In the previous post about Apache Spark SQL custom optimizations I presented a rule transforming UNION operator into JOIN. At this time I only did a simple version working only with 2 datasets. In this post, I will share its improved version.
Data Engineering Design Patterns
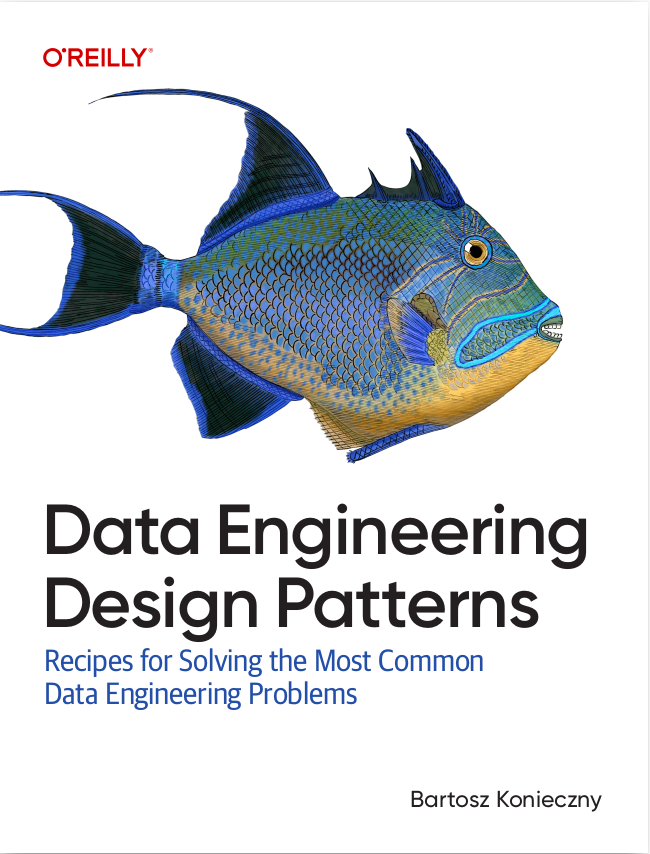
Looking for a book that defines and solves most common data engineering problems? I wrote
one on that topic! You can read it online
on the O'Reilly platform,
or get a print copy on Amazon.
I also help solve your data engineering problems 👉 contact@waitingforcode.com 📩
The post starts by showing the improved version which applies on more than 2 UNIONs. The second part gives a summary of all classes and abstractions I had to use to write the code.
Improved UNION rewritter
Initially I thought that adapting the code from the previous week's post to the case of multiple UNIONs will be complicated. First, I wanted to catch the highest UNION operator and recursively transform all its children into JOINs. However, that was not a good idea because the transform() method behaves exactly like a graph traversal. Therefore, I already have the possibility to reach and transform every node. My second thought was "maybe I will catch the UNIONs and transform only its 2 closest children". The only thing I had to verify was the state of the transformed plan. I wanted to ensure that the transformed logical plan is broadcasted up or down in the transform method. This test case proves that it's the case:
"transformation method" should "move modified plan to the next level" in { val letterReference = AttributeReference("letter", StringType, false)() val nameReference = AttributeReference("name", StringType, false)() val ageReference = AttributeReference("age", IntegerType, false)() val selectExpressions = Seq[NamedExpression]( letterReference, nameReference ) val dataset = LocalRelation(Seq(letterReference, nameReference, ageReference)) val project1 = Project(selectExpressions, dataset) val project2 = Project(selectExpressions, dataset) val join = Join(project1, project2, JoinType("fullouter"), Option(And(selectExpressions(0), selectExpressions(1)))) val projectionsFromJoin = new scala.collection.mutable.ListBuffer[Project]() // transform is called only once; // the goal is to show you that the modified plan is passed to next levels join.transformUp { case project: Project => { project.copy(projectList = project.projectList ++ project.projectList) } case join: Join => { join.children.filter(childPlan => childPlan.isInstanceOf[Project]) .foreach(childPlan => { projectionsFromJoin.append(childPlan.asInstanceOf[Project]) }) join } } val expectedFields = Seq("letter", "name", "letter", "name") projectionsFromJoin.foreach(projection => { projection.projectList.map(namedExpression => namedExpression.name) should contain allElementsOf expectedFields }) }
As you can observe in the test, the transformed Project is directly passed to upper levels of the logical plan. Thanks to this confirmation I could apply my idea of transforming tuples:
object UnionAdvancedOptimizer extends Rule[LogicalPlan] { override def apply(logicalPlan: LogicalPlan): LogicalPlan = logicalPlan transformUp { case distinct: Distinct if distinct.child.isInstanceOf[Union] => { val union = distinct.child.asInstanceOf[Union] val joinColumns = union.children.map { case localRelation: LocalRelation => localRelation.output case project: Project => project.output } val joinPairs = joinColumns.transpose val equalToExpressions = joinPairs.map(attributes => { EqualTo(attributes(0), attributes(1)) }) def concatExpressions(expression: Expression, remainingExpressions: Seq[Expression]): Expression = { if (remainingExpressions.isEmpty) { expression } else { concatExpressions(And(expression, remainingExpressions.head), remainingExpressions.tail) } } val concatenatedExpressions = concatExpressions(equalToExpressions.head, equalToExpressions.tail) val projection1 = Project(joinColumns(0), union.children(0)) val projection2 = Project(joinColumns(1), union.children(1)) val join1 = Join(projection1, projection2, JoinType("fullouter"), Option(concatenatedExpressions)) val projection = combineProjection(joinPairs, join1) projection } } private def combineProjection(joinAttributes: Seq[Seq[Attribute]], childPlan: Join): Project = { val fields = joinAttributes.map(attributes => { Alias(Coalesce(attributes), attributes(0).name)() }) Project(fields, childPlan) } }
