https://github.com/bartosz25/spark-playground/tree/master/spark-3.3.0-features/sql_functions
New Apache SQL functions are a regular position in my "What's new in Apache Spark..." series. Let's see what has changed in the most recent (3.3.0) release!
Data Engineering Design Patterns
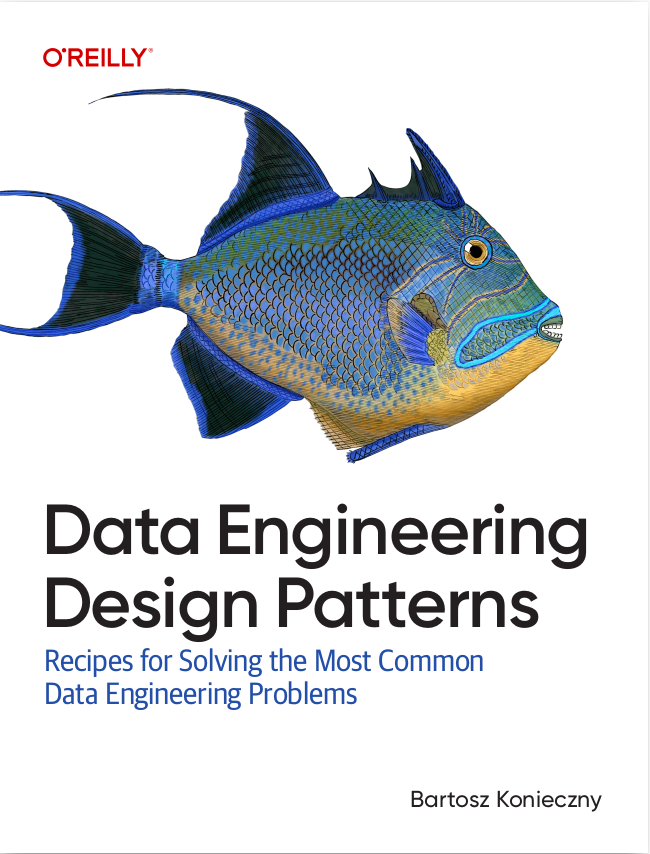
Looking for a book that defines and solves most common data engineering problems? I wrote
one on that topic! You can read it online
on the O'Reilly platform,
or get a print copy on Amazon.
I also help solve your data engineering problems 👉 contact@waitingforcode.com 📩
Date time functions
For starters, let's see the changes for the date time functions:
- TIMESTAMPADD - adds an interval to the timestamp.
- DATEADD - same as above, but adds an interval to a date field. It also returns a timestamp type.
- TIMESTAMPDIFF - returns the difference between 2 timestamps.
- DATEDIFF - same as above, but works for dates.
Credits for the changes above go to Max Gekk.
Additionally, AngersZhuuuu added a support for interval types in PERCENTILE_APPROX, PERCENTILE, and APPROX_COUNT_DISTINCT_FOR_INTERVALS:
You can find some test cases below:
sparkSession.sql( """ |SELECT | TIMESTAMPADD(HOUR, 2, TIMESTAMP '2022-06-01 20:00:00') AS eight_pm_in_2_hours, | DATEADD(HOUR, 45, DATE '2022-06-01 10:00:00') AS date_in_2_days, | DATEADD(HOUR, 48, DATE '2022-06-01 10:00:00') AS date_in_3_days |""".stripMargin) .show(false) // Prints: /* +-------------------+-------------------+-------------------+ |eight_pm_in_2_hours|date_in_2_days |date_in_3_days | +-------------------+-------------------+-------------------+ |2022-06-01 22:00:00|2022-06-02 21:00:00|2022-06-03 00:00:00| +-------------------+-------------------+-------------------+ */ sparkSession.sql( """ |SELECT | TIMESTAMPDIFF(MINUTE, TIMESTAMP '2022-06-01 20:00:00', | TIMESTAMP '2022-06-01 20:40:00') AS tms_diff, | DATEDIFF(MINUTE, DATE '2022-06-01', DATE '2022-06-02') AS date_diff |""".stripMargin) .show(false) // Prints: /* +--------+---------+ |tms_diff|date_diff| +--------+---------+ |40 |1440 | +--------+---------+ */
Error-handling functions
Moreover, Apache Spark 3.3.0 extended the list of error-handling functions that return null in case of an error. The new functions are:
- TRY_SUBTRACT - behaves as an "-" operator but returns null in case of an error.
- TRY_MULTIPLY - is a safe representation of the "*" operator.
- TRY_SUM - is an error-handling implementation of the sum operation.
- TRY_AVG - is an error handling-implementation of the average operation.
- TRY_TO_BINARY - eventually converts an input value to a binary value.
