The idea of watermark was firstly presented in the occasion of discovering the Apache Beam project. However it's also implemented in Apache Spark to respond to the same problem - the problem of late data.
Data Engineering Design Patterns
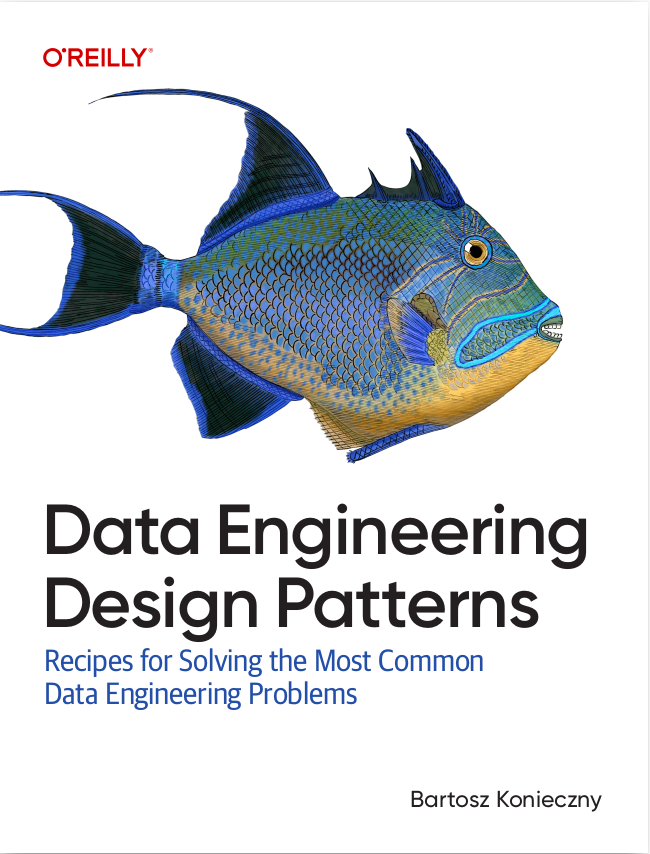
Looking for a book that defines and solves most common data engineering problems? I wrote
one on that topic! You can read it online
on the O'Reilly platform,
or get a print copy on Amazon.
I also help solve your data engineering problems 👉 contact@waitingforcode.com 📩
This post covers the watermark in Apache Spark. The first section explains the theory behind this concept. The second one focuses on the API. Finally in the last part we can discover some examples of watermark executed against a memory stream.
Watermark definition
In Structured Streaming the watermark was introduced as a response to 2 different problems. The first one concerned the late data and was about how old can be the data ingested to the pipeline. The second problem was more about the stateful aggregations. Its core subject was how to limit the amount of state stored in the result table. After all, some of output modes allow an infinitely growing state.
The watermark was then proposed as a mechanism to handle these 2 problems. First, it's able to tell which data can be accepted in the pipeline. Second, it's also able to discard too old state from the state store. The idea of the watermark consists on specify 2 elements: a column defining the event time and a value of delay threshold. Both serve to compute the watermark for given period of time. The computation is based on the subtraction of MAX(event_time) - delay_threshold observed within given micro-batch. So for instance, if the most rececent event within processed batch was observed at 12:55 and the delay threshold is 5 minutes, then only the records newer than or equal to 12:50 will be accepted.
The watermark impacts different features of the Structured Streaming applications:
- output mode - when the output mode doesn't allow the updates, the watermark can be used to control when the final result can be emitted to the result table. One example of it is the append output mode. Output modes will be covered in another post.
- on-going aggregations state - as mentioned shortly in previous paragraphs, the watermark can be used to drop too old state in the case of on-going stateful aggregations as: mapGroupsWithState or dropDuplicates transformations.
- windows - the watermark can be used to caught the late data and put it into the appropriated window.
Watermark API
The use of watermark is quite straightforward since it's defined with Dataset's withWatermark(eventTime: String, delayThreshold: String) method. The first argument represents the column storing the event time while the second one the already explained delay threshold.
