Spark 2.0 brought some changes at API level. One of them was the merge of DataFrame with Dataset. Thanks to that the 3rd data abstraction, present yet in 1.6, was finally removed.
Data Engineering Design Patterns
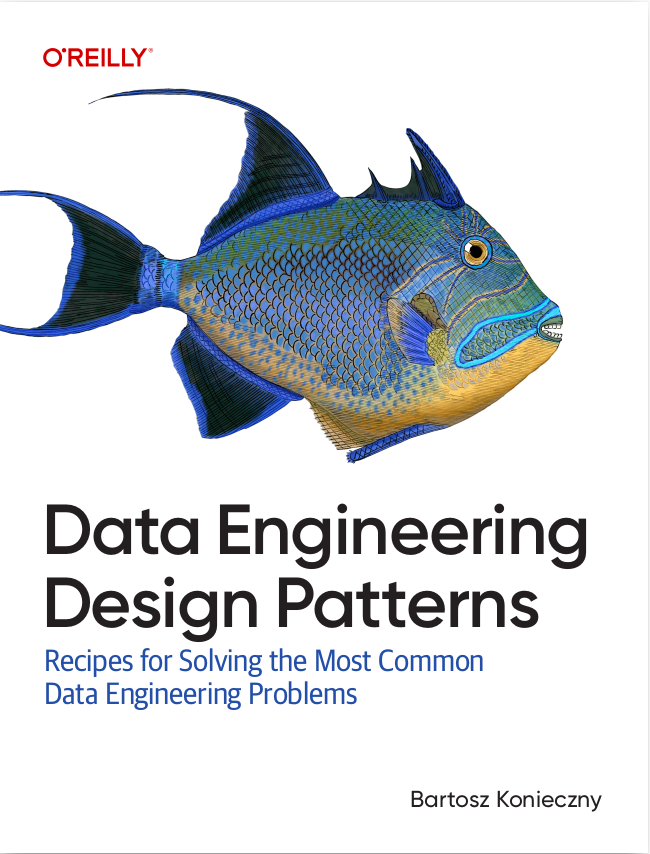
Looking for a book that defines and solves most common data engineering problems? I wrote
one on that topic! You can read it online
on the O'Reilly platform,
or get a print copy on Amazon.
I also help solve your data engineering problems 👉 contact@waitingforcode.com 📩
This post focuses on Dataset. The first part defines this data abstraction used to structured and semi-structured data. The second part shows Dataset evolution and gives an insight about its the relationship to RDD. The last part shows some code examples indicating how to create Dataset from various sources.
Dataset definition
Spark's org.apache.spark.sql.Dataset can be characterized by following points:
- Encoder and QueryExecution tuple - internally Dataset is constructed from a tuple of org.apache.spark.sql.execution.QueryExecution and org.apache.spark.sql.Encoder. This first object defines the workflow of relational query execution. Encoder is responsible for conversion of JVM object to Spark SQL internal representation (row).
- encoders - unlike RDD, Dataset doesn't use neither Java nor Kryo serialization to represent JVM object as a set of bytes. It uses for that the format provided by already quoted Encoder. This format is more concise and allows Spark to perform many operations (filtering, sorting, hashing) without the necessity of deserialization. As shown through many benchmarks (e.g. in Structuring Apache Spark 2.0: SQL, DataFrames, Datasets And Streaming - by Michael Armbrust), it improves Spark's performance.
- QueryExecution - Dataset is internally represented by QueryExecution being a Catalyst logical plan. This plan represents the query that will eventually produce data held in Dataset.
- schema-based - unlike RDD, Dataset is structured, i.e. it has a schema defining data that it can hold.
- strongly-typed - Dataset is strongly-typed. It helps to work with structured and semi-structured data.
- immutable and distributed - as RDD, Dataset can be modified only through transformations constructing new Dataset instances.
- lazy evaluated - similarly to RDD, Dataset is evaluated lazily, i.e. only when an action on it is called.
