Setting a data processing layer up has several phases. You need to write the job, define the infrastructure, CI/CD pipeline, integrate with the data orchestration layer, ... and finally, ensure the job can access the relevant datasets. The most basic authentication mechanism uses login/password pair but can we do better on the cloud? Let's see!
Data Engineering Design Patterns
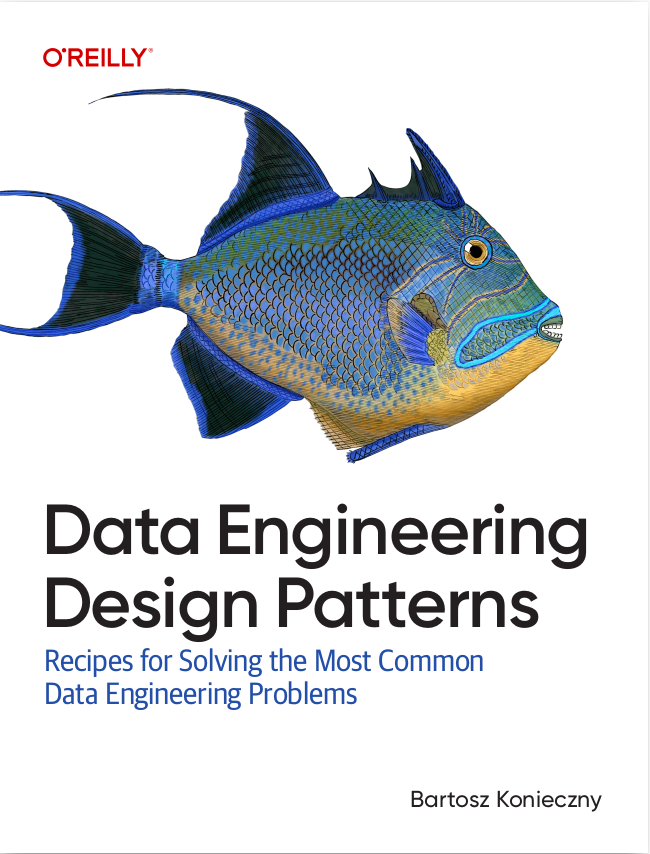
Looking for a book that defines and solves most common data engineering problems? I wrote
one on that topic! You can read it online
on the O'Reilly platform,
or get a print copy on Amazon.
I also help solve your data engineering problems 👉 contact@waitingforcode.com 📩
In this example I'm considering one data processing offering available on each major cloud provider, so Databricks for Azure, EMR for AWS, and Dataproc for GCP.
Service credentials
Using the service credentials is the easiest way to connect to other cloud services. In that configuration the data processing job uses the identity attached to the cluster. As a result, there is no need to embed anything sensitive in the code.
The implementation may be different from provider to another and not always fully covering all available services:
- AWS EMR - an EMR cluster requires service role for EMR (aka EMR role) and Amazon EC2 instance profile. The service role applies for the actions executed when provisioning the cluster resources, such as creating network connections between the cluster nodes. The instance profile is the role assumed by EMR applications, including Apache Spark jobs, to interact with other AWS services.
- Azure Databricks - the support for cloud identity-based authentication is limited to the Unity Catalog and Azure Data Lake Storage Gen2. For other scenarios, you can use Service Principals but this approach does require managing secrets.
- GCP Dataproc - a Dataproc cluster relies on the VM service account to provide credential-less access to other cloud resources.
Among the pros of this solution you can find:
- Credential-less code. Such code is easier to maintain because you get rid of the secure credentials storage management. Additionally, it's easier to deploy in various environments.
- At-least privilege enforcement. You can define a custom access scope and easily respect the at-least privilege principle.
- Maintenance. With cloud access abstractions such as roles, you can mutualize access policies and reduce the day-to-day management overhead.
Although for me it should be the preferred way to interact with cloud services, it's not always possible to set up. There are other methods that might be more adapted for other scenarios.
Multi-tenancy
Multi-tenant clusters were popular in the on-premise era and they become less and less used in the modern cloud-based data processing. However, there still might be a need to use them for whatever reason.
Besides the classical credential-based authentication, the multi-tenant clusters can also rely on the service credentials. But differently. EMR has an Amazon EMR User Role Mapper that you can run as a bootstrap action to create mappings between users or groups and customized IAM roles.
On GCP Dataproc the multi-tenancy support is similar but it's a component available at the cluster creation time. It requires passing the mapping between users and service accounts in the --secure-multi-tenancy-user-mapping or --identity-config-file flag.
Same cloud provider but a different account
Multi-tenancy is not the single more complex data access scenario. Another one is about getting data from the same cloud provider but a different account. AWS EMR relies on the assume role mechanism. Consequently, it involves adding allow policy for sts:AssumeRole action in both accounts and changing the default credentials provider of the job to com.amazonaws.emr.AssumeRoleAWSCredentialsProvider.
This configuration is a bit simpler for GCP Dataproc. The single different step from the usual permissions management is the enabling of the service account impersonation across projects by turning off the iam.disableCrossProjectServiceAccountUsage project constraint. Once it's done, you'll be able to attach a service account to a resource in a different project.