We can see the watermark's activity by analyzing the generated logs:
- the entry Filtering state store on: (created#5-T6000ms <= 4000000) cames from org.apache.spark.sql.execution.streaming.WatermarkSupport trait's watermarkExpression attribute. This attribute generates the expression used to match the data older than the watermak. Currently it's mainly used by: FlatMapGroupsWithStateExec, StateStoreSaveExec and StreamingDeduplicateExec to control the amount of memorized state.
- the eventTime property of org.apache.spark.sql.streaming.StreamingQueryProgress instance contains the watermark vaue used in the given micro-batch. This entry can be found with the logs beginning by Streaming query made progress:.
The watermark values are kept in the instance of org.apache.spark.sql.execution.streaming.EventTimeStatsAccum as EventTimeStats object. It's updated by EventTimeWatermarkExec operator.
The information about the watermark is also persisted in the checkpointed offset log file. It's defined for this purpose in org.apache.spark.sql.execution.streaming.OffsetSeqMetadata case class.
Watermark examples
Below you can find the concretization of everything was told before in the usual form of learning tests (even though sometimes they can fail because of the difficulty to test the streaming with almost real sources):
"watermark" should "discard late data and accept 1 late but within watermark with window aggregation" in { val testKey = "watermark-window-test" val inputStream = new MemoryStream[(Timestamp, String)](1, sparkSession.sqlContext) val now = 5000L val aggregatedStream = inputStream.toDS().toDF("created", "name") .withWatermark("created", "2 seconds") .groupBy(window($"created", "2 seconds")).count() val query = aggregatedStream.writeStream.outputMode("update") .foreach(new ForeachWriter[Row]() { override def open(partitionId: Long, version: Long): Boolean = true override def process(processedRow: Row): Unit = { val window = processedRow.get(0) val rowRepresentation = s"${window.toString} -> ${processedRow.getAs[Long]("count")}" InMemoryKeyedStore.addValue(testKey, rowRepresentation) } override def close(errorOrNull: Throwable): Unit = {} }).start() new Thread(new Runnable() { override def run(): Unit = { inputStream.addData((new Timestamp(now), "a1"), (new Timestamp(now), "a2"), (new Timestamp(now-4000L), "b1")) while (!query.isActive) { // wait the query to activate } // The watermark is now computed as MAX(event_time) - watermark, i.e.: // 5000 - 2000 = 3000 // Thus among the values sent above only "a6" should be accepted because it's within the watermark Thread.sleep(7000) val timeOutOfWatermark = 1000L inputStream.addData((new Timestamp(timeOutOfWatermark), "b2"), (new Timestamp(timeOutOfWatermark), "b3"), (new Timestamp(timeOutOfWatermark), "b4"), (new Timestamp(now), "a3")) } }).start() query.awaitTermination(25000) val readValues = InMemoryKeyedStore.getValues(testKey) println(s"All data=${readValues}") // As you can notice, the count for the window 0-2 wasn't updated with 3 fields (b2, b3 and b4) because they fall // before the watermark // Please see how this behavior changes in the next test where the watermark is defined to 10 seconds readValues should have size 3 readValues should contain allOf("[1970-01-01 01:00:00.0,1970-01-01 01:00:02.0] -> 1", "[1970-01-01 01:00:04.0,1970-01-01 01:00:06.0] -> 2", "[1970-01-01 01:00:04.0,1970-01-01 01:00:06.0] -> 3") } "late data but within watermark" should "be aggregated in correct windows" in { val testKey = "watermark-window-test-accepted-data" val inputStream = new MemoryStream[(Timestamp, String)](1, sparkSession.sqlContext) val now = 5000L val aggregatedStream = inputStream.toDS().toDF("created", "name") .withWatermark("created", "10 seconds") .groupBy(window($"created", "2 seconds")).count() val query = aggregatedStream.writeStream.outputMode("update") .foreach(new ForeachWriter[Row]() { override def open(partitionId: Long, version: Long): Boolean = true override def process(processedRow: Row): Unit = { val window = processedRow.get(0) val rowRepresentation = s"${window.toString} -> ${processedRow.getAs[Long]("count")}" InMemoryKeyedStore.addValue(testKey, rowRepresentation) } override