessElement public void processElement(ProcessContext processContext, @StateId(ACCUMULATOR_NAME) BagState<String> clubsAccumulator) { clubsAccumulator.add(processContext.element().getValue()); String clubs = Joiner.on(", ").join(clubsAccumulator.read()); processContext.output(clubs); } })); pipeline.run().waitUntilFinish(); }).isInstanceOf(IllegalArgumentException.class) .hasMessageContaining("Duplicate StateId \"accumulator\", used on both of " + "[private final org.apache.beam.sdk.state.StateSpec ") .hasMessageContaining("and [private final org.apache.beam.sdk.state.StateSpec"); } @Test public void should_fail_when_the_state_spec_declaration_is_not_final() { Pipeline pipeline = BeamFunctions.createPipeline("Two states with the same name"); List<KV<String, String>> clubsPerCountry = Arrays.asList( KV.of("Germany", "VfB Stuttgart"), KV.of("Germany", "Bayern Munich"), KV.of("Germany", "FC Koln")); assertThatThrownBy(() -> { pipeline.apply(Create.of(clubsPerCountry)) .apply(ParDo.of(new DoFn<KV<String, String>, String>() { private static final String ACCUMULATOR_NAME = "accumulator"; @StateId(ACCUMULATOR_NAME) private StateSpec<BagState<String>> accumulatorStateSpec = StateSpecs.bag(); @ProcessElement public void processElement(ProcessContext processContext, @StateId(ACCUMULATOR_NAME) BagState<String> clubsAccumulator) { clubsAccumulator.add(processContext.element().getValue()); String clubs = Joiner.on(", ").join(clubsAccumulator.read()); processContext.output(clubs); } })); pipeline.run().waitUntilFinish(); }).isInstanceOf(IllegalArgumentException.class) .hasMessageContaining("Non-final field private").hasMessageContaining("org.apache.beam.sdk.state.StateSpec") .hasMessageContaining("annotated with StateId. State declarations must be final."); } enum StringsAccumulator { CLUBS; private Set<String> entries = new HashSet<>(); public void add(String entry) { System.out.println("Adding " + entry); entries.add(entry); } public Set<String> getEntries() { return entries; } } public static class MinLengthTextSharedStateProcessing extends DoFn<String, String> { private static final String COUNTER_NAME = "counter"; @StateId(COUNTER_NAME) private final StateSpec<ValueState<Integer>> counter = StateSpecs.value(VarIntCoder.of()); private int textMinLength; public MinLengthTextSharedStateProcessing(int textMinLength) { this.textMinLength = textMinLength; } @ProcessElement public void process(ProcessContext processContext, @StateId(COUNTER_NAME) ValueState<Integer> counterState) { String word = processContext.element(); if (word.length() >= textMinLength) { int counterValue = counterState.read(); int newCounterValue = counterValue + 1; counterState.write(newCounterValue); processContext.output(word+"="+newCounterValue); } } } public static class SessionCombiner extends Combine.CombineFn<String, List<String>, Integer> { @Override public List<String> createAccumulator() { return new ArrayList<>(); } @Override public List<String> addInput(List<String> accumulator, String log) { accumulator.add(log); return accumulator; } @Override public List<String> mergeAccumulators(Iterable<List<String>> accumulators) { List<String> mergedAccumulator = new ArrayList<>(); accumulators.forEach(accumulatorToMerge -> mergedAccumulator.addAll(accumulatorToMerge)); return mergedAccumulator; } @Override public Integer extractOutput(List<String> accumulator) { return accumulator.size(); } } public static class SessionTransform extends DoFn<KV<String, String>, KV<String, Integer>> { private static final String COUNTER_NAME = "counter"; @StateId(COUNTER_NAME) private final StateSpec<CombiningState<String, List<String>, Integer>> sessionStateSpec = StateSpecs.combining(ListCoder.of(StringUtf8Coder.of()), new SessionCombiner()); @ProcessElement public void process(ProcessContext processContext, @StateId(COUNTER_NAME) CombiningState<String, List<String>, Integer> sessionState) { KV<String, String> element = processContext.element(); sessionState.add(element.getValue()); processContext.output(KV.of(element.getKey(), sessionState.read())); } }
The stateful processing is available in Apache Beam as it's available in the most of data processing frameworks (e.g. Spark). The state represents a situation happened between 2 different points in time. Moreover, it applies only to keyed datasets and it ends when the window expires. Its use is pretty straightforward since it's based on annotating the state object and defining it later in the processing method. As shown in the 2nd and the 3rd sections, the state can be of different type and a given key can have multiple state objects that can be of different types.
