Compaction is also a feature present in Apache Iceberg. However, it works a little bit differently than for Delta Lake presented last time. Why? Let's see in this new blog post!
Data Engineering Design Patterns
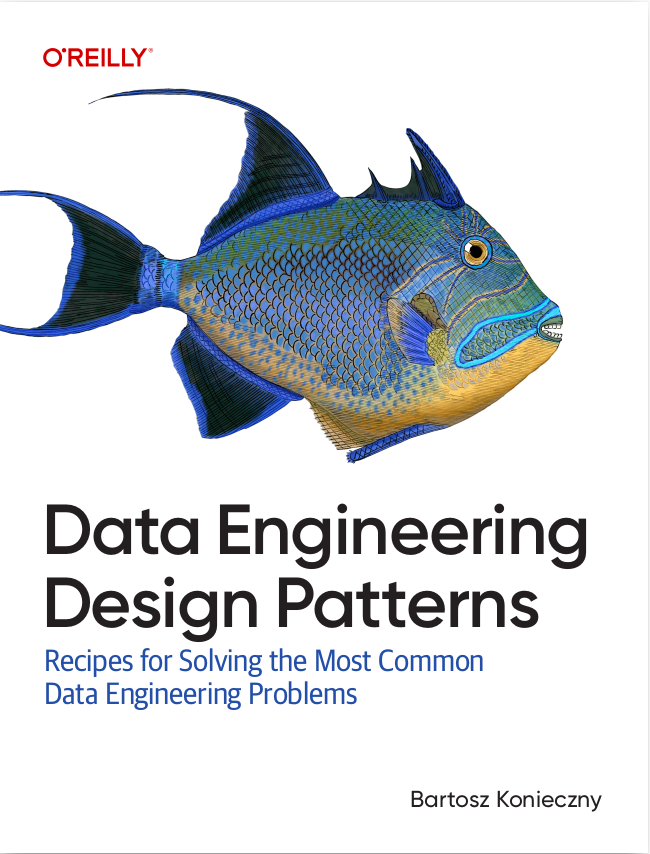
Looking for a book that defines and solves most common data engineering problems? I wrote
one on that topic! You can read it online
on the O'Reilly platform,
or get a print copy on Amazon.
I also help solve your data engineering problems 👉 contact@waitingforcode.com 📩
The general idea of compaction remains the same, though. The goal is to optimize the file storage by rearranging the data. That's the "what". When it comes to the "how", there are some differences and probably the most visible one is the presence of compaction strategies.
Compaction
Before we go into the strategies, let's focus on the big picture of the compaction itself. The operation involves the following steps:
- Planning compaction groups. The operation analyzes the metadata information and tries to put as many rows as possible into a single data file.
- Initializing a commit manager. It's an instance of RewriteDataFilesCommitManager class that will be responsible for committing or aborting the compaction transaction.
- Running the compaction. The compaction can be:
- Partial. If the option partial-progress.enabled is set to true, the compaction process will be able to commit rewrites partially. It allows some rewrite jobs to fail.
- Total. In this configuration there will be a single commit added when all data rewrite jobs succeed.
- For Apache Spark runner, the compaction process is an Apache Spark job processing the files to collocate in the same data file. Depending on the chosen strategy, the jobs may be slightly different from one another.
Bin-packing strategy
Apache Iceberg uses one of 3 strategies to generate compaction groups and execute compaction jobs. The first strategy is called Bin-packing and it relies on the same principle as in the Delta Lake compaction, i.e. reducing the number of data files by putting as much data as possible together. To enable it you can omit the strategy option in the rewriteDataFiles action or specify it explicitly, as in the following snippet:
val lettersTable = Spark3Util.loadIcebergTable(sparkSession, "local.db.letters") SparkActions .get() .rewriteDataFiles(lettersTable) .binPack()
Under-the-hood this call sets an internal strategy field to SparkBinPackStrategy. The strategy uses several options to customize the compaction behavior:
- target-file-size-bytes defines the ideal size of each data file after the compaction.
- min-file-size-bytes defines the minimal size of the files to rewrite (= files smaller than the defined value will be considered; compaction scenario)
- max-file-size-bytes defines the maximal size of the files to write (= files bigger than that value will be rewritten; data split scenario)
- max-file-group-size-bytes defines the maximal size of each group of files to rewrite.
- min-input-files defines the minimal number of files to compact in a group to consider the group as a compaction candidate.
- delete-file-threshold defines the minimal number of delete actions in a file. Files with more deletes than this threshold will be considered for rewriting.
