Without any explicit definition, Spark SQL won't partition any data, i.e. all rows will be processed by one executor. It's not optimal since Spark was designed to parallel and distributed processing.
Data Engineering Design Patterns
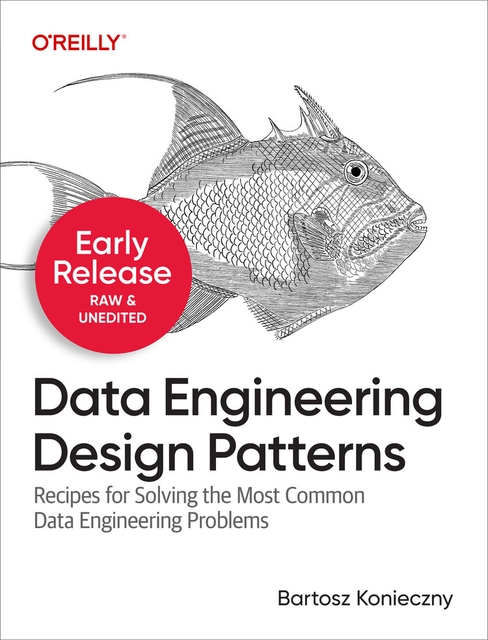
Looking for a book that defines and solves most common data engineering problems? I'm currently writing
one on that topic and the first chapters are already available in π
Early Release on the O'Reilly platform
I also help solve your data engineering problems π contact@waitingforcode.com π©
This post focuses on partitioning in Spark SQL. The first part explains how to configure it during the construction of JDBC DataFrame. The second part, through some learning tests, will show how the partitioning works.
Configure partitioning in Spark SQL
Correctly balanced partitions help to improve application performance. Ideally, each of executors would work on similar subset of data. To configure that in Spark SQL using RDBMS connections we must define 4 options during DataFrameReader building: the partition column, the upper and lower bounds and the desired number of partitions. At first glance it seems to be not complicated but after some code writing, they all deserve some explanations:
- partitionColumn - as the name indicates, it defines the name of the column which data will be used to partition rows. One important preqrequisite - the row must be of numeric (integer or decimal) type.
- numberOfPartitions - no surprises here, it defines the desired number of partitions. The "desired" is the important word to keep in mind. To see why, let's analyze the following code generating the object called PartitionInfo:
val numPartitions = if ((upperBound - lowerBound) >= partitioning.numPartitions) { partitioning.numPartitions } else { logWarning("The number of partitions is reduced because the specified number of " + "partitions is less than the difference between upper bound and lower bound. " + s"Updated number of partitions: ${upperBound - lowerBound}; Input number of " + s"partitions: ${partitioning.numPartitions}; Lower bound: $lowerBound; " + s"Upper bound: $upperBound.") upperBound - lowerBound }
As you see, the number of partitions, under some circumstances, can be lower than defined. - lower and upper bounds - as you saw above, these 2 parameters have at least an influence on partitions number. But it's not their single purpose. In additional, or maybe among others, they're used to define the slices containing partitioning data.
These boundaries generate the stride. It specifies how many rows of a given range of partition column values can be kept within a single partition. To understand it better, let's take a look at the simplified algorithm coming from org.apache.spark.sql.execution.datasources.jdbc.JDBCRelation#columnPartition(partitioning: JDBCPartitioningInfo) method:stride = (upper_bound/partitions_number) - (lower_bound/partitions_number) partition_nr = 0 while (partition_nr < partitions_number) generate WHERE clause: partition_column IS NULL OR partition_column < stride if: partition_nr == 0 AND partition_nr < partitions_number or generate WHERE clause: partition_column >= stride AND partition_column < next_stride if: partition_nr > 0 AND partition_nr < partitions_number or generate WHERE clause partition_column >= stride if: partition_nr > 0 AND partition_nr == partitions_number where next_stride is calculated after computing the left sideo of the WHERE clause by next_stride += stride
Thus, if we define the number of partitions to 5 and the upper bound to 20 and lower bound to 0, Spark SQL will retrieve data with the following queries:(stride = (20/5) - (0/5) = 4 SELECT * FROM my_table WHERE partition_column IS NULL OR partition_column < 4 SELECT * FROM my_table WHERE partition_column >= 4 AND partition_column < 8 SELECT * FROM my_table WHERE partition_column >= 8 AND partition_column < 12 SELECT * FROM my_table WHERE partition_column >= 12 AND partition_column < 16 SELECT * FROM my_table WHERE partition_column >= 16
As you see, the above queries generate 5 partitions of data, each containing the values from: (null-3), (4-7), (8-11), (12-15) and (16 and more).
