Every Apache Spark release brings not only completely new components but also new native functions. The 3.1.1 is not an exception and it also comes with some new built-in functions!
Data Engineering Design Patterns
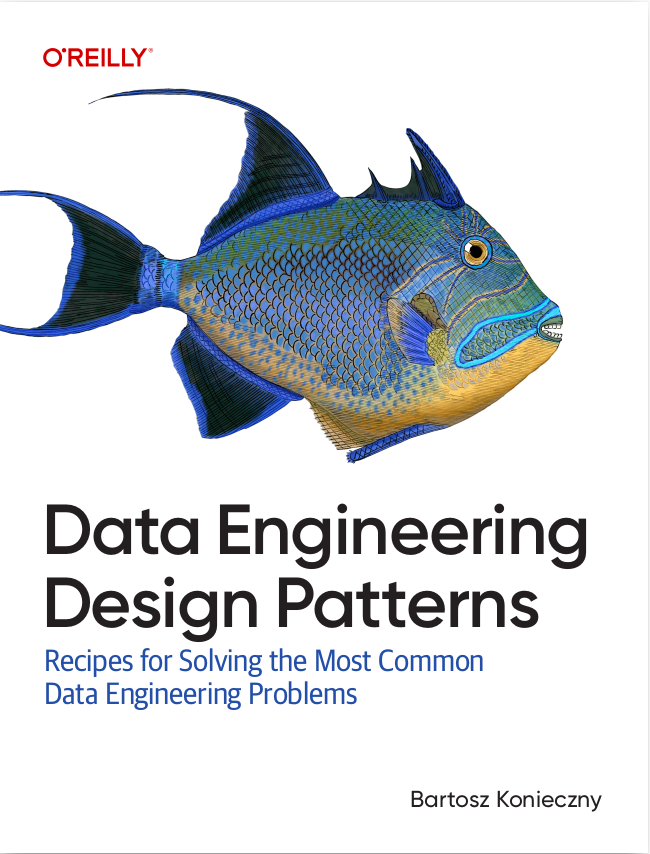
Looking for a book that defines and solves most common data engineering problems? I wrote
one on that topic! You can read it online
on the O'Reilly platform,
or get a print copy on Amazon.
I also help solve your data engineering problems 👉 contact@waitingforcode.com 📩
JSON functions
To start, we've 2 new functions operating on JSON documents. The first of them returns the number of elements in an array. The function is called json_array_length. You can use it alongside the JSON transformation functions to check the properties of the document. The second of the functions is json_object_keys. You can use it to extract the keys of the document. Once again, if mixed with a transformation function, you can analyze the content of nested fields:
Seq( ("""{"number1": 1, "number2": {"number3": 3}, "number4": 4, "number5": [5, 5, 5]}"""), ("""{"number1": 1, "number2": {"number3": 3}, "number4": 4, "number5": [5, 5, 5, 5, 5, 5], "number6": 6}""") ).toDF("json_string").createTempView("json_fields_table") val response = sparkSession.sql( """ |SELECT | JSON_OBJECT_KEYS(json_string) AS outer_fields, | JSON_OBJECT_KEYS(GET_JSON_OBJECT(json_string, "$.number2")) AS nested_keys, | JSON_ARRAY_LENGTH(GET_JSON_OBJECT(json_string, "$.number5")) AS number5_length |FROM json_fields_table |""".stripMargin).as[(Seq[String], Seq[String], Int)].collect() response should have length 2 response should contain allOf( (Seq("number1", "number2", "number4", "number5"), Seq("number3"), 3), (Seq("number1", "number2", "number4", "number5", "number6"), Seq("number3"), 6) )
Both functions were implemented by Rakesh Raushan.
