A good CI/CD process avoids many pitfalls related to manual operations. Apache Spark also has one based on Github Actions. Since this part of the project has been a small mystery for me, I wanted to spend some time exploring it.
Data Engineering Design Patterns
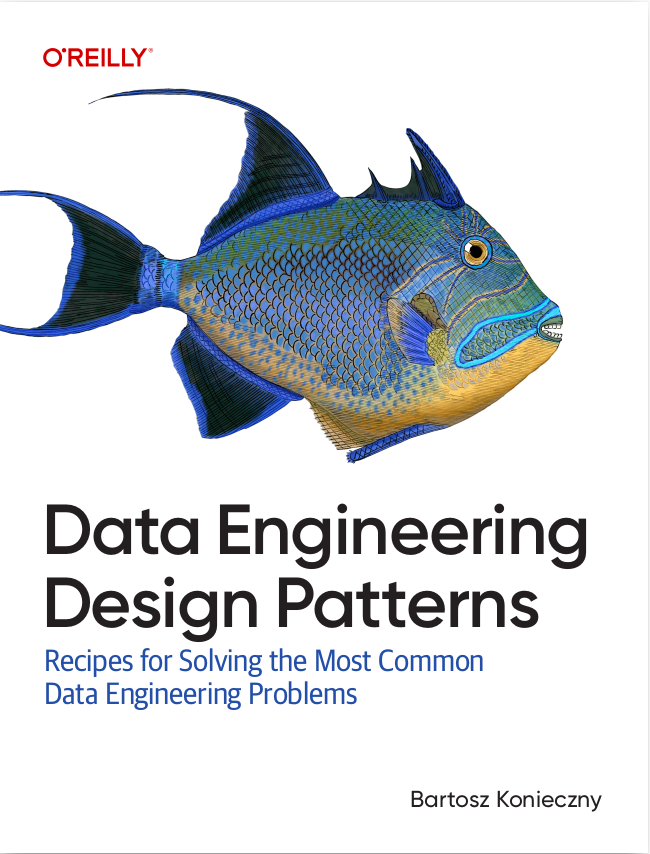
Looking for a book that defines and solves most common data engineering problems? I wrote
one on that topic! You can read it online
on the O'Reilly platform,
or get a print copy on Amazon.
I also help solve your data engineering problems 👉 contact@waitingforcode.com 📩
Workflows
I'll start with Github Actions workflows. The first builds the project and runs the tests on top of it. The workflow is present in the Apache Spark Github project but can also run in any forked repository. It's even recommended in the Developer tools documentation to run the Build and test workflow in the forked repository before creating the PullRequest to avoid burdening the limited Github Actions resources allocated to the Apache Spark project. Besides this manual execution, the workflow also runs after pushing the changes to any branch.You'll find its definition in the build_and_test.yml file.
