Data migration is one of the scenarios you can face as a data engineer. It's not always an easy task but managed cloud services can help you to put in place the pipeline and solve many common problems.
Data Engineering Design Patterns
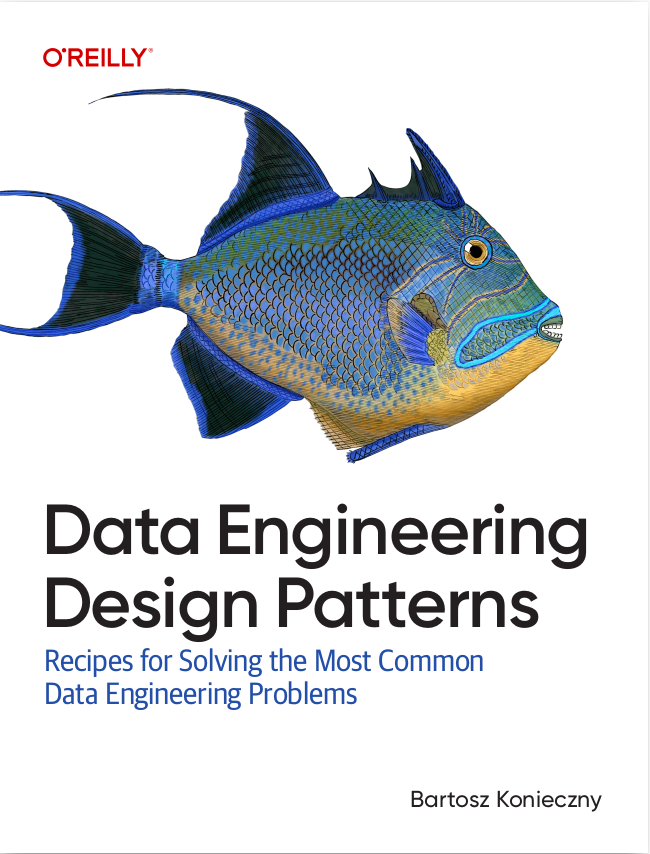
Looking for a book that defines and solves most common data engineering problems? I wrote
one on that topic! You can read it online
on the O'Reilly platform,
or get a print copy on Amazon.
I also help solve your data engineering problems 👉 contact@waitingforcode.com 📩
Database migration
A database migration is not a topic that we could summarize in a few sentences. It has multiple characteristics, such as
- Data type. The migration can be heterogeneous or homogeneous, depending on the types of the source and target databases. If both have the same type, we talk about a homogeneous migration. Heterogeneous migration is its opposite. For example, migrating a document database to a relational database would be heterogeneous, while a relational database to relational database migration would be homogeneous.
- Database type. As for the data type but this time applied to the database. For example, a migration between 2 PostgreSQL instances would be homogeneous, whereas between PostgreSQL and MySQL heterogeneous, despite the same data type.
- Mode. From that standpoint, the migration can be offline or online. An offline migration means that the source database will go offline during the process. The online mode is the opposite, where the source remains available for the consumers.
- Cardinality. Database migration can be:
- a consolidation where multiple databases are merged into a single database
- a distribution where one database distributes its data to multiple different databases
- a re-distribution where multiple databases distribute the data to multiple databases
- Writability. Here, the migration distinguishes 2 types, Active/Active and Active/Passive. In the former, consumers can write against the source and target databases. In the latter, only the source database accepts writes.
- Methods. Generally, you'll find here 2 migration techniques: the full and incremental load. As the name indicates, the full load always takes all the data. It can be good for small tables, like dimension tables but less efficient for big datasets. Big datasets should migrate easier with the incremental load that synchronizes only the changed rows. To implement this strategy you can use differential queries where one column determines a new record, or a Change Data Capture that continuously streams all new data-related operations.
- Technical solution. Multiple types of tools exist to implement the migration. It can be a dedicated database migration service, a native database replication feature, or a custom application.
Data migration is the most obvious component of a database migration but is not the single one. Generally, we can also distinguish the following major steps:
- Initial assessment. Understand the current data, detect potential schema incompatibilities, or necessary types conversions.
- Schema definition. Define the target schema that can be different depending on the migration type.
- Data migration. After the initial setup, it's time to move the data.
- Migration assessment. You should verify whether the data migrated correctly. It's a good moment to check for any data consistency issues, such as null values, truncated fields, missing or duplicated rows.
- Switchover. It's an optional step where all the clients start to use the new database.
