When I've first met the Complex Event Processing (CEP) term, I was scared. Event streaming processing itself was complex enough, so why this extra complex-specific stuff? It happens that the complexity is real but in this post I will rather focus on a different aspect. What are the services supporting the CEP on the cloud?
Data Engineering Design Patterns
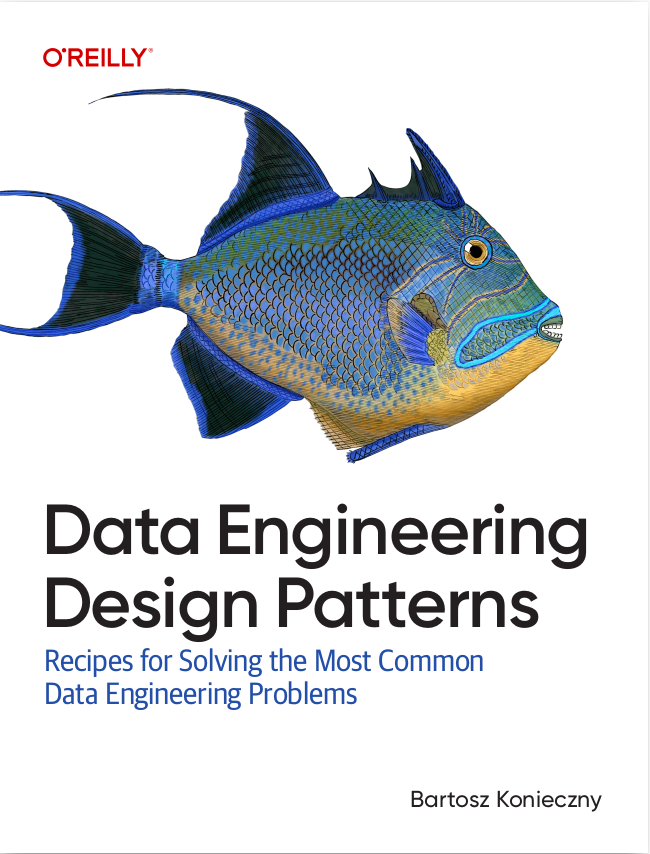
Looking for a book that defines and solves most common data engineering problems? I wrote
one on that topic! You can read it online
on the O'Reilly platform,
or get a print copy on Amazon.
I also help solve your data engineering problems 👉 contact@waitingforcode.com 📩
Complex?
Before covering the cloud aspect, let me focus on the CEP itself. The first thing to clarify is the meaning of this complex keyword. I understood it only after reading the excellent, although quite old, blog post from Octo blog about CEP.
In a nutshell, the CEP starts by complex because:
- low latency - usually, it's the first requirement. CEP systems often solve complex problems, such as anomaly detection in the IoT domain or fraud prevention in financial systems. Having real-time feedback is a must to take advantage of these pipelines fully.
- stateful - stateful processing is not easy, and the mentioned anomaly detection is often the matter of patterns. In other words, there is a sequence of events that probably will lead to some problems. This pattern-based character requires stateful processing and involves related problems like out-of-order or incomplete events.
- data sources combination - often, there will be multiple inputs to combine. Simple joins between streams because of different latency can be a difficult task.
- advanced features - such as partial patterns detection for an infinite state also contribute to the complexity of the CEP pipelines.
The goal of a CEP pipeline is to generate actionable items corresponding to the solved problem. Often, the output will go to a streaming broker because of its real-time semantic. However, it's not forbidden to write these results to a data-at-rest storage for an ad-hoc analysis. Anyway, I hope the "complex" is now clearer and we can move to the cloud architectures.
Cloud services
In the exercise of designing our own architecture, we'll use the following components:
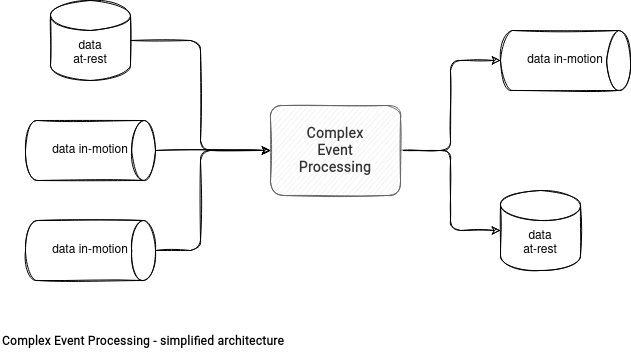
The connectivity of the CEP component is the key element in the analyzed design. We want here to use a cloud service. As you certainly know, managed solutions have their limits and do not magically support all popular data stores. That's why this data processing layer will define the input and output data stores. Let's analyze a few candidates:
- AWS Kinesis Data Analytics for Apache Flink. The solution provides a managed runtime for Apache Flink. It naturally supports AWS services (S3, Kinesis Firehose, Kinesis Data Streams) and other sources and sinks defined in the API. Moreover, if you want to extend the list of supported data stores, you can do it by leveraging the Apache Flink API! Apache Flink also has a built-in module for Complex Event Processing.
- GCP Dataflow. Although it's a fully managed runtime, it shares the Open Source character of Apache Flink. The service API only supports Apache Beam, so an Open Source framework. In addition to the already existing connectors, it can then support any custom data store. I didn't find any built-in CEP module, though.
- AWS EMR, GCP Dataproc, Azure Databricks. All these data processing services can run Apache Spark Structured Streaming pipelines, so an Open Source code that can be extended as for Flink and Beam. However, the implementation will require some custom coding, probably with arbitrary stateful processing. Apache Spark doesn't have a CEP module either.