Spark SQL partition example
After this introduction part, it's a good time to see partitioning in action:
var sparkSession: SparkSession = null before { sparkSession = SparkSession.builder().appName("Partitioning test").master("local").getOrCreate() InMemoryDatabase.createTable("CREATE TABLE IF NOT EXISTS orders_sql_partitioning " + "(id INT(11) NOT NULL AUTO_INCREMENT PRIMARY KEY, shop_id INT(1) NOT NULL, " + "customer VARCHAR(255) NOT NULL, amount DECIMAL(6, 2) NOT NULL)") case class Order(shopId: Int, customer: String, amount: Double) extends DataOperation { override def populatePreparedStatement(preparedStatement: PreparedStatement): Unit = { preparedStatement.setInt(1, shopId) preparedStatement.setString(2, customer) preparedStatement.setDouble(3, amount) } } val ordersToInsert = mutable.ListBuffer[Order]() for (shopId <- 0 until 9) { for (i <- 1 to 50) { val amount = ThreadLocalRandom.current().nextDouble(1000) ordersToInsert.append(Order(shopId, UUID.randomUUID().toString, amount)) } } InMemoryDatabase.populateTable("INSERT INTO orders_sql_partitioning (shop_id, customer, amount) VALUES (?, ?, ?)", ordersToInsert) } after { sparkSession.stop() InMemoryDatabase.cleanDatabase() } "the number of partitions" should "be the same as the number of distinct shop_ids" in { val lowerBound = 0 val upperBound = 9 val numberOfPartitions = 9 val jdbcDF = sparkSession.read .format("jdbc") .options(getOptionsMap(numberOfPartitions, lowerBound, upperBound)) .load() jdbcDF.select("shop_id") .foreachPartition(partitionRows => { val shops = partitionRows.map(row => row.getAs[Int]("shop_id")).toSet DataPerPartitionHolder.PartitionsEqualToShopIds.append(shops) }) DataPerPartitionHolder.PartitionsEqualToShopIds.size shouldEqual(9) DataPerPartitionHolder.PartitionsEqualToShopIds(0) should contain only(0) DataPerPartitionHolder.PartitionsEqualToShopIds(1) should contain only(1) DataPerPartitionHolder.PartitionsEqualToShopIds(2) should contain only(2) DataPerPartitionHolder.PartitionsEqualToShopIds(3) should contain only(3) DataPerPartitionHolder.PartitionsEqualToShopIds(4) should contain only(4) DataPerPartitionHolder.PartitionsEqualToShopIds(5) should contain only(5) DataPerPartitionHolder.PartitionsEqualToShopIds(6) should contain only(6) DataPerPartitionHolder.PartitionsEqualToShopIds(7) should contain only(7) DataPerPartitionHolder.PartitionsEqualToShopIds(8) should contain only(8) } // Partitioning logic: // If ($upperBound - $lowerBound >= $nbPartitions) => keep the $nbPartitions // Otherwise reduce the number of partitions to $upperBound - $lowerBound "the number of partitions" should "be reduced when the difference between upper and lower bounds are lower than the " + "number of expected partitions" in { val lowerBound = 0 val upperBound = 2 val numberOfPartitions = 5 val jdbcDF = sparkSession.read .format("jdbc") .options(getOptionsMap(numberOfPartitions, lowerBound, upperBound)) .load() jdbcDF.select("shop_id") .foreachPartition(partitionRows => { val shops = partitionRows.map(row => row.getAs[Int]("shop_id")).toSet DataPerPartitionHolder.DataPerPartitionReducedPartitionsNumber.append(shops) }) DataPerPartitionHolder.DataPerPartitionReducedPartitionsNumber.size shouldEqual(2) DataPerPartitionHolder.DataPerPartitionReducedPartitionsNumber(0) should contain only(0) DataPerPartitionHolder.DataPerPartitionReducedPartitionsNumber(1) should contain allOf(1, 2, 3, 4, 5, 6, 7, 8) } "partitions" should "be divided according to the stride equal to 1" in { val lowerBound = 0 val upperBound = 8 val numberOfPartitions = 5 // Stride = (8/5) - (0/3) ~ 0.533 ~ 1 // We expect 8 partitions and according to the partitioning algorithm, Spark SQL will generate // the queries with following boundaries: // 1) shop_id < 1 OR shop_id IS NULL // 2) shop_id >= 1 AND shop_id < 2 // 3) shop_id >= 2 AND shop_id < 3 // 4) shop_id >= 3 AND shop_id < 4 // 5) shop_id >= 4 val jdbcDF = sparkSession.read .format("jdbc") .options(getOptionsMap(numberOfPartitions, lowerBound, upperBound)) .load() jdbcDF.select("shop_id") .foreachPartition(partitionRows => { val shops = partitionRows.map(row => row.getAs[Int]("shop_id")).toSet