Have you ever wondered what is the relationship between drop and select operations in Apache Spark SQL? If not, I will shed some light on them in this short blog post.
Data Engineering Design Patterns
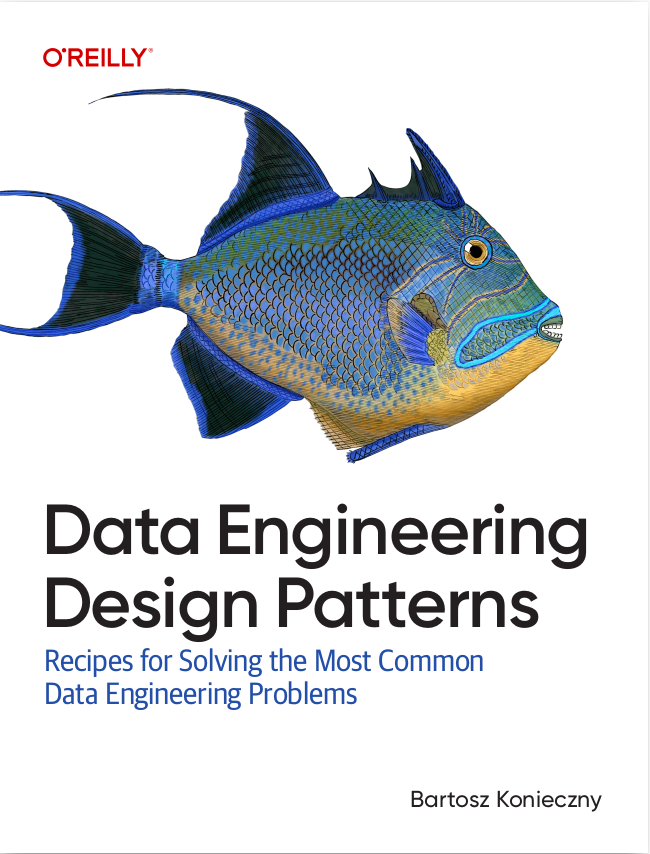
Looking for a book that defines and solves most common data engineering problems? I wrote
one on that topic! You can read it online
on the O'Reilly platform,
or get a print copy on Amazon.
I also help solve your data engineering problems 👉 contact@waitingforcode.com 📩
Let's suppose that I want to reduce the size of the dataset and select only a subset of columns. My initial input looks that way:
private val testSparkSession = SparkSession.builder() .appName("Select vs Drop").master("local[*]") .getOrCreate() import testSparkSession.implicits._ val dataset = Seq( (0, "a", "A"), (1, "b", "B"), (2, "c", "C") ).toDF("nr", "lower_letter", "upper_letter")
To retrieve only lower_letter and upper_letter columns, we can select them explicitly:
dataset.select("lower_letter", "upper_letter").show()
But it's not the single approach. Another one uses drop(colName: String) function that removes the columns from the dataset:
dataset.drop("nr").show()
An interesting thing to notice is how the drop operation works under-the-hood. In fact, it's a select statement that is executed on the columns not matching the columns from the dropped list:
def drop(colNames: String*): DataFrame = { val resolver = sparkSession.sessionState.analyzer.resolver val allColumns = queryExecution.analyzed.output val remainingCols = allColumns.filter { attribute => colNames.forall(n => !resolver(attribute.name, n)) }.map(attribute => Column(attribute)) if (remainingCols.size == allColumns.size) { toDF() } else { this.select(remainingCols: _*) } }
It's even more visible when you compare the logical plans. The first one is for the select-based reduction and the second one for the drop-based one:
# select("lower_letter", "upper_letter") == Analyzed Logical Plan == lower_letter: string, upper_letter: string Project [lower_letter#11, upper_letter#12] +- Project [_1#3 AS nr#10, _2#4 AS lower_letter#11, _3#5 AS upper_letter#12] +- LocalRelation [_1#3, _2#4, _3#5] == Optimized Logical Plan == LocalRelation [lower_letter#11, upper_letter#12] # drop("nr") == Analyzed Logical Plan == lower_letter: string, upper_letter: string Project [lower_letter#11, upper_letter#12] +- Project [_1#3 AS nr#10, _2#4 AS lower_letter#11, _3#5 AS upper_letter#12] +- LocalRelation [_1#3, _2#4, _3#5] == Optimized Logical Plan == LocalRelation [lower_letter#11, upper_letter#12]
And a short video to prove you that I'm not liying ;-):
Drop is then a select :) No need to add it then if you explicitly select some columns and want to remove the not used ones.