def close(errorOrNull: Throwable): Unit = {} }).start() new Thread(new Runnable() { override def run(): Unit = { inputStream.addData((new Timestamp(now), "a1"), (new Timestamp(now), "a2"), (new Timestamp(now-4000L), "b1")) while (!query.isActive) { // wait the query to activate } // The watermark is now computed as MAX(event_time) - watermark, i.e.: // 5000 - 2000 = 3000 // Thus among the values sent above only "a6" should be accepted because it's within the watermark Thread.sleep(7000) val timeOutOfWatermark = 1000L inputStream.addData((new Timestamp(timeOutOfWatermark), "b2"), (new Timestamp(timeOutOfWatermark), "b3"), (new Timestamp(timeOutOfWatermark), "b4"), (new Timestamp(now), "a3")) } }).start() query.awaitTermination(25000) val readValues = InMemoryKeyedStore.getValues(testKey) // As you can notice, the count for the window 0-2 wasn't updated with 3 fields (b2, b3 and b4) because they fall // before the watermark // Please see how this behavior changes in the next test where the watermark is defined to 10 seconds readValues should have size 4 readValues should contain allOf("[1970-01-01 01:00:00.0,1970-01-01 01:00:02.0] -> 1", "[1970-01-01 01:00:00.0,1970-01-01 01:00:02.0] -> 4", "[1970-01-01 01:00:04.0,1970-01-01 01:00:06.0] -> 2", "[1970-01-01 01:00:04.0,1970-01-01 01:00:06.0] -> 3") } "watermark after aggregation" should "not be allowed in append mode" in { val inputStream = new MemoryStream[(Timestamp, String)](1, sparkSession.sqlContext) inputStream.addData((new Timestamp(System.currentTimeMillis()), "a"), (new Timestamp(System.currentTimeMillis()), "b")) val aggregatedStream = inputStream.toDS().toDF("created", "name") .groupBy("created").count() .withWatermark("created", "10 seconds") val exception = intercept[AnalysisException] { val query = aggregatedStream.writeStream.outputMode("append") .foreach(new NoopForeachWriter[Row]()).start() query.awaitTermination(3000) } exception.message should include ("Append output mode not supported when there are streaming aggregations on streaming " + "DataFrames/DataSets without watermark") } "watermark applied on different field than the aggregation in append mode" should "make the processing fail" in { val inputStream = new MemoryStream[(Timestamp, String)](1, sparkSession.sqlContext) inputStream.addData((new Timestamp(System.currentTimeMillis()), "a"), (new Timestamp(System.currentTimeMillis()), "b")) val aggregatedStream = inputStream.toDS().toDF("created", "name") .withWatermark("created", "10 seconds") .agg(count("name")) val exception = intercept[AnalysisException] { // It fails only for append mode. If the watermark is not applied on grouped column it means no more // no less that this group is never finished and then that it would never output anything val query = aggregatedStream.writeStream.outputMode("append") .foreach(new NoopForeachWriter[Row]()).start() query.awaitTermination(3000) } exception.message should include ("Append output mode not supported when there are streaming aggregations on streaming " + "DataFrames/DataSets without watermark") } "watermark in append mode" should "emit the results only after watermark expiration" in { // Accordingly to the Spark's documentation // (https://spark.apache.org/docs/2.2.1/structured-streaming-programming-guide.html#handling-late-data-and-watermarking) // in the append mode the partial results are not updated. Only the final result is computed // and emitted to the result table: // ``` // Similar to the Update Mode earlier, the engine maintains intermediate counts for each window. // However, the partial counts are not updated to the Result Table and not written to sink. // The engine waits for “10 mins” for late date to be counted, then drops intermediate state of a // window < watermark, and appends the final counts to the Result Table/sink. // ``` // But please notice that in the append output mode the results // are emitted once the watermark passed. Here the results for 00:00:10 will be // emitted only when the watermark will pass to 00:00:19 (00:00:10 is now before the watermark), i.e. after updating // the watermark