- rewrite-all overrides all configurations and forces overwriting all files, regardless of their size.
What is the bin-packing algorithm? First, in the selectFilesToRewrite(Iterable
return FluentIterable.from(dataFiles) .filter( scanTask -> scanTask.length() < minFileSize || scanTask.length() > maxFileSize || taskHasTooManyDeletes(scanTask));
The selection works per partition. After getting the files, the operation prepares the compaction groups by calling the bin-packing algorithm defined in the planFileGroups method. The grouping puts file candidates into bins with the goal to reduce the number of created bins, therefore to put as much data in a single file as possible. Once groups resolved, the operation continues and either accepts all group candidates (rewrite-all flag set to true), or applies the filtering logic with the group-related options:
public Iterable<List<FileScanTask>> planFileGroups(Iterable<FileScanTask> dataFiles) { // ... return potentialGroups.stream() .filter( group -> (group.size() >= minInputFiles && group.size() > 1) || (sizeOfInputFiles(group) > targetFileSize && group.size() > 1) || sizeOfInputFiles(group) > maxFileSize || group.stream().anyMatch(this::taskHasTooManyDeletes)) .collect(Collectors.toList());
In the end of this preparation stage, tie rewrite action executes the rewriteFiles(List<FileScanTask> filesToRewrite) to physically change the storage layout. Basically, it's a read-write operation using the target and max file size configuration:
protected long writeMaxFileSize() { return (long) (targetFileSize + ((maxFileSize - targetFileSize) * 0.5)); }
Besides, there is also interesting information on how Apache Iceberg relates the files to rewrite groups with the reader. If you check the reading part, you won't notice any mention of the input files. Instead, there is a groupID:
@Override public Set<DataFile> rewriteFiles(List<FileScanTask> filesToRewrite) { String groupID = UUID.randomUUID().toString(); try { tableCache.add(groupID, table); manager.stageTasks(table, groupID, filesToRewrite); // ... Dataset<Row> scanDF = cloneSession .read() .format("iceberg") .option(SparkReadOptions.FILE_SCAN_TASK_SET_ID, groupID) .option(SparkReadOptions.SPLIT_SIZE, splitSize(inputFileSize(filesToRewrite))) .option(SparkReadOptions.FILE_OPEN_COST, "0") .load(groupID);
The rewriting process uses a FileScanTaskSetManager that remembers the group and the contained files. Later, the SparkFilesScan gets the files list and creates a list of data reading tasks:
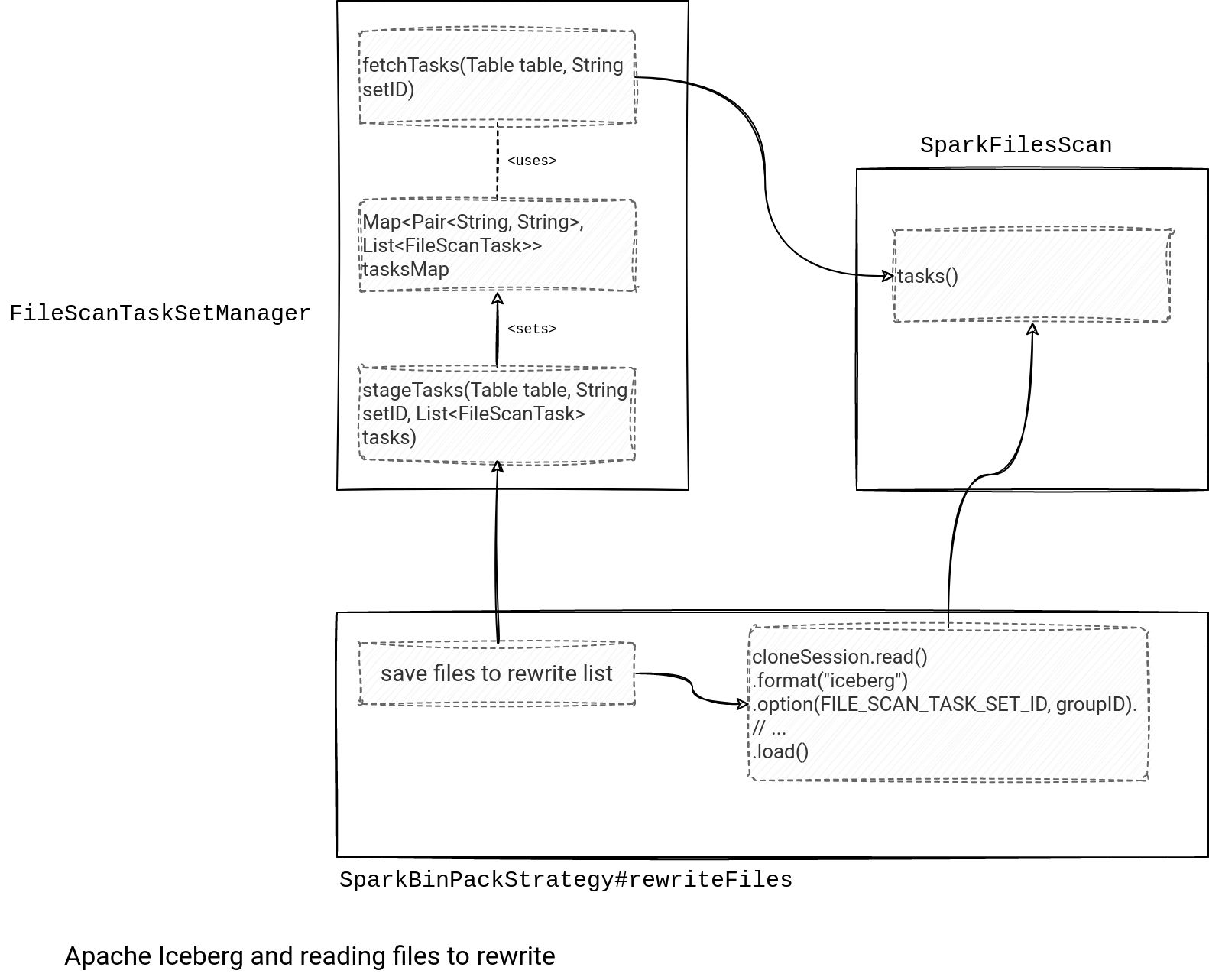
Sort strategy