Consulting
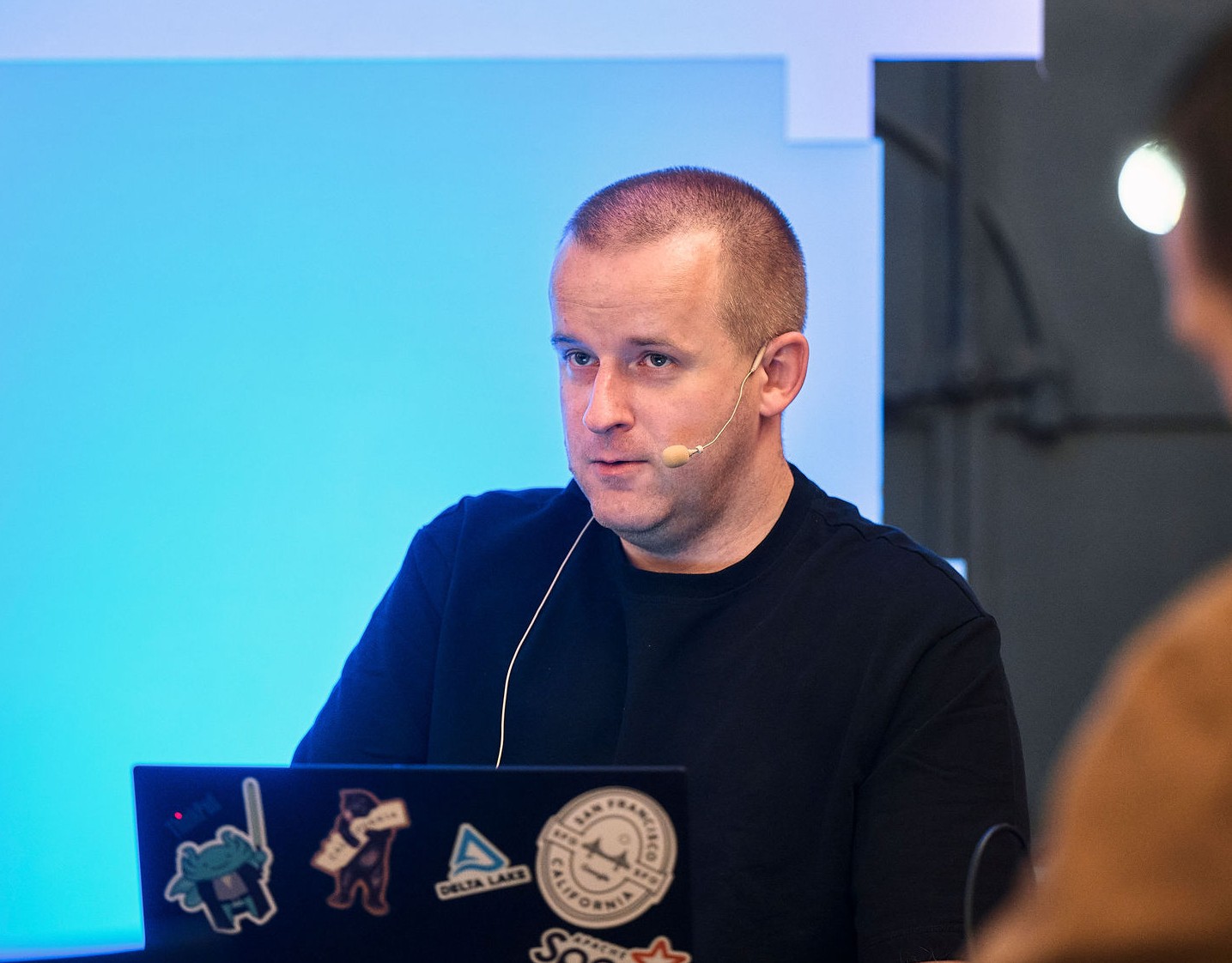
With nearly 16 years of experience, including 8 as data engineer, I offer expert consulting to design and optimize scalable data solutions.
As an O’Reilly author, Data+AI Summit speaker, and blogger, I bring cutting-edge insights to modernize infrastructure, build robust pipelines, and
drive data-driven decision-making. Let's transform your data challenges into opportunities—reach out to elevate your data engineering game today!
👉 contact@waitingforcode.com
đź”— past projects