for the record (24000L, 5). val testKey = "watermark-append-mode" val inputStream = new MemoryStream[(Timestamp, Int)](1, sparkSession.sqlContext) val now = 5000L val aggregatedStream = inputStream.toDS().toDF("created", "name") .withWatermark("created", "5 second") .groupBy("created") .count() val query = aggregatedStream.writeStream.trigger(Trigger.ProcessingTime("2 seconds")).outputMode("append") .foreach(new ForeachWriter[Row]() { override def open(partitionId: Long, version: Long): Boolean = true override def process(processedRow: Row): Unit = { val rowRepresentation = s"${processedRow.getAs[Timestamp]("created")} -> ${processedRow.getAs[Long]("count")}" InMemoryKeyedStore.addValue(testKey, rowRepresentation) println(s"Processing ${rowRepresentation} at ${new Date(System.currentTimeMillis())}") } override def close(errorOrNull: Throwable): Unit = {} }).start() new Thread(new Runnable() { override def run(): Unit = { // send the first batch - max time = 10 seconds, so the watermark will be 5 seconds inputStream.addData((new Timestamp(now+5000), 1), (new Timestamp(now+5000), 2), (new Timestamp(now+5000), 3), (new Timestamp(now+5000), 4)) while (!query.isActive) { // wait the query to activate } Thread.sleep(4000) inputStream.addData((new Timestamp(now), 6), (new Timestamp(6000), 7)) Thread.sleep(4000) inputStream.addData((new Timestamp(24000L), 8)) // Only to update the watermark Thread.sleep(4000) inputStream.addData((new Timestamp(4000L), 9)) Thread.sleep(4000) inputStream.addData((new Timestamp(now), 10)) } }).start() query.awaitTermination(45000) val readValues = InMemoryKeyedStore.getValues(testKey) readValues should have size 2 readValues should contain allOf("1970-01-01 01:00:10.0 -> 4", "1970-01-01 01:00:06.0 -> 1") } "the watermark" should "be used in aggregations others than windowing" in { val testKey = "watermark-count-aggregation" val inputStream = new MemoryStream[(Timestamp, Int)](1, sparkSession.sqlContext) val now = 5000L val aggregatedStream = inputStream.toDS().toDF("created", "number") .withWatermark("created", "1 second") .groupBy("created") .count() val query = aggregatedStream.writeStream./*trigger(Trigger.ProcessingTime("2 seconds")).*/outputMode("update") .foreach(new ForeachWriter[Row]() { override def open(partitionId: Long, version: Long): Boolean = true override def process(processedRow: Row): Unit = { val rowRepresentation = s"${processedRow.getAs[Timestamp]("created")} -> ${processedRow.getAs[Long]("count")}" InMemoryKeyedStore.addValue(testKey, rowRepresentation) } override def close(errorOrNull: Throwable): Unit = {} }).start() new Thread(new Runnable() { override def run(): Unit = { // send the first batch - max time = 10 seconds, so the watermark will be 9 seconds inputStream.addData((new Timestamp(now+5000), 1), (new Timestamp(now+5000), 2), (new Timestamp(now+5000), 3), (new Timestamp(now+5000), 4)) while (!query.isActive) { // wait the query to activate } // Here the rows#5 and #6 are ignored because of the watermark // In the other side, the row#7 will be taken into account // To see what happens when the watermark doesn't exist, you can uncomment the line .withWatermark... // Without the watermark, the counter for *now* will be updated Thread.sleep(10000) inputStream.addData((new Timestamp(4000L), 5)) inputStream.addData((new Timestamp(now), 6), (new Timestamp(11000), 7)) } }).start() query.awaitTermination(45000) val readValues = InMemoryKeyedStore.getValues(testKey) println(s"All data=${readValues}") // As you can notice, the count for the window 0-2 wasn't updated with 3 fields (b2, b3 and b4) because they fall // before the watermark // Please see how this behavior changes in the next test where the watermark is defined to 10 seconds readValues should have size 2 readValues should contain allOf("1970-01-01 01:00:10.0 -> 4", "1970-01-01 01:00:11.0 -> 1") } "the data arriving after the watermark and the state older than the watermark" should "not be discarded correctly" in { val testKey = "watermark-deduplicate" val testKeyLastProgress = "watermark-deduplicate-last-progress" val inputStream = new MemoryStream[(Timestamp, Int)](1, sparkSession.sqlContext) val