I've discovered the term from the title while learning Azure Synapse and Cosmos DB services. I had heard of NoSQL, or even NewSQL, but never of a solution supporting analytical and transactional workloads at once.
Data Engineering Design Patterns
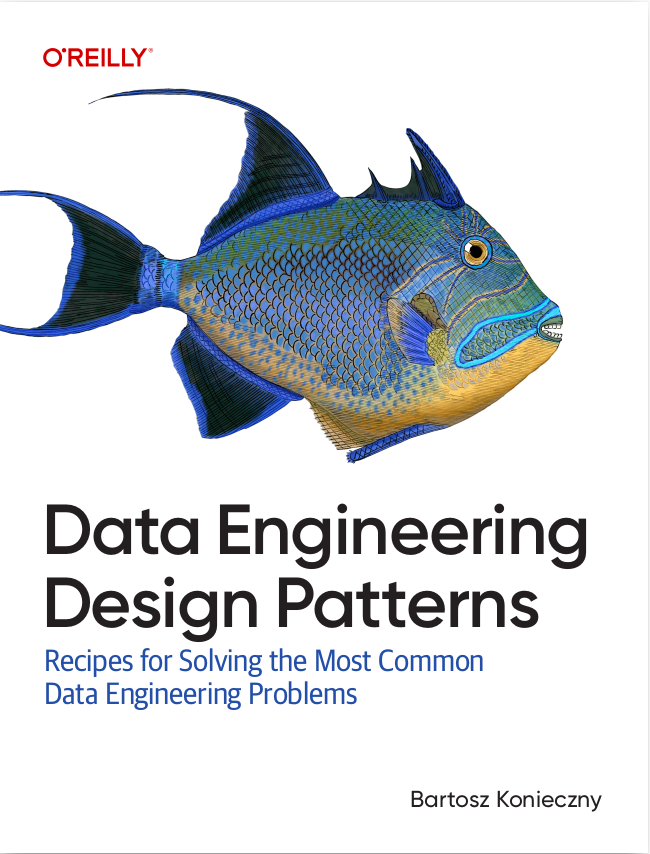
Looking for a book that defines and solves most common data engineering problems? I wrote
one on that topic! You can read it online
on the O'Reilly platform,
or get a print copy on Amazon.
I also help solve your data engineering problems 👉 contact@waitingforcode.com 📩
Hybrid Transactional/Analytical Processing 101
Although it's technically feasible to use transactional data in an analytical data store, it requires some important effort. You have to setup - and by setup I mean write the code and maintain it - a data synchronization Extract Load pipeline. It's not rocket science but it's still an extra item in the data system to take care of. Additionally, the analytical data stores are well known for their performance on handling big volumes of data. So having a job that copies each row written in the transactional data store may not be efficient.
Hybrid Transactional/Analytical Processing (HTAP) automates this manual process by making the data accessible without any prior effort. Put another way, every transactional write is available for analytical use cases without any data synchronization pipeline. It guarantees not only the seamless data exposition for each use case but also lower data latency due to the same storage implementation.
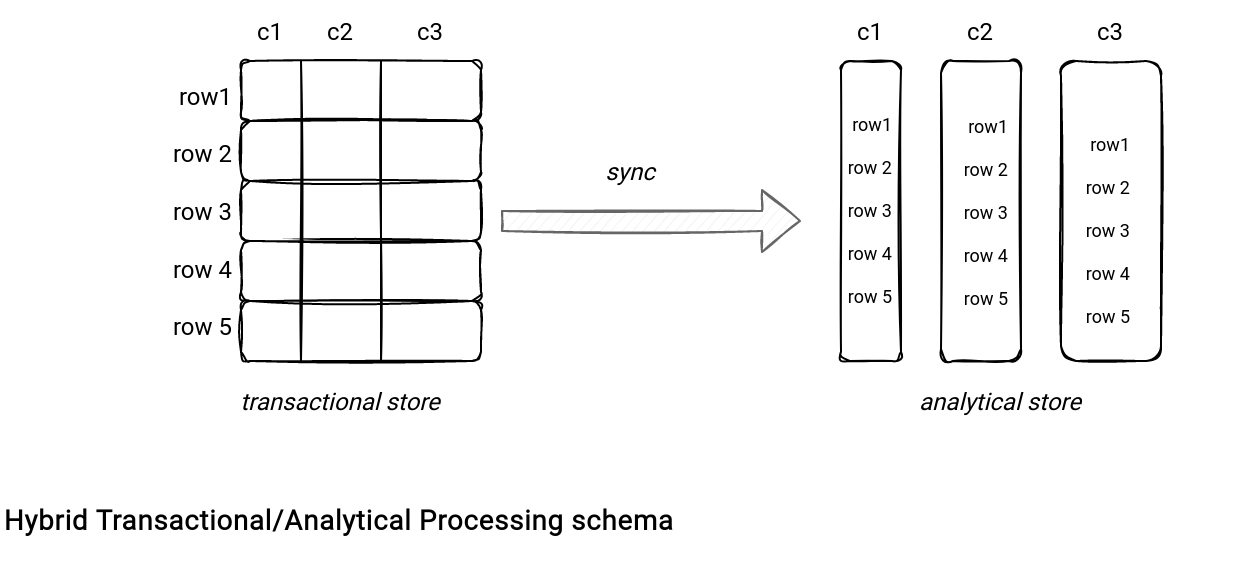
Azure Synapse Link
A great example for the HTAP is Azure Synapse Link. It's the component making transactional data from SQL Database or Cosmos DB queryable from Azure Synapse. How? Let's see the integration points for the integration with Cosmos DB.