The Build and test workflow triggers another one called Report test results that pushes test results to the Pull Request as a summary and a PR check. Below you can find an example coming from a Hyukjin Kwon's contribution:
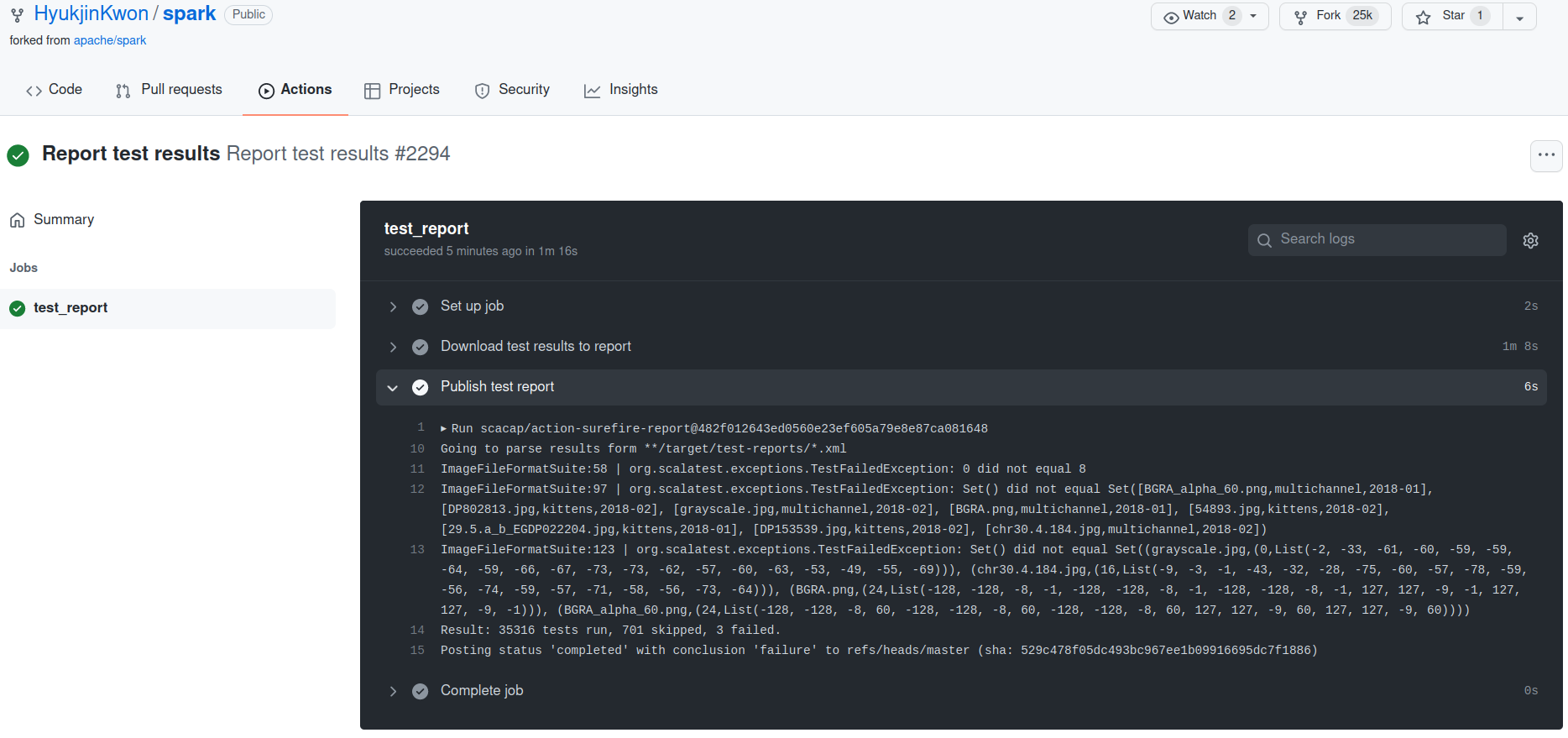
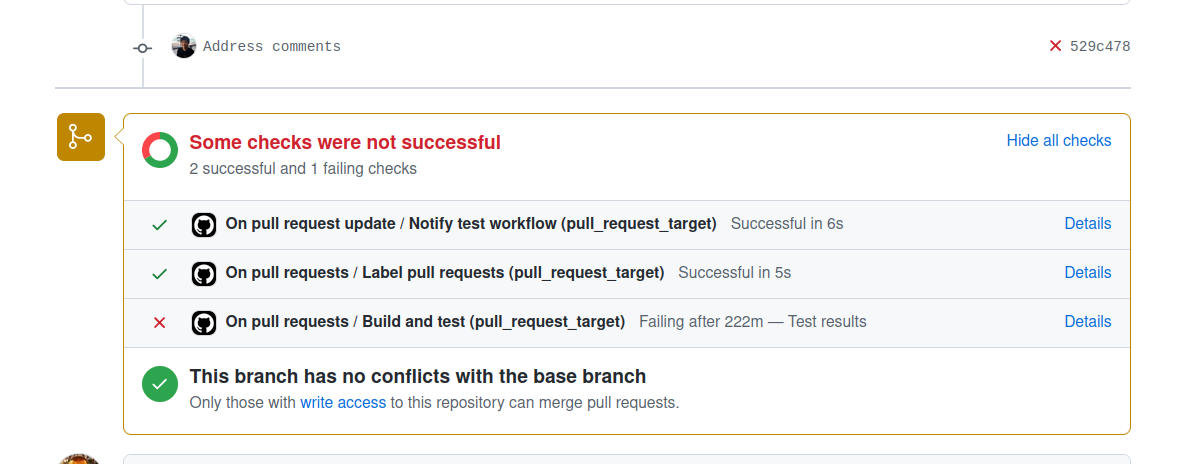
These 2 PR-related automations are not the single ones for the new changes to merge into the main branch. There is also a workflow responsible for automatically labeling the Pull Request. I've always wondered how it works, and it happens to be quite simple. The labeling uses a Github Labeler plugin that matches the modified files against the path patterns defined in the .github/labeler.yml and from that, it automatically associates the labels to the Pull Request.