now = 5000L val aggregatedStream = inputStream.toDS().toDF("created", "number") .withWatermark("created", "6 seconds") .dropDuplicates("number", "created") val query = aggregatedStream.writeStream.trigger(Trigger.ProcessingTime("2 seconds")).outputMode("update") .foreach(new ForeachWriter[Row]() { override def open(partitionId: Long, version: Long): Boolean = true override def process(processedRow: Row): Unit = { val rowRepresentation = s"${processedRow.getAs[Timestamp]("created").toString} -> ${processedRow.getAs[Int]("number")}" InMemoryKeyedStore.addValue(testKey, rowRepresentation) println(s"processing ${rowRepresentation}") } override def close(errorOrNull: Throwable): Unit = {} }).start() new Thread(new Runnable() { override def run(): Unit = { // Events sent before - they should be correctly deduplicated, i.e. (5000, 1), (5000, 2) and (10000, 2) // should be taken // As you can observe, the deduplication occurs with the pair (event time, value) inputStream.addData((new Timestamp(now), 1), (new Timestamp(now), 2), (new Timestamp(now), 1), (new Timestamp(now+5000), 2)) while (!query.isActive) { // wait the query to activate } logInfo(s"Query was activated, sleep for 9 seconds before sending new data. Current timestamp " + s"is ${System.currentTimeMillis()}") Thread.sleep(11000) logInfo(s"Awaken at ${System.currentTimeMillis()} where the query status is ${query.lastProgress.json}") // In the logs we can observe the following entry: // ``` // Filtering state store on: (created#5-T6000ms <= 4000000) // (org.apache.spark.sql.execution.streaming.StreamingDeduplicateExec:54) // ``` // As you can correctly deduce, among the entries below: // - 1, 2 and 3 will be filtered // - 4 will be accepted // Moreover, the 4 will be used to compute the new watermark. Later in the logs we can observe the following: // ``` // Filtering state store on: (created#5-T6000ms <= 6000000) // (org.apache.spark.sql.execution.streaming.StreamingDeduplicateExec:54) // ``` inputStream.addData((new Timestamp(now), 1), (new Timestamp(now-1000), 2), (new Timestamp(now-3000), 3), (new Timestamp(now+7000), 4)) Thread.sleep(9000) // Here the value 1 is after the watermark so automatically discarded InMemoryKeyedStore.addValue(testKeyLastProgress, query.lastProgress.json) inputStream.addData((new Timestamp(now), 1)) Thread.sleep(7000) inputStream.addData((new Timestamp(now), 1)) InMemoryKeyedStore.addValue(testKeyLastProgress, query.lastProgress.json) } }).start() query.awaitTermination(55000) val accumulatedValues = InMemoryKeyedStore.getValues(testKey) accumulatedValues should have size 4 accumulatedValues should contain allOf("1970-01-01 01:00:10.0 -> 2", "1970-01-01 01:00:05.0 -> 1", "1970-01-01 01:00:05.0 -> 2", "1970-01-01 01:00:12.0 -> 4") val seenProgresses = InMemoryKeyedStore.getValues(testKeyLastProgress) // This progress represents the moment where all 4 rows are considered as within the watermark val initialProgress = seenProgresses(0) initialProgress should include("\"stateOperators\":[{\"numRowsTotal\":4,\"numRowsUpdated\":0}]") initialProgress should include("\"eventTime\":{\"watermark\":\"1970-01-01T00:00:04.000Z\"}") // This progress represents the moment where 2 rows (1970-01-01 01:00:05.0 -> 1 and 1970-01-01 01:00:05.0 -> 2) // were removed from the state store because of the watermark expiration val progressWithRemovedStates = seenProgresses(1) progressWithRemovedStates should include("\"stateOperators\":[{\"numRowsTotal\":2,\"numRowsUpdated\":0}]") progressWithRemovedStates should include("\"eventTime\":{\"watermark\":\"1970-01-01T00:00:06.000Z\"}") }
The watermark in Apache Spark is the mechanism helping to dropping too old state and discarding too old data. As explained in the first section, it's present in a lot of places: windows, stateful aggregations and output modes. It's defined quite simply with the withWatermark(...) method. Its activity can be found in the logs thanks to the entries listed in the second section of the post. The last part shown in its turn, how the watermark was interacting with Apache Spark transformations and output modes through the usual learning tests.