If you remember my previous post, you can see that the code didn't evolve a lot. The only difference is the used transformation method. Since I wanted to avoid to recursively convert UNIONs into JOINs, I preferred this time to start from the bottom and be able to find the pairs of projections at every level (remember: our rewriter returns a projection on top of the JOIN). You can see the optimizer in action in the following test:
private val sparkSession: SparkSession = SparkSession.builder().appName("Advanced UNION hint test").master("local[*]") .withExtensions(extensions => { // I should use here an optimizer rule but I should then apply it on an HashAggregate + Union which is // less understandable than Union + Distinct extensions.injectResolutionRule(_ => UnionAdvancedOptimizer) }) .getOrCreate() "multiple UNION" should "be rewritten to JOINs" in { import sparkSession.implicits._ val dataset1 = Seq(("A", 1, 1), ("B", 2, 1), ("C", 3, 1), ("D", 4, 1), ("E", 5, 1)).toDF("letter", "nr", "a_flag") val dataset2 = Seq(("F", 10, 1), ("G", 11, 1), ("H", 12, 1)).toDF("letter", "nr", "a_flag") val dataset3 = Seq(("A", 20, 1), ("B", 21, 1), ("C", 22, 1)).toDF("letter", "nr", "a_flag") val dataset4 = Seq(("A", 20, 1), ("B", 21, 1), ("C", 22, 1)).toDF("letter", "nr", "a_flag") dataset1.createOrReplaceTempView("dataset_1") dataset2.createOrReplaceTempView("dataset_2") dataset3.createOrReplaceTempView("dataset_3") dataset4.createOrReplaceTempView("dataset_4") val rewrittenQuery = sparkSession.sql("SELECT letter, nr, a_flag FROM dataset_1 " + "UNION SELECT letter, nr, a_flag FROM dataset_2 " + "UNION SELECT letter, nr, a_flag FROM dataset_3 UNION SELECT letter, nr, a_flag FROM dataset_4") val unionDataset = rewrittenQuery.map(row => s"${row.getAs[String]("letter")}-${row.getAs[Int]("nr")}-${row.getAs[Int]("a_flag")}").collect() unionDataset should have size 11 unionDataset should contain allOf("A-1-1", "A-20-1", "B-2-1", "B-21-1", "C-3-1", "C-22-1", "D-4-1", "E-5-1", "F-10-1", "G-11-1", "H-12-1") rewrittenQuery.queryExecution.executedPlan.toString should include("SortMergeJoin") }
The code has still some points to improve like filtering but I won't going into this right now and instead, I will rather move to the physical plans.
Used classes and abstractions
Last time I shared with you some takeaways about writing a custom optimization. Now I would like to complete this list with the abstractions that you may need to implement it:
- NamedExpression - I found it for the first time when I wrote custom Alias taking the first non-null value from both projections. And more globally, this class represents every operator that can be named, so for instance an alias but also an attribute which can be a column selected in Project operator.
- BinaryExpression - the name is quite meaningful. This expression takes 2 input expressions to generate a single output. An example of it in UNION rewritter is org.apache.spark.sql.catalyst.expressions.And, used to build ON clause of the JOIN.
- Attribute - as mentioned, it's a single attribute. It can reference the attribute produced by other operator, in such a case you will use an implementation called AttributeReference. But it can also be an OuterReference which points to the attribute resolved outside of the current plan, like for instance in correlated subquery.
- UnaryNode and BinaryNode - respectively, a logical plan with a single child and with 2 children. The example of the former one is Project which in our test case was resolved from a LocalRelation. The implementation of the latter class is Join which, as you saw, uses 2 different projections.