Besides the aforementioned workflows, Apache Spark has a few others, this time in an alphabetical order:
- benchmark.yml to run benchmark code defined in the org.apache.spark.benchmark.Benchmarks class. The task generates benchmark results for the specified classes and the Pull Request authors are asked to update the corresponding files in Git, as for example below where Dongjoon Hyun updated the results of BLASBenchmark:
- cancel_duplicate_workflow_runs.yml workflow stops duplicated "Build and test" runs for the Pull Requests to reduce the pressure on the Github Action resources.
- notify_test_workflow.yml to automatically start the "Build and test" workflow in the fork repository when a Pull Request gets opened, reopened, or synchronized.
- publish_snapshot.yml generates a -SNAPSHOT distribution every midnight and pushes it to the Apache Software Foundation snapshot repository.
- stale.yml to automatically close Pull Request having 100 days without an activity.
- update_build_status.yml runs every 15 minutes and synchronizes the to Pull Requests status with the "Build and test" results from the forked repository. Previously it added a comment in the Pull Request, now it adds a green/yellow/red icon (success/in progress/failed).
Others
But Apache Spark ops is not only about workflows. I discovered a few other interesting concepts:
- .idea/vcs.xml contains a configuration for the Pull Request and JIRA links you can visualize in the IntelliJ once you open the Apache Spark project:
- appveyor.yml that runs SparkR tests on Windows. The tests execute on the instance managed by Appveyor service.
- create-release directory with the scripts used to create a new release. It's particularly complex compared to the previous files, so let me list some of the scripts just below:
- generate-contributors.py - that's the proof that having strong project conventions you can make complex stuff easy. Generally, the commits to the main branch of the project are always prefixed with [SPARK-xxx] where xxx is the number of JIRA ticket related to the committed work. The script relies on this information to generate a list of contributors with the work they've done in the released version.
- release-build.sh - the script to create an Apache Spark deliverable from a Git commit hash. It handles the scenarios like publishing a release or a snapshot, or validating the Release Candidate by creating a new tag on Git, and pushing the package to PyPI and Apache Software Foundation release repository.
- templates - besides the executables, the directory also contains some template files used by the release managers to announce the new version or a new Release Candidate.
When it comes to the code itself, the project leverages some external tools to ensure code quality. PySpark uses Mypy for types checking and Coverage.py for code coverage generation, whereas Scala-Spark ensures code consistency with Scalastyle module.
Apache Spark is an established Open Source project, and proper ops tooling was a part of its success. I hope that the article showed you something new that you might find interesting to integrate into your internal projects!