- optimized - Dataset is tuned by a query optimizer called Catalyst Optimizer (explained in the article about Catalyst Optymizer in Spark).
- uniform and universal - Dataset can handle data coming from various sources, beginning by natively supported relational databases, JSON or HDFS files, and finishing on NoSQL engines as ElasticSearch or Cassandra(theirs connectors are available as third-part plugins).
- RDD interoperatbility - Dataset can be easily converted to RDD thanks to rdd() or toJavaRDD() functions.
Dataset and RDD relationship
Historically Dataset evolved from SchemaRDD. SchemaRDD was the extension of RDD typed to Row object. As its name indicates, it can be thought as a structured RDD (RDD with schema). On analyzing its API (for example in the commit deleting SchemaRDD from Spark) we can easily see that it uses similar concepts that its successors. It's associated with LogicalPlan class, actually being a part of QueryExecution. The same QueryExecution that in its turn composes Dataset.
But meantime Spark 1.3 brought some changes in the API, especially with SPARK-5097. SchemaRDD was then replaced by DataFrame. With redesigned API, DataFrame wasn't anymore coupled to RDD. It was simply an object composed of SQLContext and already mentioned QueryExecution. RDD became lazy evaluated member of DataFrame.
Spark 1.6 introduced Dataset and the next release (2.0) transformed DataFrame to an alias for Dataset<Row>. Dataset, beside some API changes, brought another improvement - org.apache.spark.sql.Encoder that helps to work with Spark's efficient internal format.
Even if Dataset is less coupled to RDDs, it still uses them to execute computations. Physical operations in Spark SQL are represented by org.apache.spark.sql.execution.SparkPlan implementations. And the method responsible for the real execution clearly invokes RDD:
/** * Returns the result of this query as an RDD[InternalRow] by delegating to `doExecute` after * preparations. * * Concrete implementations of SparkPlan should override `doExecute`. */ final def execute(): RDD[InternalRow] = executeQuery { doExecute() }
The use of RDD can also be detected through events listening, configured with spark.eventLog.enabled and spark.eventLog.dir properties. Below tested code and generated output:
@Test public void should_create_dataset_from_objects() throws InterruptedException { City london = valueOf("London", "England", "Europe"); City paris = valueOf("Paris", "France", "Europe"); City warsaw = valueOf("Warsaw", "Poland", "Europe"); City tokio = valueOf("Tokio", "Japan", "Asia"); Encoder<City> cityEncoder = Encoders.bean(City.class); Dataset<City> dataset = SESSION.createDataset(Arrays.asList(london, paris, warsaw, tokio), cityEncoder); assertThat(dataset.count()).isEqualTo(4); }
The output was:
{ "Event":"SparkListenerStageCompleted", "Stage Info":{ "Stage Name":"count at DatasetTest.java:144", "Number of Tasks":1, "RDD Info":[ { "RDD ID":3, "Name":"MapPartitionsRDD", "Scope":"{\"id\":\"3\",\"name\":\"Exchange\"}", "Callsite":"count at DatasetTest.java:144", "Parent IDs":[ 2 ], //... }, { "RDD ID":1, "Name":"MapPartitionsRDD", "Scope":"{\"id\":\"7\",\"name\":\"LocalTableScan\"}", "Callsite":"count at DatasetTest.java:144", "Parent IDs":[ 0 ], //... }, { "RDD ID":2, "Name":"MapPartitionsRDD", "Scope":"{\"id\":\"4\",\"name\":\"WholeStageCodegen\"}", "Callsite":"count at DatasetTest.java:144", "Parent IDs":[ 1 ], //... }, { "RDD ID":0, "Name":"ParallelCollectionRDD", "Scope":"{\"id\":\"7\",\"name\":\"LocalTableScan\"}" // ... } ] //... } }
As shown, the call of Dataset's count() method caused the creation of several RDDs under-the-hood.
