One of patterns that you may implement in batch ETL is sequential execution. It means that the output of one job execution is a part of the input for the next job execution. Even though Apache Airflow comes with 3 properties to deal with the concurrence, you may need another one to avoid bad surprises.
Data Engineering Design Patterns
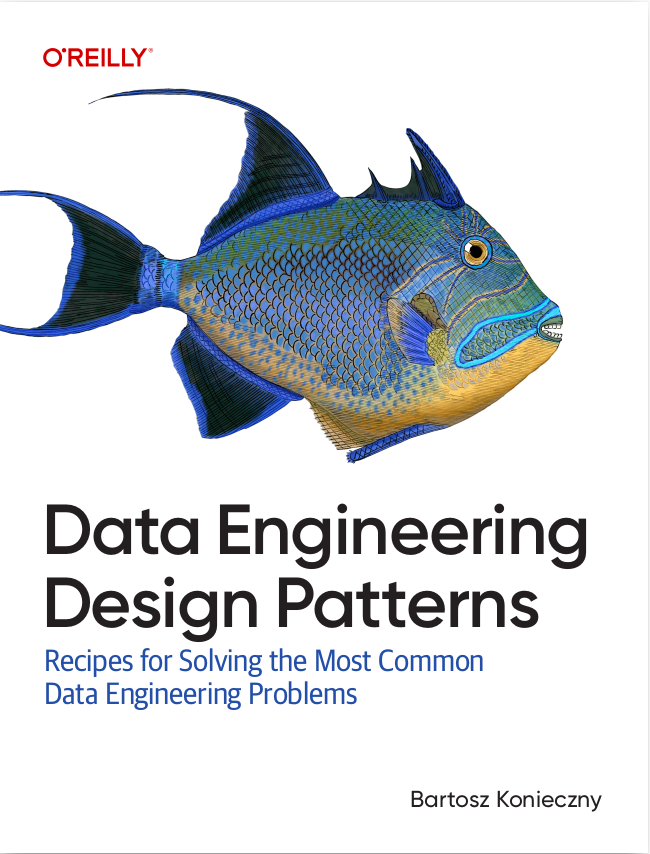
Looking for a book that defines and solves most common data engineering problems? I wrote
one on that topic! You can read it online
on the O'Reilly platform,
or get a print copy on Amazon.
I also help solve your data engineering problems 👉 contact@waitingforcode.com 📩
This post starts by describing 3 properties that you can use to control the concurrency of your Apache Airflow workloads. By the end of this section, you will see that using them is not enough to guarantee the sequential character of the pipelines. Only the next section will present an improved solution that should work for most of the use cases.
3 properties to control concurrency
The 3 properties from the title are:
- depends_on_past - this task-level property defines whether given task execution depends on the result of the past task execution. Sounds unclear? Let's take a sample DAG and see how depends_on_past looks on the job executions diagram:
dag = DAG( dag_id='concurrency_dag', default_args={ 'start_date': airflow.utils.dates.days_ago(1), 'depends_on_past': True, }, schedule_interval=timedelta(minutes=1) ) with dag: task_1 = DummyOperator(task_id='task_1') task_2 = DummyOperator(task_id='task_2') task_3 = DummyOperator(task_id='task_3') task_4 = DummyOperator(task_id='task_4') task_1 >> task_2 >> task_3 >> task_4
The following screenshot shows how the DAG behaves when it's running: - wait_for_downstream - this property is quite similar to depends_on_past unless it refers to the downstream tasks. In other words, it means that if all tasks (task1) -> (task2) -> (task3) are waiting for downstream, the second execution of task2 will wait for the first execution of task1, the second execution of task3 will wait for the first execution of task2 and so on.