- AWS Lambda, Azure Functions, GCP Cloud Functions. These serverless functions are also candidates for the compute environment. However, here the coding effort will be much more serious. Besides the CEP logic, you'll have to deal with state checkpointing and shuffle. Put another way, you'll have to provide an abstraction to group related events and persist the current state of the computation to avoid losing it after the function's timeout.
- Azure Stream Analytics. The service supports pattern matching with the MATCH_RECOGNIZE function. It's like a RegEx for events because it supports similar things, like the main pattern, the number of occurrences for each subpattern, etc. The service also supports User-Defined Functions to implement any unsupported column transformation with the code. However, it cannot be extended by extra sources and sinks.
So, which one to choose? Thanks to the native Complex Event Processing module, extendibility, and support on the cloud services, Apache Flink seems to be the best option. But in reality, it depends on the cloud provider and the use case. If you're an Azure user and want to detect some simple patterns, Azure Stream Analytics can be enough. On the other hand, writing some code and deploying a Structured Streaming job can be the single option for other scenarios.
Consulting
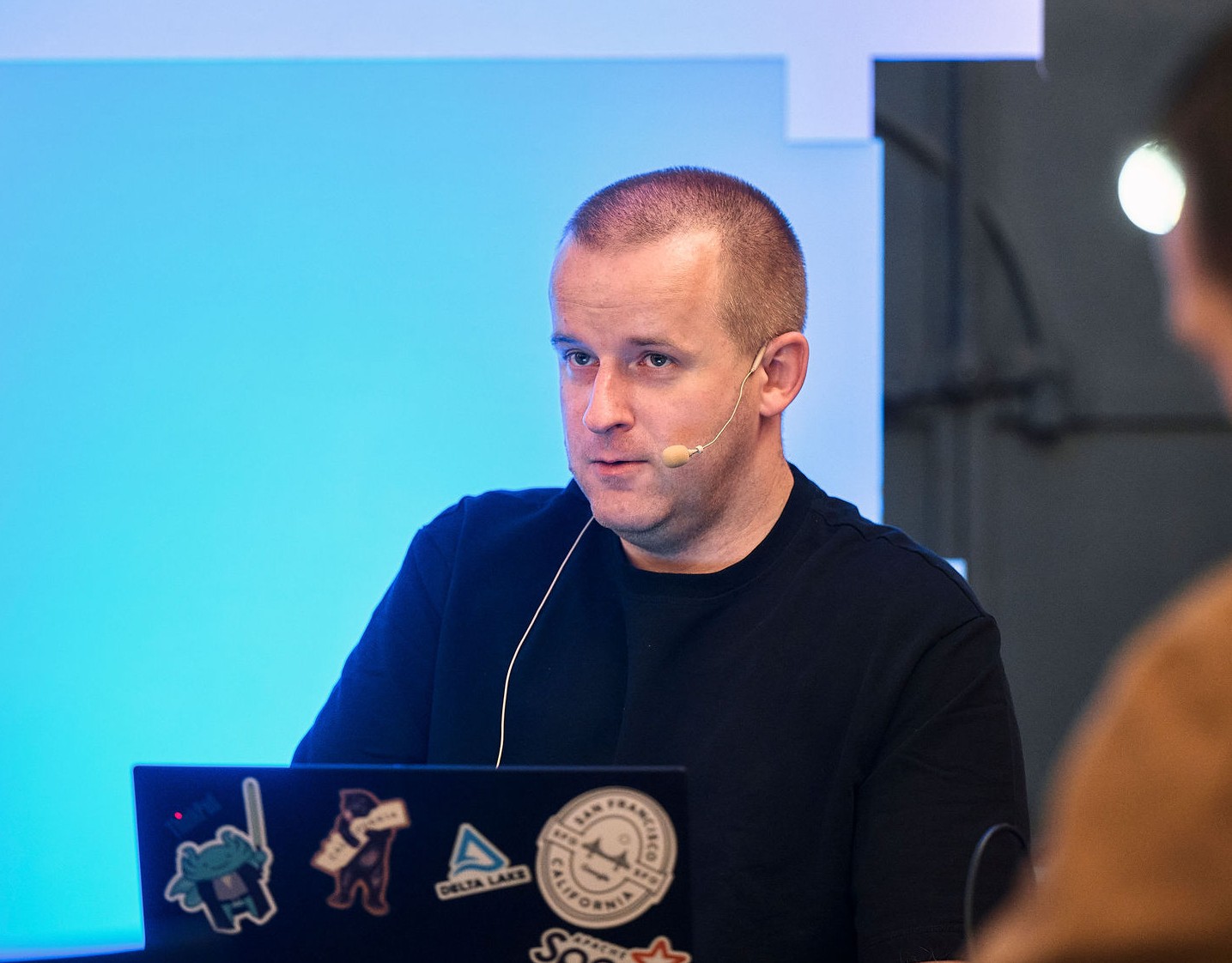
With nearly 16 years of experience, including 8 as data engineer, I offer expert consulting to design and optimize scalable data solutions.
As an O’Reilly author, Data+AI Summit speaker, and blogger, I bring cutting-edge insights to modernize infrastructure, build robust pipelines, and
drive data-driven decision-making. Let's transform your data challenges into opportunities—reach out to elevate your data engineering game today!
👉 contact@waitingforcode.com
đź”— past projects