Dataset examples
Below some examples of Dataset creation:
private static final SparkSession SESSION = SparkSession.builder() .master("local[1]").appName("Dataset Test").getOrCreate(); @Test public void should_construct_dataset_from_json() { // cities.json is not correctly formatted JSON. It's because // .json(String) reads objects line per line which is shown // in should_construct_bad_dataset_from_correctly_formatted_json test Dataset<Row> cities = readCitiesFromJson(); assertThat(cities.collectAsList()).hasSize(4); } @Test public void should_construct_bad_dataset_from_correctly_formatted_json() { String filePath = getClass().getClassLoader().getResource("dataset/cities_correct_format.json").getPath(); Dataset<Row> cities = SESSION.read().json(filePath); // It's 8 because .json(String) takes 1 row per line, even if the row doesn't // contain defined object (city) assertThat(cities.collectAsList()).hasSize(8); cities.printSchema(); } @Test public void should_create_dataset_from_rdd() { JavaSparkContext closeableContext = new JavaSparkContext(SESSION.sparkContext()); try { List<String> textList = Lists.newArrayList("Txt1", "Txt2"); JavaRDD<Row> textRdd = closeableContext.parallelize(textList) .map((Function<String, Row>) record -> RowFactory.create(record)); StructType schema = DataTypes.createStructType(new StructField[] { DataTypes.createStructField("content", DataTypes.StringType, true) }); Dataset<Row> datasetFromRdd = SESSION.createDataFrame(textRdd, schema); assertThat(datasetFromRdd.count()).isEqualTo(2L); List<Row> rows = datasetFromRdd.collectAsList(); assertThat(rows.get(0).mkString()).isEqualTo(textList.get(0)); assertThat(rows.get(1).mkString()).isEqualTo(textList.get(1)); } finally { closeableContext.close(); } } @Test public void should_fail_on_creating_create_dataset_from_rdd_with_incorrect_schema() { JavaSparkContext closeableContext = new JavaSparkContext(SESSION.sparkContext()); try { List<String> textList = Lists.newArrayList("Txt1", "Txt2"); JavaRDD<Row> textRdd = closeableContext.parallelize(textList) .map((Function<String, Row>) record -> RowFactory.create(record)); StructType schema = DataTypes.createStructType(new StructField[]{ DataTypes.createStructField("content", DataTypes.TimestampType, true) }); Dataset<Row> datasetFromRdd = SESSION.createDataFrame(textRdd, schema); // Trigger action to make Dataset really created assertThatExceptionOfType(Exception.class).isThrownBy(() -> datasetFromRdd.show()) .withMessageContaining("java.lang.String is not a valid external type for schema of timestamp"); } finally { closeableContext.close(); } } @Test public void should_print_dataset_schema() { Dataset<Row> cities = readCitiesFromJson(); cities.printSchema(); // Schema should look like: // root // |-- continent: string (nullable = true) // |-- country: string (nullable = true) // |-- name: string (nullable = true) // Here also it's difficult to test, so check simply if Dataset // was constructed assertThat(cities).isNotNull(); } @Test public void should_get_rdd_from_dataset() { Dataset<Row> cities = readCitiesFromJson(); // Dataset stores collected data as RDD // which is a Dataset's field access in Java from rdd() // method RDD<Row> scalaRdd = cities.rdd(); assertThat(scalaRdd).isNotNull(); String rddDag = scalaRdd.toDebugString(); assertThat(rddDag).contains("FileScanRDD[3]", "MapPartitionsRDD[4]", "MapPartitionsRDD[5]", "MapPartitionsRDD[6]"); // We could also use cities.toJavaRDD() JavaRDD<Row> javaRdd = scalaRdd.toJavaRDD(); List<City> citiesList = javaRdd.map(row -> fromRow(row)).collect(); assertThat(citiesList).hasSize(4); assertThat(citiesList).extracting("name").containsOnly("Paris", "Warsaw", "Rome", "Buenos Aires"); } @Test public void should_create_dataset_from_objects() throws InterruptedException { City london = valueOf("London", "England", "Europe"); City paris = valueOf("Paris", "France", "Europe"); City