Datetime functions
Much more things happened for datetime functions. To start, a new set of functions were added by philipse, TJX2014 and Max Gekk to convert integer values representing seconds, milliseconds or microseconds, to UNIX timestamps:
val timestampsFromInts = sparkSession.sql( """ |SELECT | TIMESTAMP_SECONDS(1) AS timestamp_seconds_value, | TIMESTAMP_MILLIS(1000) AS timestamp_millis_value, | TIMESTAMP_MICROS(1000000) AS timestamp_micros_value |""".stripMargin).as[(String, String, String)].collect() timestampsFromInts should have size 1 timestampsFromInts(0) shouldEqual ("1970-01-01 01:00:01", "1970-01-01 01:00:01", "1970-01-01 01:00:01")
In addition to them, you can do the opposite: convert a timestamp into seconds, milliseconds, or microseconds. Gengliang Wang implemented the feature and you can find its example just below:
Seq( (Timestamp.valueOf("1970-01-01 01:00:01.000000000")) ).toDF("timestamp_column").createTempView("datetime_functions_table") val integersFromTimestamps = sparkSession.sql( """ |SELECT | UNIX_SECONDS(timestamp_column) AS unix_seconds_value, | UNIX_MILLIS(timestamp_column) AS unix_milliseconds_value, | UNIX_MICROS(timestamp_column) AS unix_microseconds_value |FROM datetime_functions_table |""".stripMargin).as[(Long, Long, Long)].collect() integersFromTimestamps should have size 1 integersFromTimestamps(0) shouldEqual (1L, 1000L, 1000000L)
Gengliang Wang is also the author of 2 other functions, which support conversion between dates and integers. Remember, 01/01/1970 corresponds to 0 days, meaning you will always generate the date for one day later than the integer from the parameter. An example just below where the "30" generates the 31/01/1970:
val dateConversionResults = sparkSession.sql( """ |SELECT | DATE_FROM_UNIX_DATE(0) AS date_from_unix_date_day_0, | DATE_FROM_UNIX_DATE(30) AS date_from_unix_date_day_30, | UNIX_DATE(DATE_FROM_UNIX_DATE(30)) AS unix_date_value_day_30 |""".stripMargin).as[(String, String, String)].collect() dateConversionResults should have size 1 dateConversionResults(0) shouldEqual (("1970-01-01", "1970-01-31", "30"))
Finally, thanks to the work made by ulysses-you you can read the current timezone of your application:
private val sparkSession = SparkSession.builder() .appName("Spark 3.1: new functions").master("local[*]") .config("spark.sql.session.timeZone", "UTC") .getOrCreate() val currentTimezone = sparkSession.sql( """ |SELECT CURRENT_TIMEZONE() |""".stripMargin).as[String].collect() currentTimezone should have size 1 currentTimezone(0) shouldEqual "UTC"
Metadata functions
In the next category of functions Kent Yao added a function returning possibility to get the name of the current catalog backing the read dataset. In the second feature from this category, Yuming Wang and Takeshi Yamamuro added a function computing the number of bucket for an expression:
val bucketsWithCatalog = sparkSession.sql( """ |SELECT | WIDTH_BUCKET(6, 1, 10, 5) AS bucket_for_5_buckets, | WIDTH_BUCKET(6, 1, 10, 2) AS bucket_for_2_buckets, | CURRENT_CATALOG() AS current_catalog |""".stripMargin).as[(BigInt, BigInt, String)].collect() bucketsWithCatalog should have size 1 bucketsWithCatalog(0) shouldEqual((3, 2, "spark_catalog"))
Remaining functions: RegEx, window and errors
To terminate, let's see what happened for "other" functions. To start, you can get any value from a window thanks to the nth_value implemented by Jiaan Geng. One thing to notice, though. The window is a "sliding" window, meaning that if the queried offset was not evaluated yet, you will see a null. Also, you can skip the nulls in your offset evaluation. You can find both these cases presented in the snippet below:
val playersDataset = Seq( ("player1", Some(1), 1), ("player2", Some(1), 1), ("player1", Some(5), 2), ("player2", Some(3), 2), ("player1", Some(11), 3), ("player2", None, 3), ("player1", Some(19), 4), ("player2", Some(7), 4) ).toDF("player_login", "new_points", "timestamp") val playerWindow = Window.partitionBy($"player_login").orderBy($"timestamp".asc) val thirdPointDoesntSkipNulls = functions.nth_value($"new_points", 3, ignoreNulls = false).over(playerWindow) val thirdPointSkipsNulls = functions.nth_value($"new_points", 3, ignoreNulls = true).over(playerWindow) val playersPointIn3rdPosition = playersDataset .select(functions.concat($"player_login", functions.lit("-"), $"timestamp"), thirdPointDoesntSkipNulls.as("point_not_ignore_nulls"), thirdPointSkipsNulls.as("point_ignore_nulls")) .as[(String, Option[Int], Option[Int])] .collect() playersPointIn3rdPosition should have size 8 playersPointIn3rdPosition should contain allOf( ("player1-1", None, None), ("player1-2", None, None), ("player1-3", Some(11), Some(11)), ("player1-4", Some(11), Some(11)), ("player2-1", None, None), ("player2-2", None, None), // As you can see, with ignoreNulls=true, the nth position ignores nulls // and that's why you can see that the 4th row is returned ("player2-3", None, None), ("player2-4", None, Some(7)) )
The second function, added by Jiaan Geng, is regexp_extract_all. Unlike the regexp_extract, it returns all substrings matching the pattern. You can see the difference in the test below:
Seq(("1,2,3 abcdef 4,5,6")) .toDF("sample_text").createTempView("regexp_table") val extractedExpressions = sparkSession.sql( """ |SELECT | REGEXP_EXTRACT_ALL(sample_text, "(\\d+)") AS extraction, | REGEXP_EXTRACT_ALL(sample_text, "(\\d+),(\\d+),(\\d+)", 2) AS extraction_groups, | REGEXP_EXTRACT(sample_text, "(\\d+)") AS extraction_simple, | REGEXP_EXTRACT(sample_text, "(\\d+),(\\d+),(\\d+)", 2) AS extraction_simple_groups |FROM regexp_table |""".stripMargin).as[(Seq[String], Seq[String], String, String)].collect() extractedExpressions should have size 1 extractedExpressions(0) shouldEqual ( Seq("1", "2", "3", "4", "5", "6"), Seq("2", "5"), "1", "2" )
To terminate this category, Karen Feng worked on the assertion and error trigger functions. She added a new function called RAISE_ERROR to fail the processing if some condition is not met. She also extended the ASSERT_TRUE by a possibility to define a custom error message. If the condition evaluates to false, you should see the exception like:
Job aborted due to stage failure: Task 2 in stage 3.0 failed 1 times, most recent failure: Lost task 2.0 in stage 3.0 (TID 8) (192.168.0.55 executor driver): java.lang.RuntimeException: 3 is an odd number at org.apache.spark.sql.catalyst.expressions.GeneratedClass$GeneratedIteratorForCodegenStage1.project_doConsume_0$(generated.java:72) at org.apache.spark.sql.catalyst.expressions.GeneratedClass$GeneratedIteratorForCodegenStage1.processNext(generated.java:108) at org.apache.spark.sql.execution.BufferedRowIterator.hasNext(BufferedRowIterator.java:43) // ...
You can play with this feature on your own here:
// Repartition to show that there are more than 1 tasks Seq((4), (8), (10), (12), (3), (6)).toDF("number1").repartition(6) .createTempView("error_management_table") val exception = intercept[SparkException] { sparkSession.sql( """ |SELECT | ASSERT_TRUE(number1 % 2 == 0, CONCAT(number1, " is an odd number")), | number1 |FROM error_management_table |""".stripMargin).show(false) } exception.getMessage should include("java.lang.RuntimeException: 3 is an odd number")
and here:
// Repartition to show that there are more than 1 task Seq((4), (8), (10), (12), (3), (6)).toDF("number1") .repartition(6).createTempView("error_management_table") val exception = intercept[SparkException] { sparkSession.sql( """ |SELECT | number1, | CASE WHEN number1 % 2 != 0 THEN RAISE_ERROR(CONCAT(number1, " is an odd number")) | ELSE "The number is even" | END |FROM error_management_table |""".stripMargin).show(false) } exception.getMessage should include("java.lang.RuntimeException: 3 is an odd number")
That's all for the new functions in Apache Spark 3.1.1, and at the same time, all for the "What's new in Apache Spark 3.1.1" series. Next week I will resume my work on other Apache Spark features not necessarily related to this specific release.