DataPerPartitionHolder.DataPerPartitionStridesComputationExample.append(shops) }) DataPerPartitionHolder.DataPerPartitionStridesComputationExample.size shouldEqual(5) DataPerPartitionHolder.DataPerPartitionStridesComputationExample(0) should contain only(0) DataPerPartitionHolder.DataPerPartitionStridesComputationExample(1) should contain only(1) DataPerPartitionHolder.DataPerPartitionStridesComputationExample(2) should contain only(2) DataPerPartitionHolder.DataPerPartitionStridesComputationExample(3) should contain only(3) DataPerPartitionHolder.DataPerPartitionStridesComputationExample(4) should contain allOf(4, 5, 6, 7, 8) } "two empty partitions" should "be created when the upper bound is too big" in { val lowerBound = 0 // Here upperBound is much bigger than the maximum shop_id value (8) // In consequence, empty partitions will be generated val upperBound = 20 val numberOfPartitions = 5 // // Stride = (20/5) - (0/5) = 4 // We expect 8 partitions and according to the partitioning algorithm, Spark SQL will generate // the queries with following boundaries: // 1) shop_id < 4 OR shop_id IS NULL // 2) shop_id >= 4 AND shop_id < 8 // 3) shop_id >= 8 AND shop_id < 12 // 4) shop_id >= 12 AND shop_id < 16 // 5) shop_id >= 16 val jdbcDF = sparkSession.read .format("jdbc") .options(getOptionsMap(numberOfPartitions, lowerBound, upperBound)) .load() jdbcDF.select("shop_id") .foreachPartition(partitionRows => { val shops = partitionRows.map(row => row.getAs[Int]("shop_id")).toSet DataPerPartitionHolder.DataPerPartitionEmptyPartitions.append(shops) }) DataPerPartitionHolder.DataPerPartitionEmptyPartitions.size shouldEqual(5) DataPerPartitionHolder.DataPerPartitionEmptyPartitions(0) should contain allOf(0, 1, 2, 3) DataPerPartitionHolder.DataPerPartitionEmptyPartitions(1) should contain allOf(4, 5, 6, 7) DataPerPartitionHolder.DataPerPartitionEmptyPartitions(2) should contain only(8) DataPerPartitionHolder.DataPerPartitionEmptyPartitions(3) shouldBe empty DataPerPartitionHolder.DataPerPartitionEmptyPartitions(4) shouldBe empty } "the decimal partition column" should "be accepted" in { val lowerBound = 0 val upperBound = 1000 val numberOfPartitions = 2 val jdbcDF = sparkSession.read .format("jdbc") .options(getOptionsMap(numberOfPartitions, lowerBound, upperBound, "amount")) .load() jdbcDF.select("amount") .foreachPartition(partitionRows => { val shops = partitionRows.map(row => row.getAs[BigDecimal]("amount")).toSet DataPerPartitionHolder.DataPerPartitionDecimalColumn.append(shops) println(s">>> ${shops.mkString(",")}") }) DataPerPartitionHolder.DataPerPartitionDecimalColumn.size shouldEqual(2) // Do not make exact assertions since the amount is generated randomly // and the test can fail from time to time DataPerPartitionHolder.DataPerPartitionDecimalColumn(0) should not be (empty) DataPerPartitionHolder.DataPerPartitionDecimalColumn(1) should not be (empty) } private def getOptionsMap(numberOfPartitions: Int, lowerBound: Int, upperBound: Int, column: String = "shop_id"): Map[String, String] = { Map("url" -> InMemoryDatabase.DbConnection, "dbtable" -> "orders_sql_partitioning", "user" -> InMemoryDatabase.DbUser, "password" -> InMemoryDatabase.DbPassword, "driver" -> InMemoryDatabase.DbDriver, "partitionColumn" -> s"${column}", "numPartitions" -> s"${numberOfPartitions}", "lowerBound" -> s"${lowerBound}", "upperBound" -> s"${upperBound}") } object DataPerPartitionHolder { val PartitionsEqualToShopIds = mutable.ListBuffer[Set[Int]]() val DataPerPartitionReducedPartitionsNumber = mutable.ListBuffer[Set[Int]]() val DataPerPartitionStridesComputationExample = mutable.ListBuffer[Set[Int]]() val DataPerPartitionEmptyPartitions = mutable.ListBuffer[Set[Int]]() val DataPerPartitionDecimalColumn = mutable.ListBuffer[Set[BigDecimal]]() }
This post shown how to configure partitioning in Spark SQL working with RDBMS. The first part explained some tricky and unclear parts about the configuration parameters. Especially 2 of them, upper and lower bound, appeared to be important to understand. In fact they'll determine the chunks of data included in each partition. The second part proved that through some learning tests.