The second strategy adds an extra sorting step, hence the name sort strategy. However, it's also related to bin-packing. The SortStrategy class extends the BinPackStrategy and it does overwrite nothing but the rewriteFiles method. As a result, the file groups are also created with the bin-packing algorithm and the single difference will be in the content of the compacted files.
The compaction result with sort-based strategy sorts rows inside the compacted data files. It's ensured by an extra sorting step added to the Apache Spark execution plan:
public class SparkSortStrategy extends SortStrategy { // ... public Set<DataFile> rewriteFiles(List<FileScanTask> filesToRewrite) { // ... SortOrder[] ordering; if (requiresRepartition) { // Build in the requirement for Partition Sorting into our sort order ordering = SparkDistributionAndOrderingUtil.convert( SortOrderUtil.buildSortOrder(table, sortOrder())); } else { ordering = SparkDistributionAndOrderingUtil.convert(sortOrder()); } Distribution distribution = Distributions.ordered(ordering); // ... SQLConf sqlConf = cloneSession.sessionState().conf(); LogicalPlan sortPlan = sortPlan(distribution, ordering, scanDF.logicalPlan(), sqlConf); Dataset<Row> sortedDf = new Dataset<>(cloneSession, sortPlan, scanDF.encoder()); // ...
Besides the classical sort-by, Apache Iceberg also supports Z-Order compaction strategy but as for Delta Lake, I'll cover it in another part of the "Table file formats..." series.
Manifests rewriting
The above was true for the data files. But Apache Iceberg also has a cleaning mechanism for the metadata files. The snippet rewrites manifest files that has deleted files:
val lettersTable = Spark3Util.loadIcebergTable(sparkSession, "local.db.letters") SparkActions .get() .rewriteManifests(lettersTable) .rewriteIf(manifestFile => manifestFile.hasDeletedFiles) .execute()
The rewriteIf adds a new predicate to the rewrite action. The whole algorithm looks like:
- Getting the list of ManifestFiles to rewrite. They should match the predicate and the rewritten partition.
- Creating a DataFrame with these manifests.
- Using the commit.manifest.target-size-bytes to compute the ideal manifest file size:
private int targetNumManifests(long totalSizeBytes) { return (int) ((totalSizeBytes + targetManifestSizeBytes - 1) / targetManifestSizeBytes); }
- Applying the repartitioning for the manifests DataFrame for a partitioned or unpartitioned table. The difference consists mainly of the repartitioning method:
public class RewriteManifestsSparkAction extends BaseSnapshotUpdateSparkAction<RewriteManifestsSparkAction> implements RewriteManifests { // ... // partitioned table // ... return df.repartitionByRange(numManifests, partitionColumn) .sortWithinPartitions(partitionColumn) // ... // unpartitioned table // ... return manifestEntryDF .repartition(numManifests) // ...