warsaw = valueOf("Warsaw", "Poland", "Europe"); City tokio = valueOf("Tokio", "Japan", "Asia"); Encoder<City> cityEncoder = Encoders.bean(City.class); Dataset<City> dataset = SESSION.createDataset(Arrays.asList(london, paris, warsaw, tokio), cityEncoder); assertThat(dataset.count()).isEqualTo(4); } @Test public void should_get_dataset_from_database() { InMemoryDatabase.init(); Dataset<Row> dataset = SESSION.read() .format("jdbc") .option("url", InMemoryDatabase.DB_CONNECTION) .option("driver", InMemoryDatabase.DB_DRIVER) .option("dbtable", "city") .option("user", InMemoryDatabase.DB_USER) .option("password", InMemoryDatabase.DB_PASSWORD) .load(); assertThat(dataset.count()).isEqualTo(7); } @Test public void should_show_how_physical_plan_is_planed() { // Datasets are strictly related to Catalyst Optimizer described // in another post Dataset<Row> cities = readCitiesFromJson(); Dataset<Row> europeanCities = cities.filter((Row cityRow) -> cityRow.<String>getAs("continent").equals("Europe")) .orderBy(new Column("name").asc()) .filter((Row cityRow) -> cityRow.<String>getAs("name").length() > 2) .limit(1); europeanCities.explain(); // It's quite impossible to test physical plan explanation // It's the reason why we test the result and give physical plan output as // a comment: // == Physical Plan == // CollectLimit 1 // +- *Filter com.waitingforcode.sql.DatasetTest$$Lambda$10/295937119@69796bd0.call // +- *Sort [name#2 ASC NULLS FIRST], true, 0 // +- Exchange rangepartitioning(name#2 ASC NULLS FIRST, 200) // +- *Filter com.waitingforcode.sql.DatasetTest$$Lambda$9/907858780@69ed5ea2.call // +- *FileScan json [continent#0,country#1,name#2] Batched: false, Format: JSON, Location: // InMemoryFileIndex[file:sandbox/spark/target/test-classes/dataset..., PartitionFilters: // [], PushedFilters: [], ReadSchema: struct<continent:string,country:string,name:string> // As you can see, the physical plan started with file reading, without any // pushdown predicate. After that both filters were applied // together. assertThat(europeanCities.count()).isEqualTo(1L); } @Test public void should_show_logical_and_physical_plan_phases() { Dataset<Row> cities = readCitiesFromJson(); Dataset<Row> europeanCities = cities.filter((Row cityRow) -> cityRow.<String>getAs("continent").equals("Europe")) .orderBy(new Column("name").asc()) .filter((Row cityRow) -> cityRow.<String>getAs("name").length() > 2) .limit(1); boolean withLogicalPlan = true; europeanCities.explain(withLogicalPlan); // As should_show_how_physical_plan_is_planed, it's difficult to test printed output // It's the reason why the output is printed here and the test concerns returned elements // == Parsed Logical Plan == // GlobalLimit 1 // +- LocalLimit 1 // +- TypedFilter com.waitingforcode.sql.DatasetTest$$Lambda$10/295937119@69796bd0, // interface org.apache.spark.sql.Row, [StructField(continent,StringType,true), // StructField(country,StringType,true), StructField(name,StringType,true)], // createexternalrow(continent#0.toString, country#1.toString, name#2.toString, // StructField(continent,StringType,true), StructField(country,StringType,true), // StructField(name,StringType,true)) // +- Sort [name#2 ASC NULLS FIRST], true // +- TypedFilter com.waitingforcode.sql.DatasetTest$$Lambda$9/907858780@69ed5ea2, // interface org.apache.spark.sql.Row, [StructField(continent,StringType,true), // StructField(country,StringType,true), StructField(name,StringType,true)], // createexternalrow(continent#0.toString, country#1.toString, name#2.toString, // StructField(continent,StringType,true), StructField(country,StringType,true), // StructField(name,StringType,true)) // +- Relation[continent#0,country#1,name#2] json // == Analyzed Logical Plan == // continent: string, country: string, name: string // GlobalLimit 1 // +- LocalLimit 1 // +- TypedFilter