After adding this property to default arguments of tested DAG (depends_on_past will be automatically set to True), the execution graph looks like: - max_active_runs - this DAG-level property enforces that at given moment there will be only one task instance executed. Alongside the 2 previously presented properties, when max_active_runs is set to 1, it gives an impression of sequentiality:
Why it's only an impression? Let's take an example of an ephemeral EMR cluster that starts, executes a job and destroys the resource. Depending on your DAG construction, you may end up with such bad situation when, despite the failure of the previous DAG execution, your cloud resource is up and running, and it's unable to stop because of broken task dependencies:
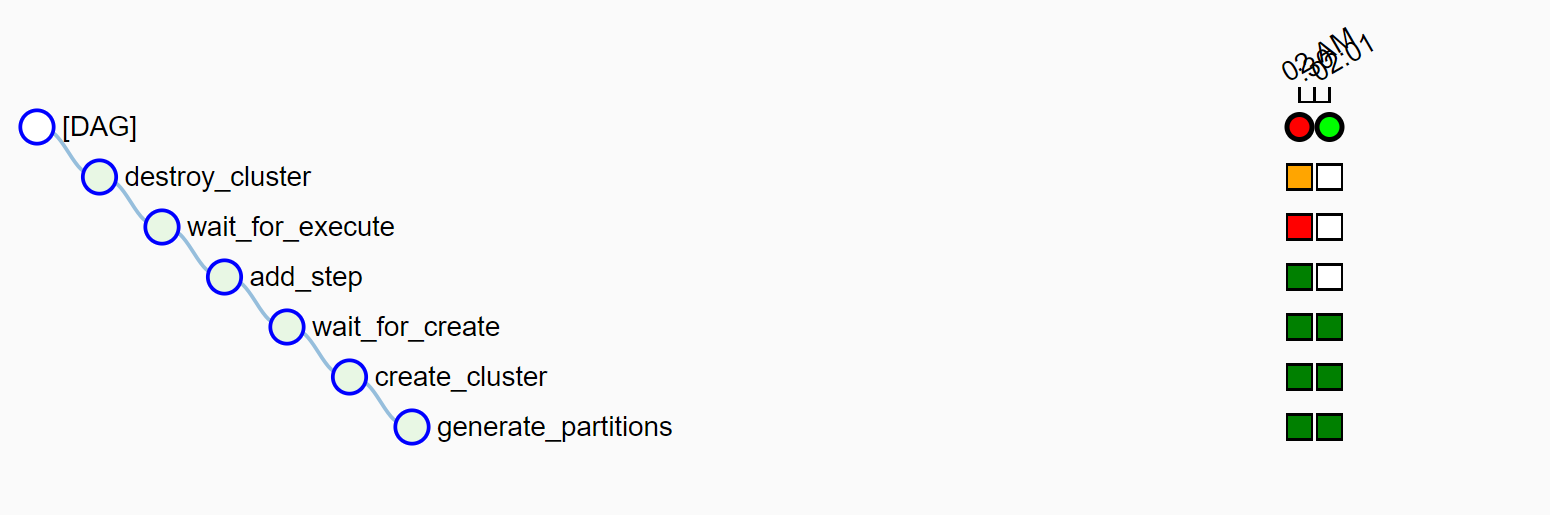
When the 3 properties are not enough
As you can see, sometimes using these 3 properties won't be enough. Even though one specific task will be blocked, meantime you waste your budget on not used resources. To solve the problem, you can use sensors. In the example, we're starting the DAG by generating partition information. So, a simple check could be a DAG like this:

It will work but you will encounter a problem for the first execution. In that case, you can either mark the first task as successful or add a branch to check whether Airflow is executing the DAG for the first time or not.
Another solution, sharing the problem of the first execution though, could consist on using ExternalTaskSensor. As the name indicates, the sensor is used to listen for the execution status for the specified task:
with dag: previous_run_waiter = ExternalTaskSensor( task_id='wait_for_previous', external_dag_id=dag.dag_id, # execution_delta - used to get the instance of the # previous DAG execution; if task is croned every minute, # it should be a minute. Unless you've dependencies on other # tasks from the past execution_delta=timedelta(minutes=1), external_task_id='last_task') processing_task = DummyOperator(task_id='processing_task') last_task = DummyOperator(task_id='last_task') previous_run_waiter >> processing_task ... >>> last_task
I hope you understand better now the subtleties of sequential execution of DAGs in Apache Airflow. As you can learn at the beginning, it's hard to enforce sequentiality only with Airflow's properties. However, it's a little bit simpler with sensors, either the ones listening for data presence or directly with the ones waiting for given task instances to succeed.