Different cloud provider
That's the final and probably the toughest scenario where you have a job in one cloud provider and must read data from another one. The easiest solution here is to use credentials in the code, such as Shared Access Signatures (typically Azure), signed URLs (AWS S3, GCP GCS), or classical login/password method.
Using that type of access management has different challenges than the ones presented above. First, you must ensure that the credentials don't leak. It means storing them encrypted with only the necessary services authorized reading them. It also means not leaking them inadvertently by putting them in the logged messages or as input parameters visible from the cloud provider's UI.
Besides this privacy challenge, there is another one. You must be able to rotate the credentials if needed, so be able to easily use the new generated access keys. The likelihood of losing old credentials is bigger than for the regularly recreated ones.
With the service credentials approach, the cloud workloads are simplified. You no more need to store things in the code or a secret store. The service directly uses the cloud-managed identity service to make your job interaction with other services possible. However, the things get complicated for less usual scenarios where you might need to deal with multi-tenancy, multi-account, or multi-cloud.
Consulting
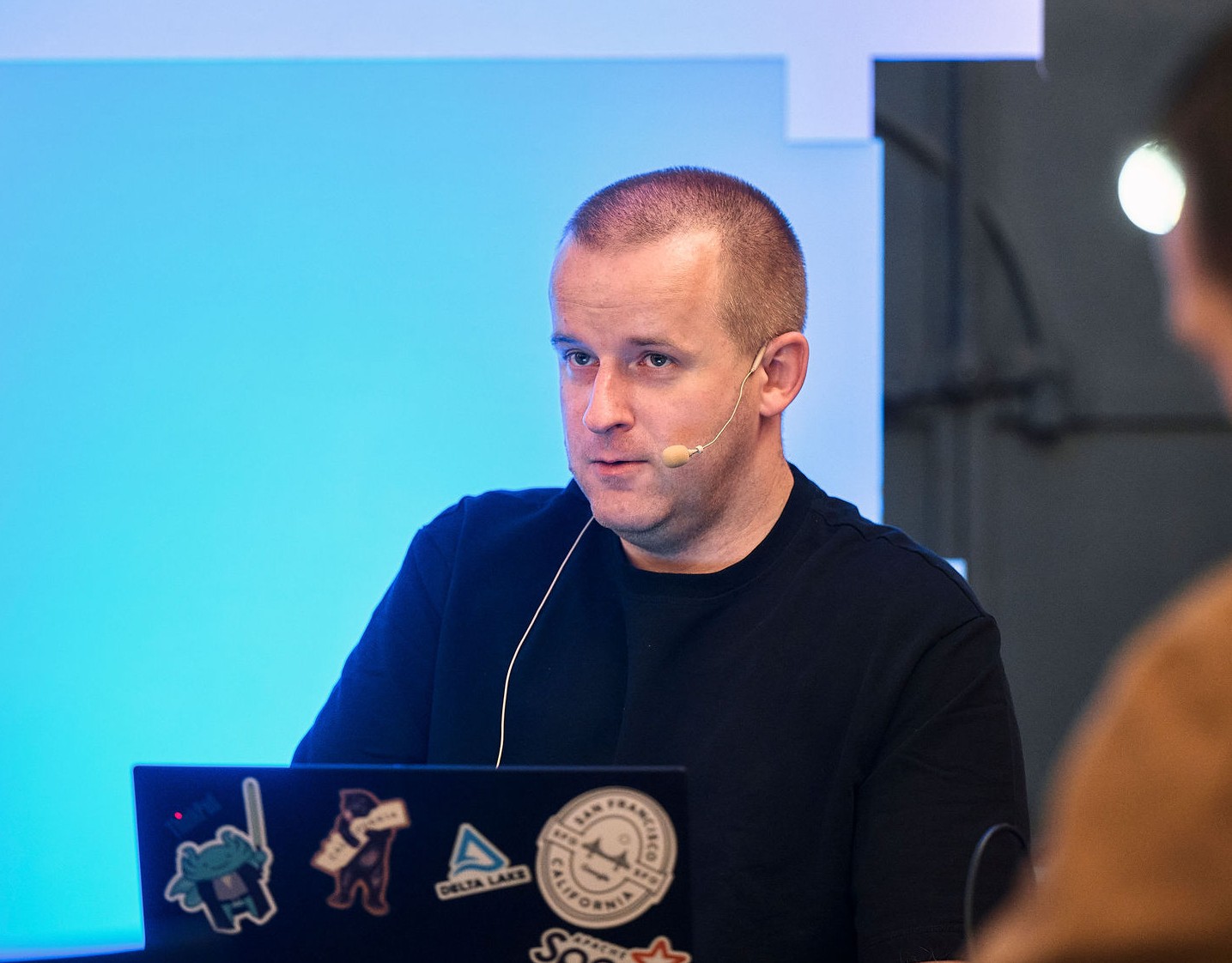
With nearly 16 years of experience, including 8 as data engineer, I offer expert consulting to design and optimize scalable data solutions.
As an O’Reilly author, Data+AI Summit speaker, and blogger, I bring cutting-edge insights to modernize infrastructure, build robust pipelines, and
drive data-driven decision-making. Let's transform your data challenges into opportunities—reach out to elevate your data engineering game today!
👉 contact@waitingforcode.com
đź”— past projects