com.waitingforcode.sql.DatasetTest$$Lambda$10/295937119@69796bd0, // interface org.apache.spark.sql.Row, [StructField(continent,StringType,true), // StructField(country,StringType,true), StructField(name,StringType,true)], // createexternalrow(continent#0.toString, country#1.toString, name#2.toString, // StructField(continent,StringType,true), StructField(country,StringType,true), // StructField(name,StringType,true)) // +- Sort [name#2 ASC NULLS FIRST], true // +- TypedFilter com.waitingforcode.sql.DatasetTest$$Lambda$9/907858780@69ed5ea2, // interface org.apache.spark.sql.Row, [StructField(continent,StringType,true), // StructField(country,StringType,true), StructField(name,StringType,true)], // createexternalrow(continent#0.toString, country#1.toString, name#2.toString, // StructField(continent,StringType,true), StructField(country,StringType,true), // StructField(name,StringType,true)) // +- Relation[continent#0,country#1,name#2] json // Analyzed logical plan is a plan with resolved relations and attributes. // The resolution is made with analyzer. It works only on still unresolved // attributes and relations. Unlike parsed plan, we can see that row attributes // (continent, country, name) are defined and type. // == Optimized Logical Plan == // GlobalLimit 1 // +- LocalLimit 1 // +- TypedFilter com.waitingforcode.sql.DatasetTest$$Lambda$10/295937119@69796bd0, interface org.apache.spark.sql.Row, [StructField(continent,StringType,true), StructField(country,StringType,true), StructField(name,StringType,true)], createexternalrow(continent#0.toString, country#1.toString, name#2.toString, StructField(continent,StringType,true), StructField(country,StringType,true), StructField(name,StringType,true)) // +- Sort [name#2 ASC NULLS FIRST], true // +- TypedFilter com.waitingforcode.sql.DatasetTest$$Lambda$9/907858780@69ed5ea2, interface org.apache.spark.sql.Row, [StructField(continent,StringType,true), StructField(country,StringType,true), StructField(name,StringType,true)], createexternalrow(continent#0.toString, country#1.toString, name#2.toString, StructField(continent,StringType,true), StructField(country,StringType,true), StructField(name,StringType,true)) // +- Relation[continent#0,country#1,name#2] json // Represents optimized logical plan, ie. a logical plan // on which rule-based optimizations were applied // == Physical Plan == // ... // And finally physical plan is the same as in should_show_how_physical_plan_is_planed // It's omitted here for brevity assertThat(europeanCities.count()).isEqualTo(1L); } private Dataset<Row> readCitiesFromJson() { String filePath = getClass().getClassLoader().getResource("dataset/cities.json").getPath(); return SESSION.read().json(filePath); } public static class City implements Serializable { private String name; private String country; private String continent; public String getName() { return name; } public void setName(String name) { this.name = name; } public String getCountry() { return country; } public void setCountry(String country) { this.country = country; } public String getContinent() { return continent; } public void setContinent(String continent) { this.continent = continent; } public static City fromRow(Row row) { City city = new City(); city.name = row.getAs("name"); city.country = row.getAs("country"); city.continent = row.getAs("continent"); return city; } public static City valueOf(String name, String country, String continent) { City city = new City(); city.name = name; city.country = country; city.continent = continent; return city; } }
This post describes Dataset handling structured and semi-structured data in Spark. The first part lists and explains the main characteristics of Dataset object. The second part gives an insight about Dataset evolution from SchemaRDD to its current format. The last part shows how to create Dataset in different ways (relational database, JSON file, plain objects).