Consulting
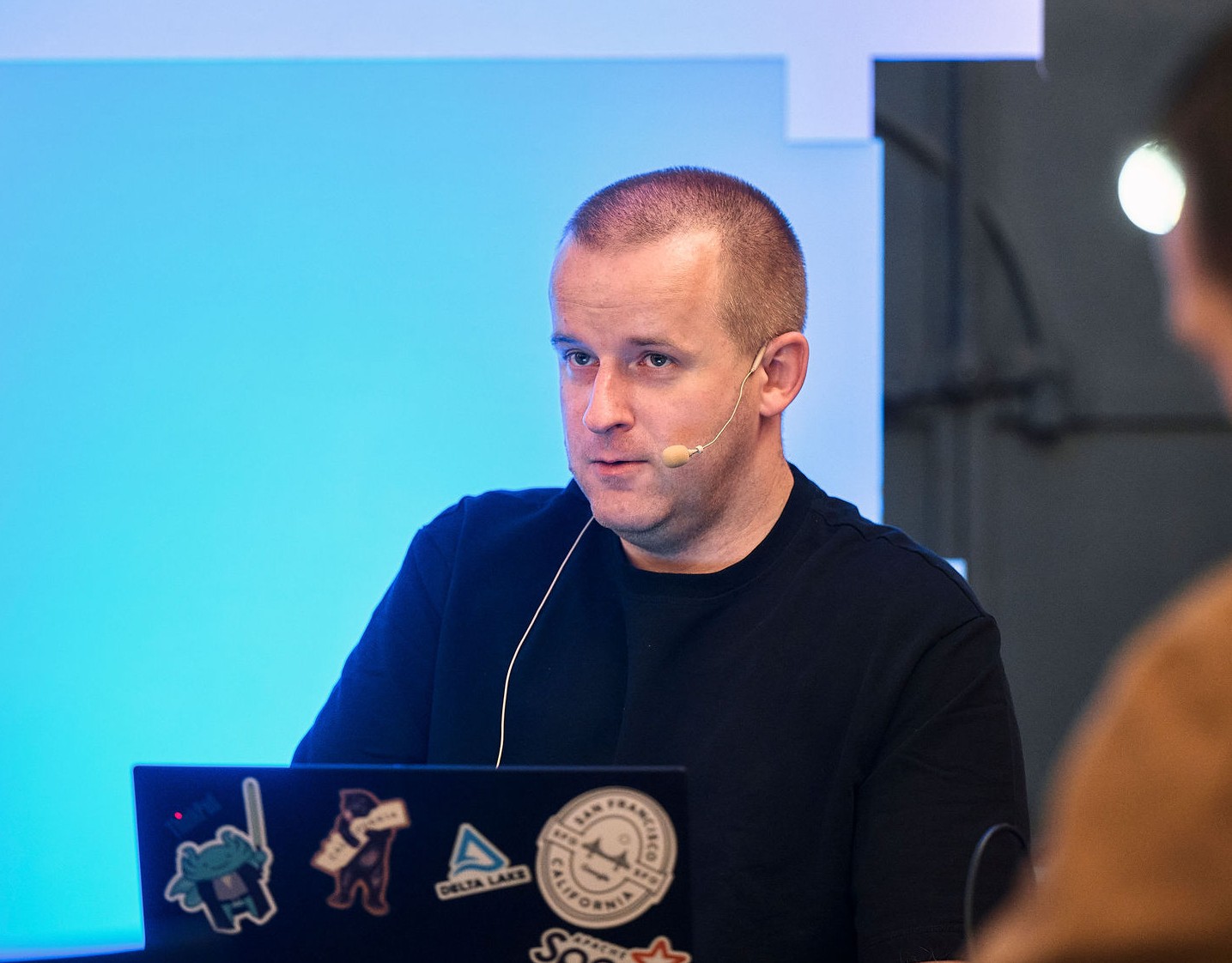
With nearly 16 years of experience, including 8 as data engineer, I offer expert consulting to design and optimize scalable data solutions.
As an O’Reilly author, Data+AI Summit speaker, and blogger, I bring cutting-edge insights to modernize infrastructure, build robust pipelines, and
drive data-driven decision-making. Let's transform your data challenges into opportunities—reach out to elevate your data engineering game today!
👉 contact@waitingforcode.com
đź”— past projects