- Throughput isolation. The transactional workload isn't impacted by the analytical queries.
- Storage isolation. Transactional and analytical stores can have different Time To Leave (TTL) properties to control how long the records should be retained. It may be a trap because the synchronization happens only in one direction (transactional to analytical). As a result, if the TTL of the analytical storage is bigger (e.g., 2 months vs. 1 week), at the restore process it'll only contain the data available in the transactional store (1 week in our case).
- Partitioning isolation. The partitioning strategy of the transactional and analytical stores is separated. By default, the analytical store isn't partitioned but there is a custom partitioning feature in Preview.
- Schema. Even though Cosmos DB doesn't enforce a unique schema for all documents, its analytical store does to achieve better query performance.
- Schema evolution. Additionally to the schema storage, the analytical data store also performs schema evolution.
- Latency. Synapse Link synchronizes all changes from the transactional to the analytical storage under-the-hood and provides near real-time querying capacity.
- Storage paradigm. Transactional store persists the data as row which is more efficient for the transactional queries. On the other hand, this format is not well suited for analytical queries. That's why the analytical store uses a more adapted columnar format.
Hybrid Transactional/Analytical Processing is not available for all cloud services but the example of Azure Synapse shows how it's interesting compared to the custom EL synchronization jobs!
Consulting
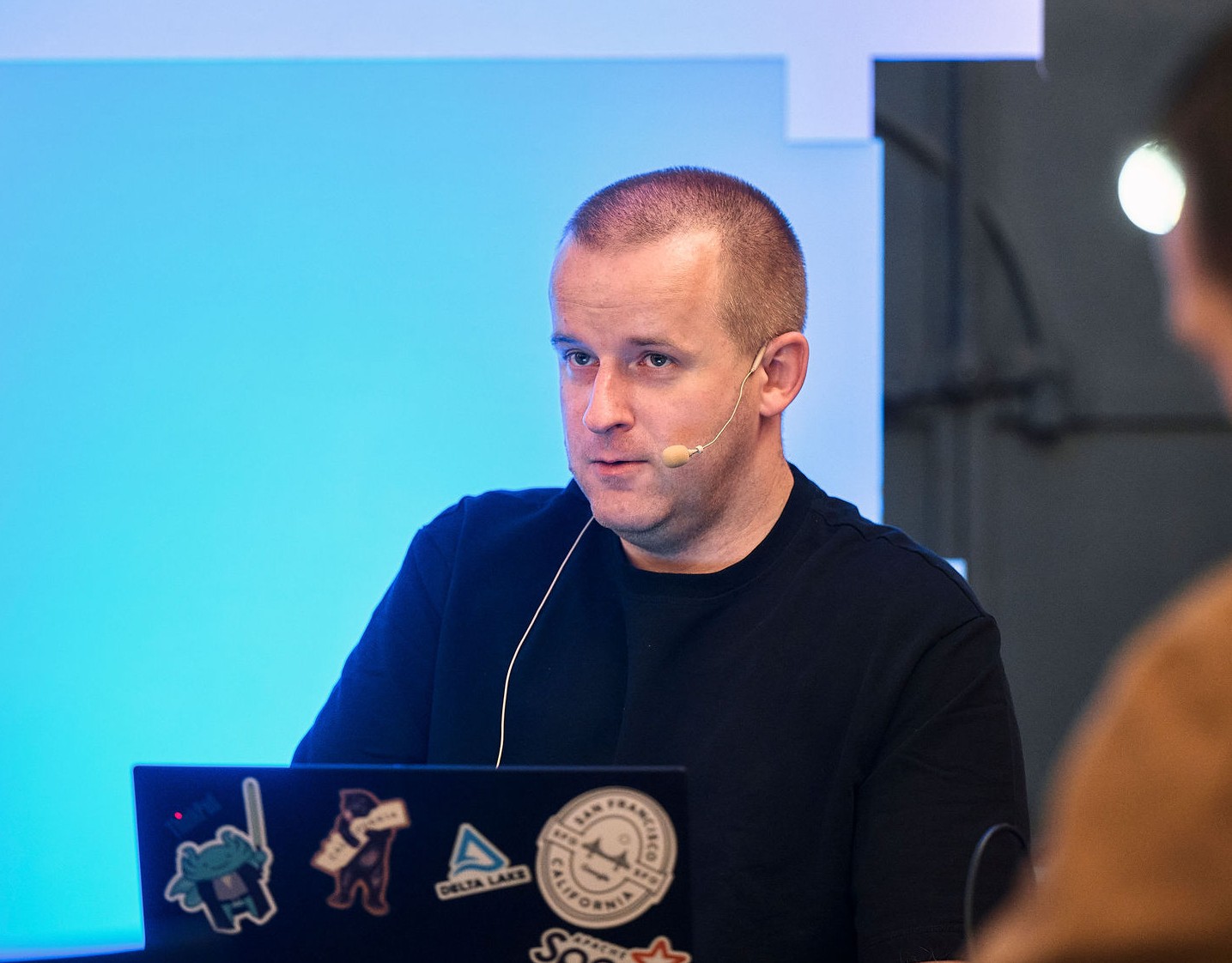
With nearly 16 years of experience, including 8 as data engineer, I offer expert consulting to design and optimize scalable data solutions.
As an O’Reilly author, Data+AI Summit speaker, and blogger, I bring cutting-edge insights to modernize infrastructure, build robust pipelines, and
drive data-driven decision-making. Let's transform your data challenges into opportunities—reach out to elevate your data engineering game today!
👉 contact@waitingforcode.com
đź”— past projects