Database migration on the cloud
Let's see now what cloud services we can use in the data migration scenario:
AWS Database Migration Service |
Azure Data Factory |
Azure Data Share |
GCP Database Migration Service |
GCP Datastream |
|
---|---|---|---|---|---|
Data type | Heterogeneous and homogeneous scenarios are supported. For example, the service can migrate data from an RDBMS to DynamoDB. | Heterogeneous and homogeneous scenarios are supported with CopyActivity action. For example, you can copy a relational data from SQL Server to columnar Parquet files stored on a Storage Account, as well as from an on-premise MySQL to an Azure SQL Database. | Homogeneous and heterogeneous types are supported. | Homogeneous scenario is supported. It's limited to RDBMS data. | Heterogeneous scenarios is supported. The service writes RDBMS data to GCS. |
Database type | Both heterogeneous and homogeneous scenarios are covered. For example, you can migrate a PostgreSQL data to MySQL. | Heterogeneous and homogeneous scenarios are supported with CopyActivity action. | Homogeneous and heterogeneous types are supported. | Homogeneous scenario. The service supports MySQL and PostgreSQL migrations. | Heterogeneous scenario. The service synchronizes RDBMS to GCS. |
Methods | Change Data Capture and full loads. | Full and incremental loads with Change Data Capture or a delta column. | In the snapshot mode, the service supports full and incremental modes. | Continuous and one-time migrations are supported. | Continuous migration based on Change Data Capture. |
Schema migration | AWS comes with a AWS Schema Conversion Tool to migrate the schemas. | Supported in the mapping tab of the CopyActivity. | No. | No. | The service maps the schema from the RDBMS sources to unified types. Consequently, it represents all the sources with the same types in the synchronized files. |
Data transformation | The replication task can have a Transformation rule. It can operate at the table or column level and for example, rename any of these objects. It also supports some expressions, such as columns concatenation or nullable values coalescing. | The service supports datetime formatting, data truncation, language-based types conversion in the CopyActivity. Additionally, Data Factory can use other types of activities to perform more customized data transformations. | No. | No. | No. |
Data migration might seem a very popular topic in the to-cloud migration context. However, we can migrate the data in other scenarios as well. One of such situations is data access specialization, where we move the data to optimize its querying. And it happens that you can perform this task on all of the major cloud providers!
Consulting
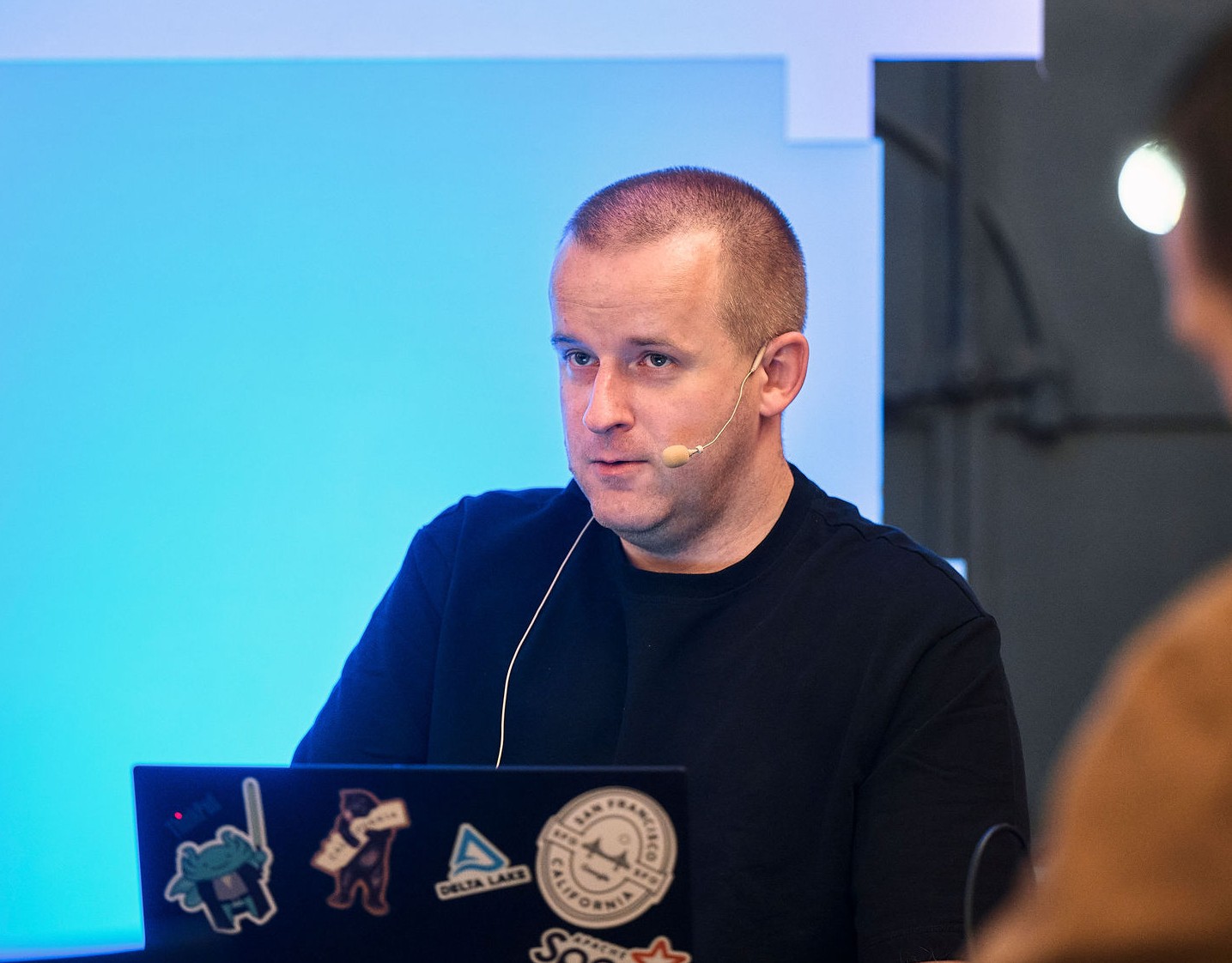
With nearly 16 years of experience, including 8 as data engineer, I offer expert consulting to design and optimize scalable data solutions.
As an O’Reilly author, Data+AI Summit speaker, and blogger, I bring cutting-edge insights to modernize infrastructure, build robust pipelines, and
drive data-driven decision-making. Let's transform your data challenges into opportunities—reach out to elevate your data engineering game today!
👉 contact@waitingforcode.com
đź”— past projects