An efficient data storage is one of success keys of a good storage format. One of methods helping to improve that is an appropriate encoding and Parquet comes with several different methods.
Data Engineering Design Patterns
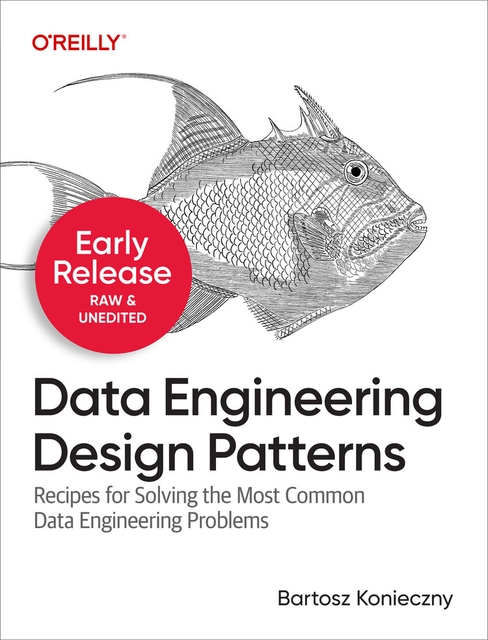
Looking for a book that defines and solves most common data engineering problems? I'm currently writing
one on that topic and the first chapters are already available in π
Early Release on the O'Reilly platform
I also help solve your data engineering problems π contact@waitingforcode.com π©
This post starts with a short reminder about encoding. The second part lists the encodings available in the version 2 of Parquet format. Only the encodings that are not marked as deprecated will be explained here. The last part defines several learning tests showing how an adapted encoding method can reduce the storage needs.
Encoding definition
The encoding consists on transforming given data to its coded form. Depending on used method, the encoding can have different purposes: ensuring the data correctness (e.g. checksums), shortening the data (e.g. base64) or writing characters correctly (e.g. UTF-8 encoding).
A very illustrative example of encoding is the base64 algorithm that converts a text into ASCII representation. If we take the method given in MDN and we try to encode the text "waitingforcode", we'll receive its encoded version as d2FpdGluZ2ZvcmNvZGU=.
Encodings in Parquet
Since Apache Parquet is supposed to deal with a lot of data, the encodings are used mostly to store the data more efficiently. Among the list of available and not deprecated encodings we can distinguish:
- plain - it's available for all types supported by Parquet. It encodes the values back to back and is used as a last resort when there is no more efficient encoding for given data. The plain encoding always reserves the same amount of place for given type. For instance, an int 32 bits will be always stored in 4 bytes. The image below shows how the numbers from 0 to 3 will be stored with plain encoding:
- RLE/bit-packing hybrid - this method deals with one of plain encoding drawbacks - space wasting. Let's imagine that out column stores the age of our users. If we use plain encoding, each value will be stored in 4 bytes. But if we know that the oldest user is 99 years old and that we can store this column in 7 bits in maximum (99 in binary number is represented as 1100011). RLE/bit-packing hybrid approach helps to fix this issue.
The "hybrid" word in the name is not a mistake. The method is a combination of RLE and bit-packing algorithms. Depending on the values character (repeatable or not a lot) it chooses one of these 2 methods to encode given range of values. It means that one column can have different encodings ! To understand better what the hybrid approach can do, let's first explain globally the ideas of RLE and bit-packing encodings.
RLE is an acronym from Run-Length Encoding. It fits pretty well for repeated data. Rather than encoding values back to back, it detects how many time given value appears consecutively (this appearance is called data run). Later, it encodes given value as: the number of repetitions and the value. It's very efficient when the encoded data contains a lot of data runs, as in the picture below:
Bit-packing is based on the assumption that every int32 or int64 value doesn't always need all 32 or 64 bits. Thus instead of storing these values in their full ranges, the bit-packing packs multiple values into a single space. The values are packed from the least significant bit of each byte to the most significant bit. The least significant bit is the lowest bit in the series of numbers represented in binary format. It's located at the far right of the representation. The formula used to pack the values uses bitwise operations. For example, to pack the numbers from 0 to 7 with the bitwidth of 3, it uses the following bitwise operations:
# bitwidth of 3 output[0] = ((input[0] & 7) | ((input[1] & 7) << 3) | ((input[2] & 7) << 6)) & 255 output[1] = (((input[2] & 7) >>> 2) | ((input[3] & 7) << 1) | ((input[4] & 7) << 4)) | ((input[5] & 7) << 7)) & 255 output[2] = (((input[5] & 7) >>> 1) | ((input[6] & 7) << 2) | ((input[7] & 7) << 5)) & 255
The image below shows the generation of the first element of the array:
- delta - this encoding can be used either for integers (int32 and int64) or for array of bytes. This method is quite easy to understand on the example of integers. The following snippet shows how the decimal values can be encoded:
to_encode = [1, 2, 5, 7, 9] encoded = [1, 1, 3, 2, 2]
As you simply deduce, the delta encoding encodes the first value then the difference between subsequent entries. But in Parquet the format is a little bit different. It first stores the header that contains the block size, miniblock count (each block with encoded values is composed of multiple mini blocks), the number of values and finally the first value. Later each miniblock is composed of the minimal delta and the bitwidth of encoded bytes.