Consulting
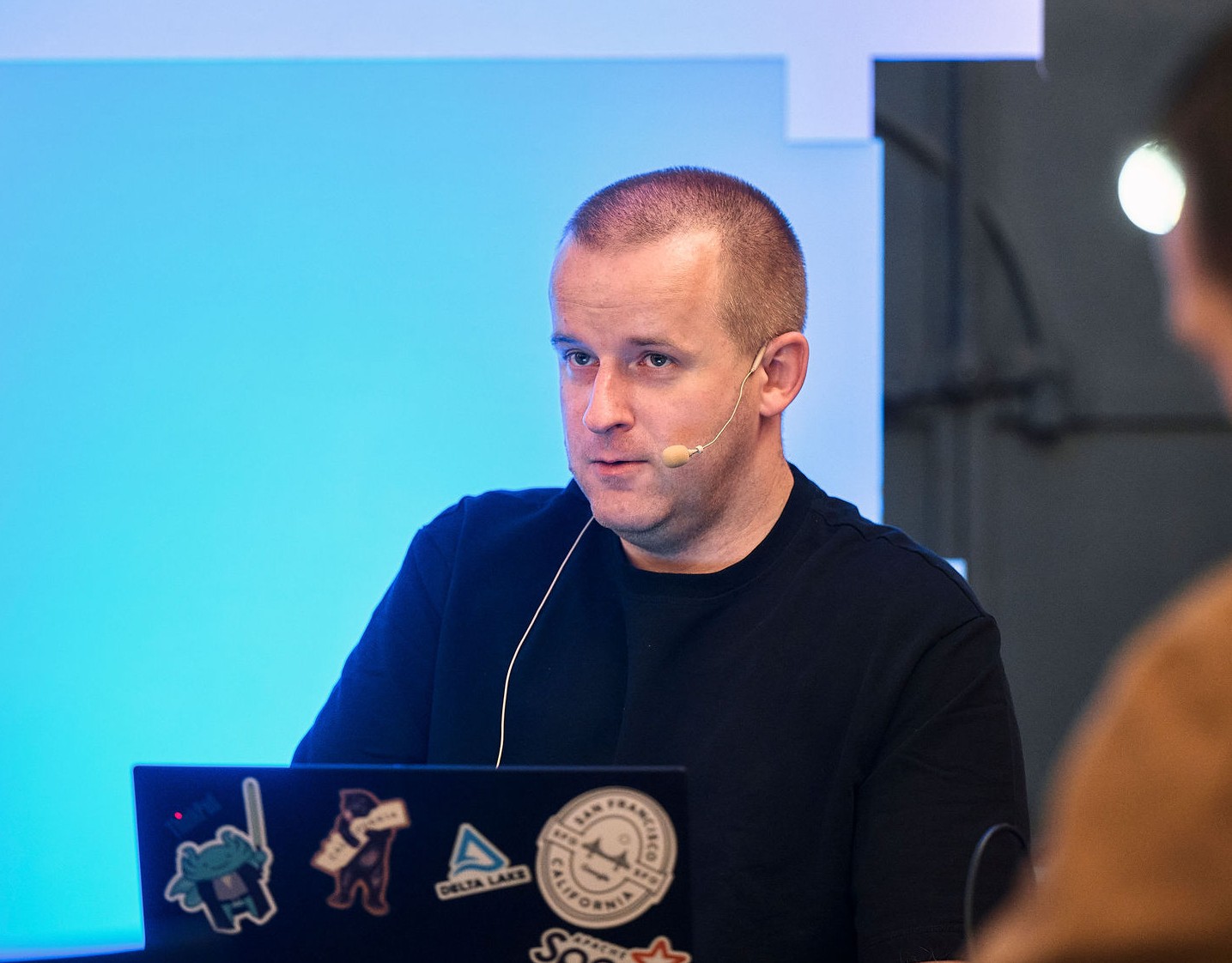
With nearly 16 years of experience, including 8 as data engineer, I offer expert consulting to design and optimize scalable data solutions.
As an O’Reilly author, Data+AI Summit speaker, and blogger, I bring cutting-edge insights to modernize infrastructure, build robust pipelines, and
drive data-driven decision-making. Let's transform your data challenges into opportunities—reach out to elevate your data engineering game today!
👉 contact@waitingforcode.com
đź”— past projects