- Unevaluable - I met it during my first try when I used UnresolvedAlias. Generally, this trait represents everything that cannot be evaluated, therefore physically executed. Normally if you use it, the unresolved elements should be resolved by one of Resolve* rules like ResolveAliases, ResolveMissingReferences or ResolveFunctions.
That's all for writing a custom resolution rule. But that's not finished for Apache Spark SQL customization. In the next post, I will explore other customization rules and make the exercise of implementing them.
Consulting
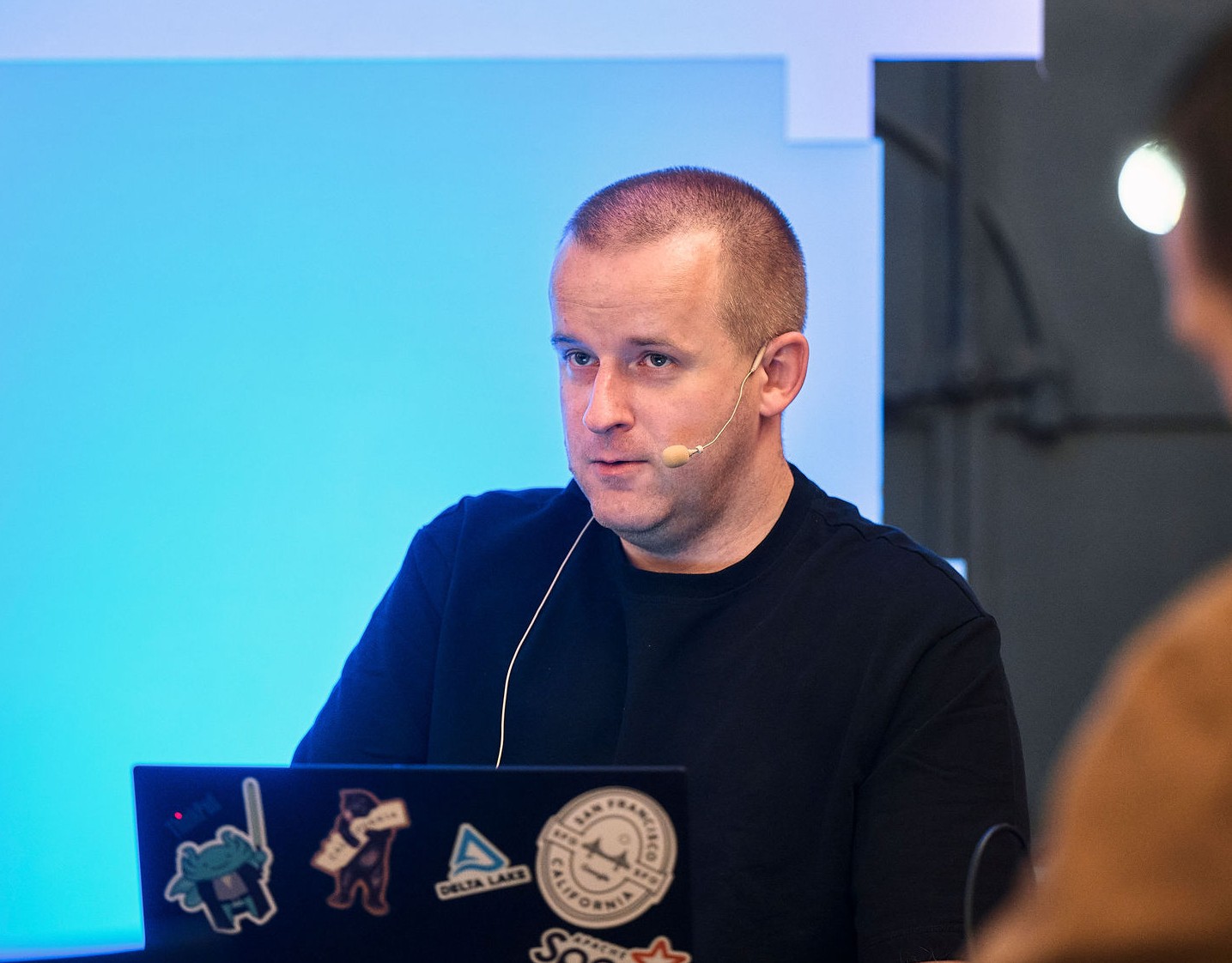
With nearly 16 years of experience, including 8 as data engineer, I offer expert consulting to design and optimize scalable data solutions.
As an O’Reilly author, Data+AI Summit speaker, and blogger, I bring cutting-edge insights to modernize infrastructure, build robust pipelines, and
drive data-driven decision-making. Let's transform your data challenges into opportunities—reach out to elevate your data engineering game today!
👉 contact@waitingforcode.com
đź”— past projects