The delta encoding can reveal very efficient for datetime columns, stored for instance in milliseconds where only the difference between subsequent events will be saved instead of each value occupied every time 64 bits. Globally it's advised for the cases where the variation is much smaller than the absolute values.
That was for integers but what with binary and especially text values ? The delta encoding used here is also called incremental encoding and is very efficient for the ordered strings with common prefix or suffix, for instance in dictionaries. Let's take an precise example:
to_encode = [abc, abcd, abcde, abcdef] encoded = [abc, 3d, 4e, 5e, 6f]
As shown, the incremental encoding stores the first value and then saves only the number corresponding to the common prefix concatenated with different characters.
Parquet encoding examples
Through the following learning tests we'll see how Parquet encodings behave against the real data:
@Test public void should_store_repeatable_data_more_efficiently_than_in_plain_storage() throws IOException { DirectByteBufferAllocator bytesAllocator = DirectByteBufferAllocator.getInstance(); int slabSize = 256; int pageSize = 1000; DeltaByteArrayWriter deltaByteArrayWriter = new DeltaByteArrayWriter(slabSize, pageSize, bytesAllocator); List<String> words = Lists.newArrayList("absorb", "absorption", "acceleration", "action", "ampere", "amplitude", "cadency", "cadent", "cadential", "cadet", "collision", "color", "colorfast", "colorful", "racketeering", "racketing", "rackets", "rackety"); List<Binary> binaryWords = words.stream().map(word -> fromString(word)).collect(Collectors.toList()); binaryWords.forEach(binaryWord -> deltaByteArrayWriter.writeBytes(binaryWord)); String allWords = words.stream().reduce(String::concat).get(); byte[] encodedBytes = deltaByteArrayWriter.getBytes().toByteArray(); byte[] allWordsBytes = allWords.getBytes("UTF-8"); // As you can see, the DeltaByteArray is more efficient in the case when the letters are dictionary-like // However, you can go to the test should_store_non_repeatable_data_less_efficiently_than_in_plain_storage // to see what happens if the data is not dictionary-like assertThat(encodedBytes).hasSize(138); assertThat(allWordsBytes).hasSize(142); } @Test public void should_store_non_repeatable_data_less_efficiently_than_in_plain_storage() throws IOException { DirectByteBufferAllocator bytesAllocator = DirectByteBufferAllocator.getInstance(); int slabSize = 256; int pageSize = 1000; DeltaByteArrayWriter deltaByteArrayWriter = new DeltaByteArrayWriter(slabSize, pageSize, bytesAllocator); List<String> words = Lists.newArrayList("absorb", "acceleration", "ampere", "cadency", "collision", "racketeering", "sad", "sale", "sanction"); List<Binary> binaryWords = words.stream().map(word -> fromString(word)).collect(Collectors.toList()); binaryWords.forEach(binaryWord -> deltaByteArrayWriter.writeBytes(binaryWord)); String allWords = words.stream().reduce(String::concat).get(); byte[] encodedBytes = deltaByteArrayWriter.getBytes().toByteArray(); byte[] allWordsBytes = allWords.getBytes("UTF-8"); // Here the data is less dictionary-like, so the encoding should be worse assertThat(encodedBytes).hasSize(104); assertThat(allWordsBytes).hasSize(67); } @Test public void should_store_ints_with_rle_hybrid_encoder_more_efficiently_than_in_plain_storage() throws IOException { DirectByteBufferAllocator bytesAllocator = DirectByteBufferAllocator.getInstance(); int initialCapacity = 256; int pageSize = 1000; int bitWidth = 4; RunLengthBitPackingHybridEncoder runLengthBitPackingHybridEncoder = new RunLengthBitPackingHybridEncoder(bitWidth, initialCapacity, pageSize, bytesAllocator); List<Integer> numbers = IntStream.range(0, 100).boxed().collect(Collectors.toList()); List<Byte> plainNumberBytes = new ArrayList<>(); for (int i = 0; i < numbers.size(); i++) { runLengthBitPackingHybridEncoder.writeInt(numbers.get(i)); plainNumberBytes.add(numbers.get(i).byteValue()); } byte[] encodedBytes = runLengthBitPackingHybridEncoder.toBytes().toByteArray(); assertThat(encodedBytes).hasSize(53); assertThat(plainNumberBytes).hasSize(100); } @Test public void should_apply_either_rle_or_bit_packing_for_values_of_different_characteristics() throws IOException { DirectByteBufferAllocator bytesAllocator = DirectByteBufferAllocator.getInstance(); int initialCapacity = 256; int pageSize = 1000; int bitWidth = 4; RunLengthBitPackingHybridEncoder runLengthBitPackingHybridEncoder = new RunLengthBitPackingHybridEncoder(bitWidth, initialCapacity, pageSize, bytesAllocator); // RLE is written after 8 repeated values int repeatedValue = 3; for(int iteration = 0; iteration < 9; iteration++) { runLengthBitPackingHybridEncoder.writeInt(repeatedValue); } // Otherwise the values are bit-packed int bitPackedValue1 = 0; int bitPackedValue2 = 1; for (int iteration = 0; iteration < 4; iteration++) { runLengthBitPackingHybridEncoder.writeInt(bitPackedValue1); runLengthBitPackingHybridEncoder.writeInt(bitPackedValue2); } byte[] encodedBytes = runLengthBitPackingHybridEncoder.toBytes().toByteArray(); String encodedBytesRepresentation = stringifyBytes(encodedBytes); // In received results: // * 00010010 - 1 bit left shifted number of repetitions (18, after >> 1 it gives 9 repetitions) // * 00000101 - repeated value (3 in that case) // * the next values represent bit packed 0,1,0,1,0,1,0,1 pairs assertThat(encodedBytes).hasSize(7); assertThat(encodedBytesRepresentation).isEqualTo("00010010 00000011 00000011 00010000 00010000 00010000 00010000"); } @Test public void should_store_small_variations_with_delta_encoding_more_efficiently_than_in_plain_storage() throws IOException { DirectByteBufferAllocator bytesAllocator = DirectByteBufferAllocator.getInstance(); int slabSize = 256; int pageSize = 1000; DeltaBinaryPackingValuesWriterForInteger deltaBinaryPackingValuesWriterForInteger = new DeltaBinaryPackingValuesWriterForInteger(slabSize, pageSize, bytesAllocator); List<Integer> numbers = IntStream.range(0, 100).boxed().collect(Collectors.toList()); List<Byte> plainNumberBytes = new ArrayList<>(); for (int i = 0; i < numbers.size(); i++) { deltaBinaryPackingValuesWriterForInteger.writeInteger(numbers.get(i)); plainNumberBytes.add(numbers.get(i).byteValue()); } byte[] encodedBytes = deltaBinaryPackingValuesWriterForInteger.getBytes().toByteArray(); assertThat(encodedBytes).hasSize(10); // 10 times less space is needed for delta encoding with auto-incremented values // Even if the variation is bigger, the storage is still efficient assertThat(plainNumberBytes).hasSize(100); } @Test public void should_store_big_variations_with_delta_encoding_more_efficiently_than_in_plain_storage() throws IOException { DirectByteBufferAllocator bytesAllocator = DirectByteBufferAllocator.getInstance(); int slabSize = 256; int pageSize = 1000; DeltaBinaryPackingValuesWriterForInteger deltaBinaryPackingValuesWriterForInteger = new DeltaBinaryPackingValuesWriterForInteger(slabSize, pageSize, bytesAllocator); List<Integer> numbers = IntStream.range(0, 100).boxed().collect(Collectors.toList()); List<Byte> plainNumberBytes = new ArrayList<>(); for (int i = 0; i < numbers.size(); i++) { Integer multipleOf3000 = numbers.get(i)*3000; deltaBinaryPackingValuesWriterForInteger.writeInteger(multipleOf3000); plainNumberBytes.add(multipleOf3000.byteValue()); } byte[] encodedBytes = deltaBinaryPackingValuesWriterForInteger.getBytes().toByteArray(); assertThat(encodedBytes).hasSize(11); assertThat(plainNumberBytes).hasSize(100); } @Test public void should_store_ints_with_deprecated_bit_packing() throws IOException { ByteArrayOutputStream byteArrayOutputStream = new ByteArrayOutputStream(); int intLengthInBits = 3; BitPacking.BitPackingWriter bitPackingWriter = BitPacking.getBitPackingWriter(intLengthInBits, byteArrayOutputStream); List<Integer> numbers = Arrays.asList(0, 1, 2, 3, 4, 5, 6, 7); for (int i = 0; i < numbers.size(); i++) { bitPackingWriter.write(numbers.get(i)); } bitPackingWriter.finish(); byte[] encodedBytes = byteArrayOutputStream.toByteArray(); // 8 int32 number, represented as 3 bits are finally written with only 3 bytes // instead of 8 bytes if we'd use the plain encoding assertThat(encodedBytes).hasSize(3); String encodedBytesRepresentation = stringifyBytes(encodedBytes); // This bit packing corresponds to the deprecated version - the values are packed from the most significant bit // to the least significant bit; So in our case they're packed back to back assertThat(encodedBytesRepresentation).isEqualTo("00000101 