Compaction is a process that helps, among others, eliminate small files and optimize the reading I/O. Apache Iceberg supports it for data and metadata layers with the fluent-builder API. How does it work for Apache Hudi? Let's see this in the next blog post of the Table file formats series!
Consulting
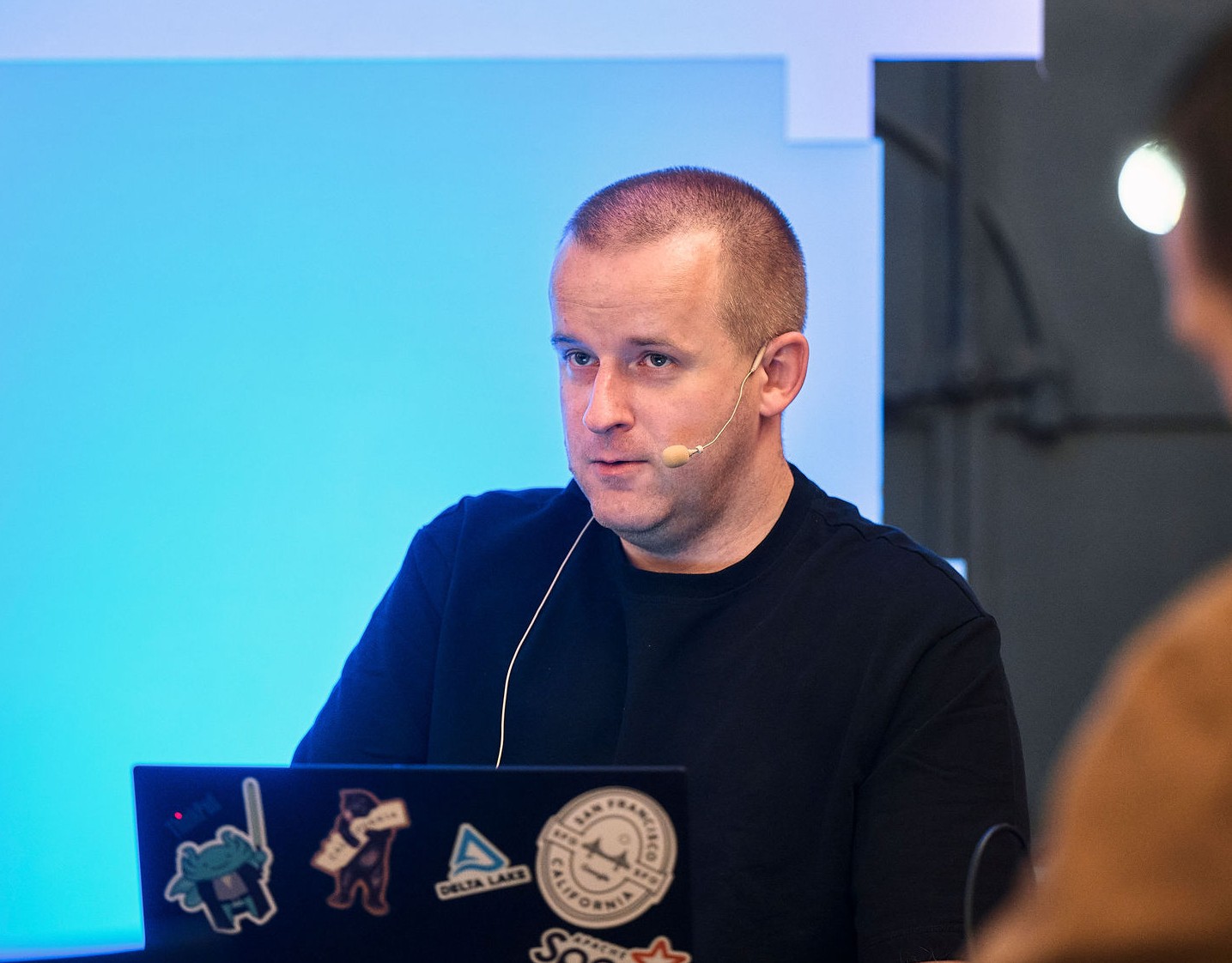
With nearly 16 years of experience, including 8 as data engineer, I offer expert consulting to design and optimize scalable data solutions.
As an O’Reilly author, Data+AI Summit speaker, and blogger, I bring cutting-edge insights to modernize infrastructure, build robust pipelines, and
drive data-driven decision-making. Let's transform your data challenges into opportunities—reach out to elevate your data engineering game today!
👉 contact@waitingforcode.com
đź”— past projects