Consulting
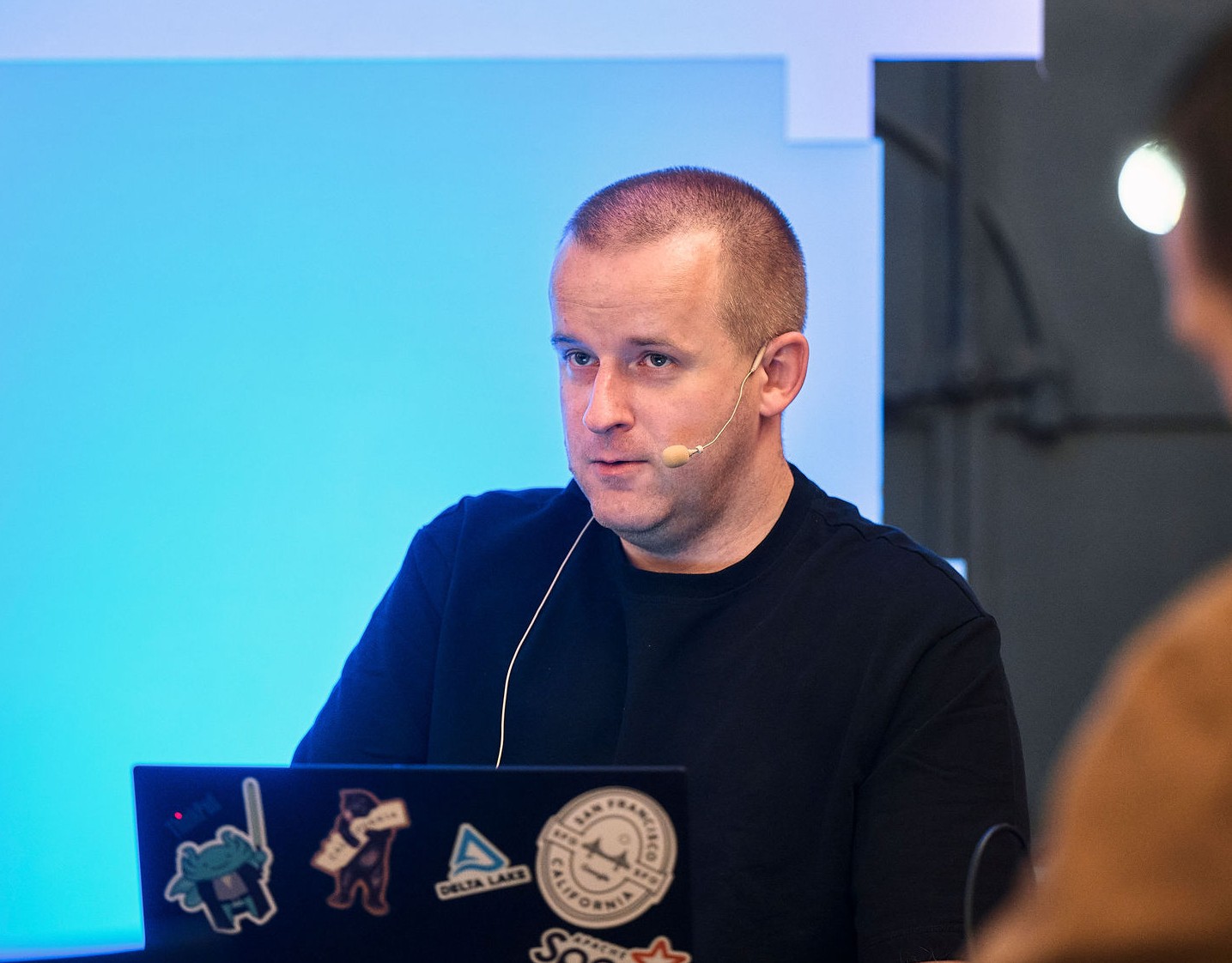
With nearly 16 years of experience, including 8 as data engineer, I offer expert consulting to design and optimize scalable data solutions.
As an O’Reilly author, Data+AI Summit speaker, and blogger, I bring cutting-edge insights to modernize infrastructure, build robust pipelines, and
drive data-driven decision-making. Let's transform your data challenges into opportunities—reach out to elevate your data engineering game today!
👉 contact@waitingforcode.com
đź”— past projects