Consulting
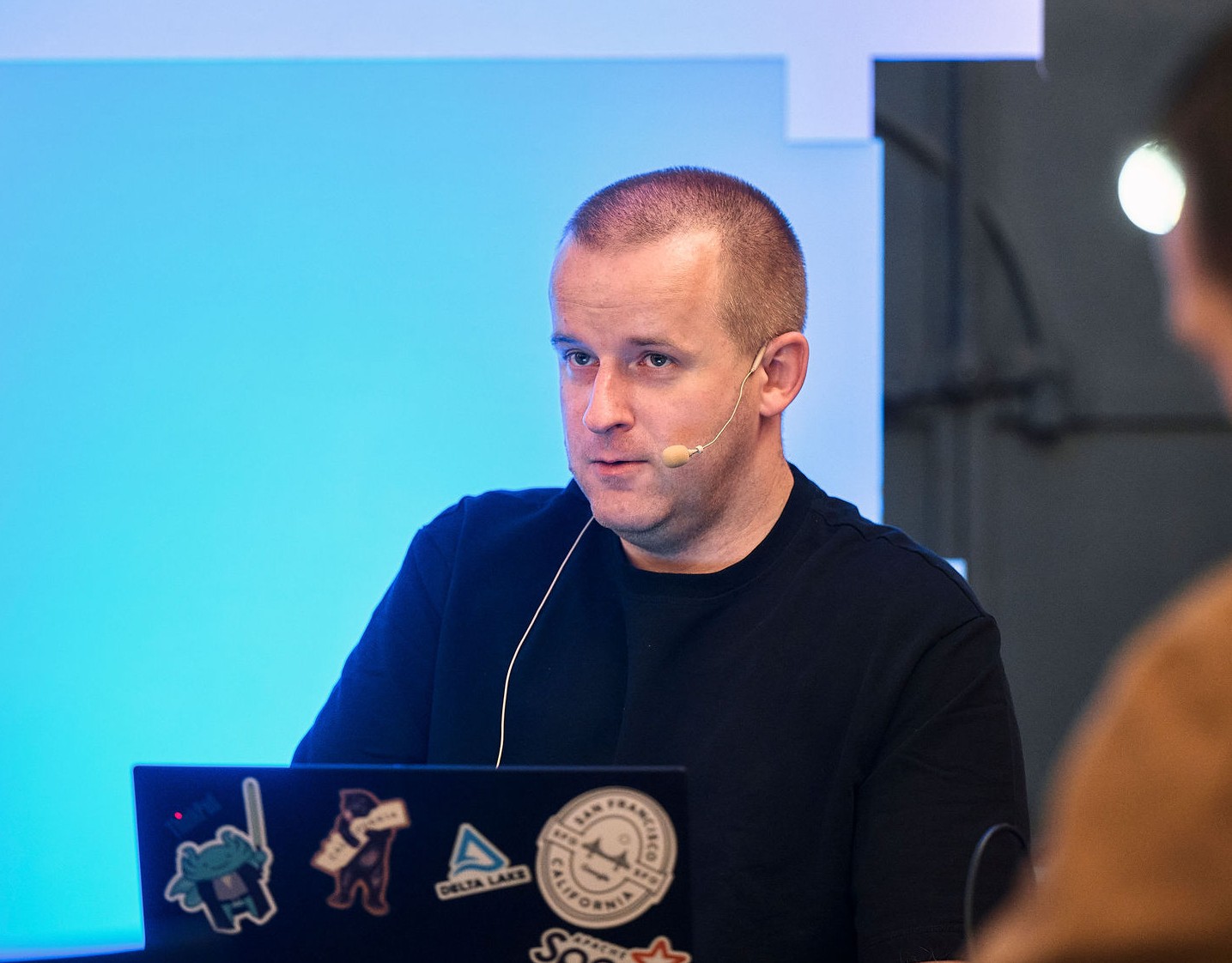
With nearly 16 years of experience, including 8 as data engineer, I offer expert consulting to design and optimize scalable data solutions.
As an O’Reilly author, Data+AI Summit speaker, and blogger, I bring cutting-edge insights to modernize infrastructure, build robust pipelines, and
drive data-driven decision-making. Let's transform your data challenges into opportunities—reach out to elevate your data engineering game today!
👉 contact@waitingforcode.com
đź”— past projects