00111001 01110111"); } @Test public void should_not_change_int_storage_with_deprecated_8_bitwidth() throws IOException { ByteArrayOutputStream byteArrayOutputStream = new ByteArrayOutputStream(); int intLengthInBits = 8; BitPacking.BitPackingWriter bitPackingWriter = BitPacking.getBitPackingWriter(intLengthInBits, byteArrayOutputStream); List<Integer> numbers = Arrays.asList(0, 1, 2, 3, 4, 5, 6, 7); for (int i = 0; i < numbers.size(); i++) { bitPackingWriter.write(numbers.get(i)); } bitPackingWriter.finish(); byte[] encodedBytes = byteArrayOutputStream.toByteArray(); // Here the ints are represented as in plain encoding, so it doesn't bring a lot of advantages here assertThat(encodedBytes).hasSize(8); } @Test public void should_store_small_number_of_ints_with_rle_bit_packing_approach() throws IOException { int bitWidth = 3; BytePacker bytePacker = ByteBitPackingLE.factory.newBytePacker(bitWidth); byte[] outputValues = new byte[bitWidth]; int[] inputValues = new int[] {0, 1, 2, 3, 4, 5, 6, 7}; int startPosition = 0; int outputPosition = 0; bytePacker.pack8Values(inputValues, startPosition, outputValues, outputPosition); String encodedBytesRepresentation = stringifyBytes(outputValues); // If you compare the result with should_store_ints_with_deprecated_bit_packing // you will see that the bits are not encoded in the same logic assertThat(encodedBytesRepresentation).isEqualTo("10001000 11000110 11111010"); } @Test public void should_store_plain_encoded_values_with_defined_rule_of_32_bytes() throws IOException { DirectByteBufferAllocator bytesAllocator = DirectByteBufferAllocator.getInstance(); int initialSize = 128; int pageSize = 1000; PlainValuesWriter plainValuesWriter = new PlainValuesWriter(initialSize, pageSize, bytesAllocator); List<Integer> numbers = IntStream.rangeClosed(1, 10).boxed().collect(Collectors.toList()); for (int i = 0; i < numbers.size(); i++) { plainValuesWriter.writeInteger(numbers.get(i)); } byte[] encodedBytes = plainValuesWriter.getBytes().toByteArray(); String encodedBytesRepresentation = stringifyBytes(encodedBytes); System.out.println("Encoded ="+encodedBytesRepresentation); // 40 bytes should be used because we're writing int32 that takes // 4 bytes for every value assertThat(encodedBytes).hasSize(40); } @Test public void should_store_plain_encoded_texts_as_length_variable_arrays() throws IOException { DirectByteBufferAllocator bytesAllocator = DirectByteBufferAllocator.getInstance(); int initialSize = 128; int pageSize = 1000; PlainValuesWriter plainValuesWriter = new PlainValuesWriter(initialSize, pageSize, bytesAllocator); plainValuesWriter.writeBytes(fromString("Amsterdam")); plainValuesWriter.writeBytes(fromString("Basel")); plainValuesWriter.writeBytes(fromString("Chicago")); plainValuesWriter.writeBytes(fromString("Dortmund")); byte[] encodedBytes = plainValuesWriter.getBytes().toByteArray(); // for binary data, the following information is written: // * the length in 4 bytes // * the value // Thus it gives: // Amsterdam = 4 + 9 = 13 // Basel = 4 + 5 = 9 // Chicago = 4 + 7 = 11 // Dortmund = 4 + 8 = 12 // = 45 assertThat(encodedBytes).hasSize(45); } private static String stringifyBytes(byte[] bytes) { StringBuilder stringBuilder = new StringBuilder(); for(int i = 0; i < bytes.length; i++) { int oneByte = bytes[i]; if (oneByte < 0) { oneByte = 256 + oneByte; } stringBuilder.append(stringifyNumber(oneByte)).append(" "); } return stringBuilder.toString().trim(); } private static String stringifyNumber(int number) { StringBuilder stringBuilder = new StringBuilder(); String numberBinaryRepresentation = Integer.toBinaryString(number); int representationLength = numberBinaryRepresentation.length(); while (representationLength < 8) { stringBuilder.append("0"); representationLength++; } stringBuilder.append(numberBinaryRepresentation); return stringBuilder.toString(); }
Apache Parquet shows how an appropriated encoding can make data storage more efficient. The framework implements different algorithms that are chosen depending of the column type (delta) or values characteristics (RLE/Bit-packing hybrid). For the cases when there is no special encoding that could be applied, Parquet stores the values in plain encoding that for each entry takes a predefined amount of space.