Consulting
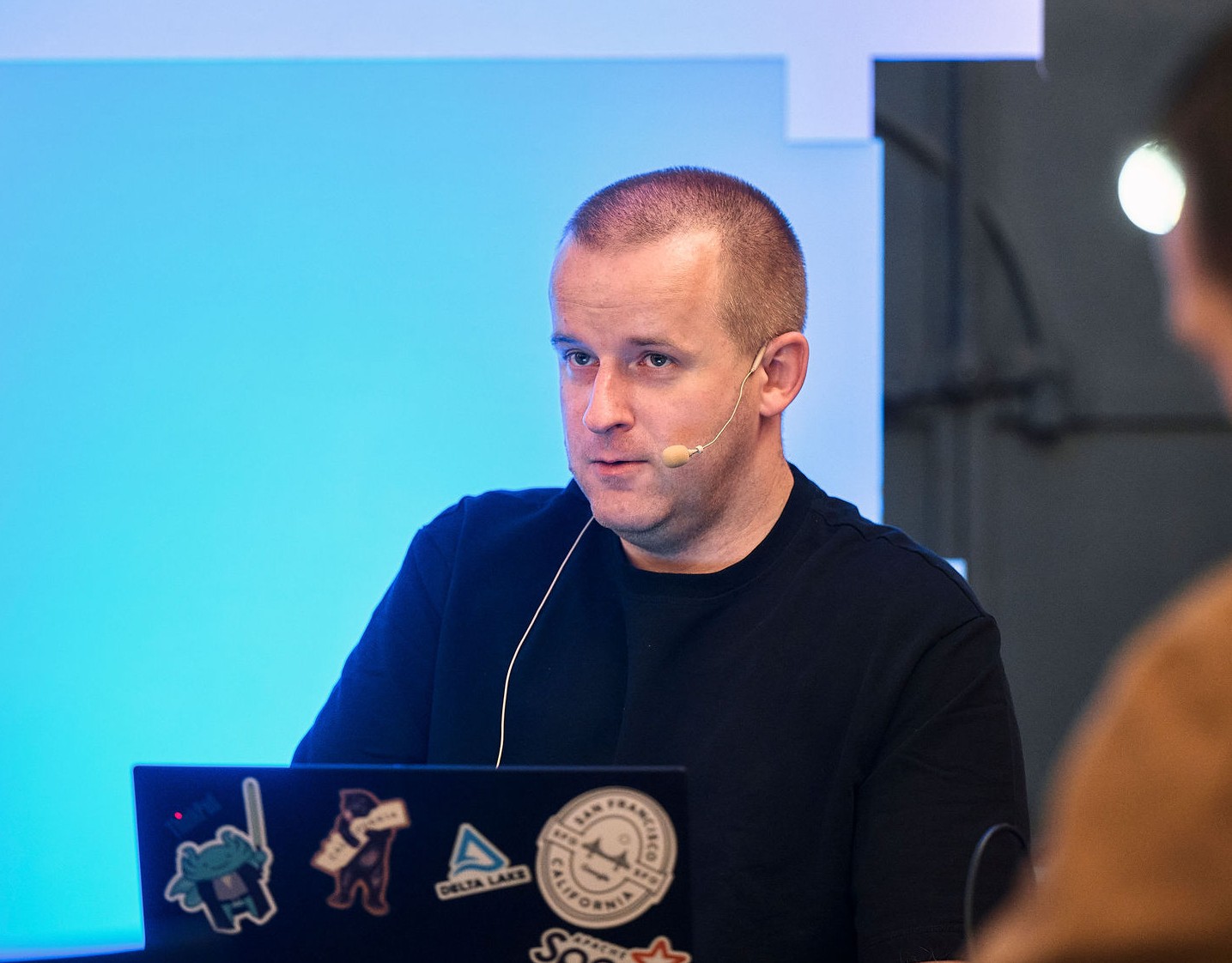
With nearly 16 years of experience, including 8 as data engineer, I offer expert consulting to design and optimize scalable data solutions.
As an O’Reilly author, Data+AI Summit speaker, and blogger, I bring cutting-edge insights to modernize infrastructure, build robust pipelines, and
drive data-driven decision-making. Let's transform your data challenges into opportunities—reach out to elevate your data engineering game today!
👉 contact@waitingforcode.com
đź”— past projects