Consulting
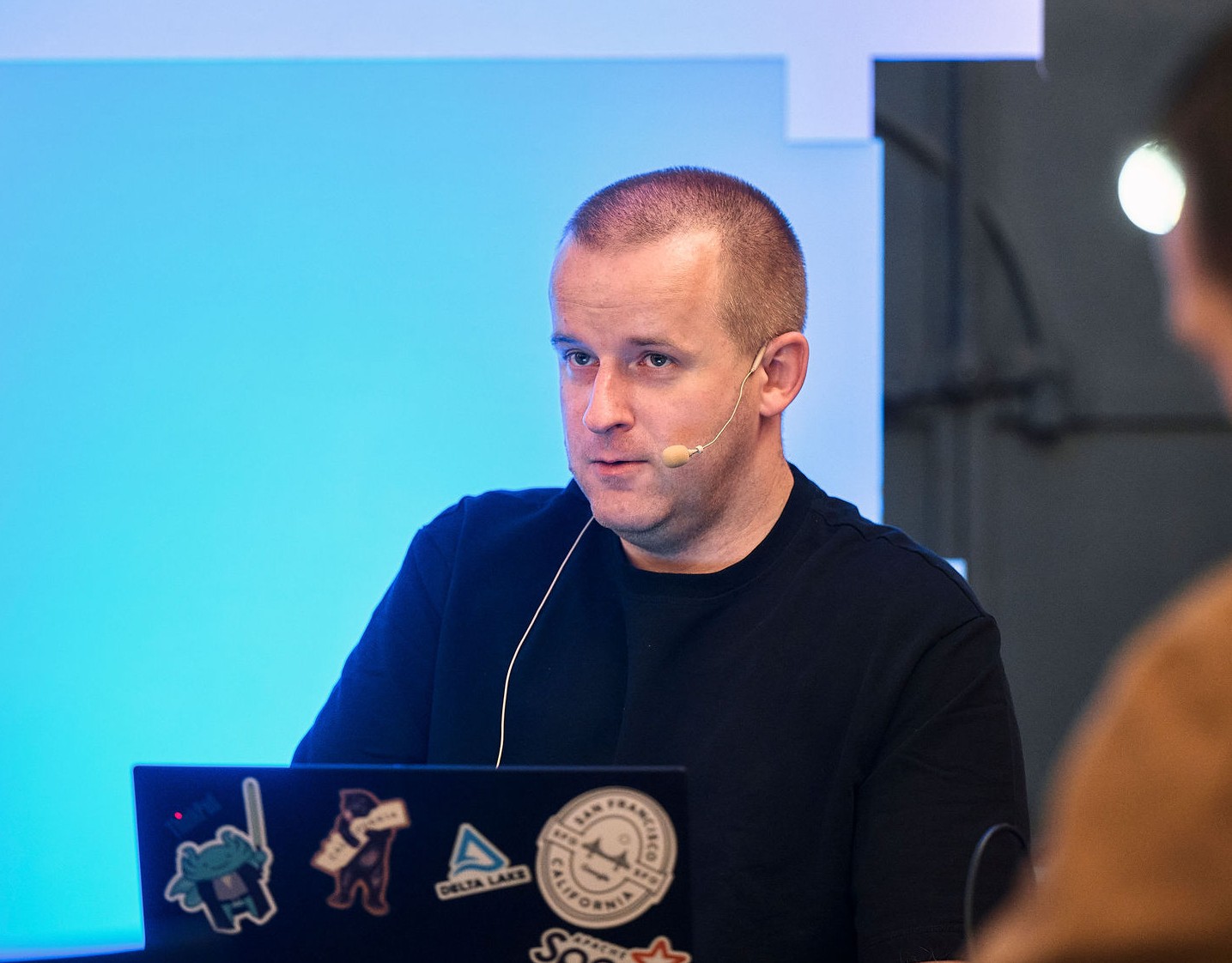
With nearly 16 years of experience, including 8 as data engineer, I offer expert consulting to design and optimize scalable data solutions.
As an O’Reilly author, Data+AI Summit speaker, and blogger, I bring cutting-edge insights to modernize infrastructure, build robust pipelines, and
drive data-driven decision-making. Let's transform your data challenges into opportunities—reach out to elevate your data engineering game today!
👉 contact@waitingforcode.com
đź”— past projects