Credits for them go to Gengliang Wang and you will find their examples below:
sparkSession.sql( s""" |SELECT |${Long.MaxValue} * 2 AS not_safe_multiply, |TRY_MULTIPLY(${Long.MaxValue}, 2) AS long_safe_multiply |""".stripMargin) .show(false) // Prints /* +-----------------+------------------+ |not_safe_multiply|long_safe_multiply| +-----------------+------------------+ |-2 |null | +-----------------+------------------+ */ sparkSession.sql( s""" |SELECT |TRY_SUM(data), |SUM(data) |FROM VALUES (${Long.MaxValue}), (${Long.MaxValue}) AS test_table(data) |""".stripMargin) .show(false) // Prints /* +-------------+---------+ |try_sum(data)|sum(data)| +-------------+---------+ |null |-2 | +-------------+---------+ */
Complex types
In the most recent release Apache Spark also got 2 new functions to handle complex types. The ARRAY_SIZE, added by Xinrong Meng, returns the length of an array. Another function, the MAP_CONTAINS_KEY implemented by Gengliang Wang, returns true if the map has a specific key:
sparkSession.sql( """ |SELECT | ARRAY_SIZE(ARRAY(1, 2, 3, 4, 5)) AS array_length, | MAP_CONTAINS_KEY(MAP('a', 'AA', 'b', 'BB'), 'b') AS b_key_in_map |""".stripMargin).show(false) // Prints /* +------------+------------+ |array_length|b_key_in_map| +------------+------------+ |5 |true | +------------+------------+ */
String functions
Several interesting functions are also available for strings. Max Gekk added CONTAINS(), STARTSWITH(), ENDSWITH() and ILIKE() to facilitate finding/matching a substring. The most mysterious from them is ILIKE which is a case-insensitive like that you can see in action just below:
sparkSession.sql( """ |SELECT | CONTAINS('defabcxyz', 'abc') AS contains_abc, | STARTSWITH('abcdefxyz', 'abc') AS startswith_abc, | ENDSWITH('defxyzabc', 'abc') AS endswith_abc, | 'defxyzABC' LIKE '%abc' AS case_sensitive_like, | 'defxyzABC' ILIKE '%abc' AS case_insensitive_like |""".stripMargin).show(false) // Prints /* +------------+--------------+------------+-------------------+---------------------+ |contains_abc|startswith_abc|endswith_abc|case_sensitive_like|case_insensitive_like| +------------+--------------+------------+-------------------+---------------------+ |true |true |true |false |true | +------------+--------------+------------+-------------------+---------------------+ */
ANSI aggregations
Three new aggregation functions are also available thanks to the Jiaan Geng contribution:
- PERCENTILE_DISC and PERCENTILE_CONT are 2 window functions. The "disc" returns a discrete percentile, so the first value in the set whose ordered position is the same or more than the specified fraction. The first parameter of the function is the percentile you want to find. Later the WITHIN GROUP defines the values ordering column. On the other hand, the "cont" returns a percentile for a continuous distribution:
sparkSession.sql( """ |SELECT | PERCENTILE_DISC(0.5) WITHIN GROUP (ORDER BY col) AS disc0_5, | PERCENTILE_DISC(0.25) WITHIN GROUP (ORDER BY col) AS disc0_75, | PERCENTILE_CONT(0.5) WITHIN GROUP (ORDER BY col) AS cont0_75, | PERCENTILE_CONT(0.25) WITHIN GROUP (ORDER BY col) AS cont0_75 | FROM VALUES (10), (20), (30) AS tab(col) |""".stripMargin).show(false) // Prints /* +-------+--------+--------+--------+ |disc0_5|disc0_75|cont0_75|cont0_75| +-------+--------+--------+--------+ |20.0 |10.0 |20.0 |15.0 | +-------+--------+--------+--------+ */
- ARRAY_AGG - applies an aggregation function on a column and returns an array:
sparkSession.sql( """ |SELECT | ARRAY_AGG(DISTINCT col) AS distinct_numbers | FROM VALUES (1), (2), (2), (3) AS tab(col) |""".stripMargin).show(false) // Prints /* +----------------+ |distinct_numbers| +----------------+ |[1, 2, 3] | +----------------+ */
In addition to this contribution, Jiaan Geng added functions for regression analysis. In "official" terms, it's a statistical method to calculate the relationship between an independent variable and a dependent variable with a linear regression equation. In simpler terms, these functions are helpful to find trends in the data. For example, the REGR_AVGY calculates the average of the dependent variable as sum(Y)/N. There are also less simpler functions in the group, like REGR_COUNT(X, Y) that returns the number of rows where X and Y aren't null:
sparkSession.sql( """ |SELECT | REGR_COUNT(x, y), | REGR_AVGY(x, y) | FROM VALUES (10, NULL), (20, 200), (NULL, 300), (40, 400) AS tab(x, y) |""".stripMargin).show(false) // Prints /* +----------------+---------------+ |regr_count(x, y)|regr_avgy(x, y)| +----------------+---------------+ |2 |30.0 | +----------------+---------------+ */
Misc
Let me finish with the other functions changes:
- zhengruifeng added the max_by and min_by functions to the sql.functions package. Previously, they were only available in SQL.
- Xinrong Meng added TO_BINARY function to support conversion from string to binary formats.
- Kousuke Saruta worked on AES_ENCRYPT and AES_DECRYPT functions to, respectively, encrypt and decrypt a value. The basic signature requires the value and passphrase of 16, 24 or 32 bytes:
sparkSession.sql( """ |SELECT | AES_ENCRYPT('a b c d e', 'passphrase_12345') AS encrypted, | CAST(AES_DECRYPT(AES_ENCRYPT('a b c d e', 'passphrase_12345'), 'passphrase_12345') AS STRING) AS decrypted |""".stripMargin).show(true) // Prints /* +--------------------+---------+ | encrypted|decrypted| +--------------------+---------+ |[39 DC 4C A5 08 6...|a b c d e| +--------------------+---------+ */
- Sathiya Kumar added a scale parameter to the FLOOR and CEIL functions:
sparkSession.sql( """ |SELECT | CEIL(3.53221, 3), | FLOOR(3.53221, 3) |""".stripMargin).show(false) // Prints /* +----------------+-----------------+ |ceil(3.53221, 3)|floor(3.53221, 3)| +----------------+-----------------+ |3.533 |3.532 | +----------------+-----------------+ */
- Yuto Akutsu added new functions to calculate secant and cosecant. In addition, he also brought the cotangent support to the sql.functions package
Thanks to the recent effort, Apache Spark extended its support for SQL-based processing and compatibility with the SQL standards. Next week we'll see what changed in the DataSource V2 API!
Consulting
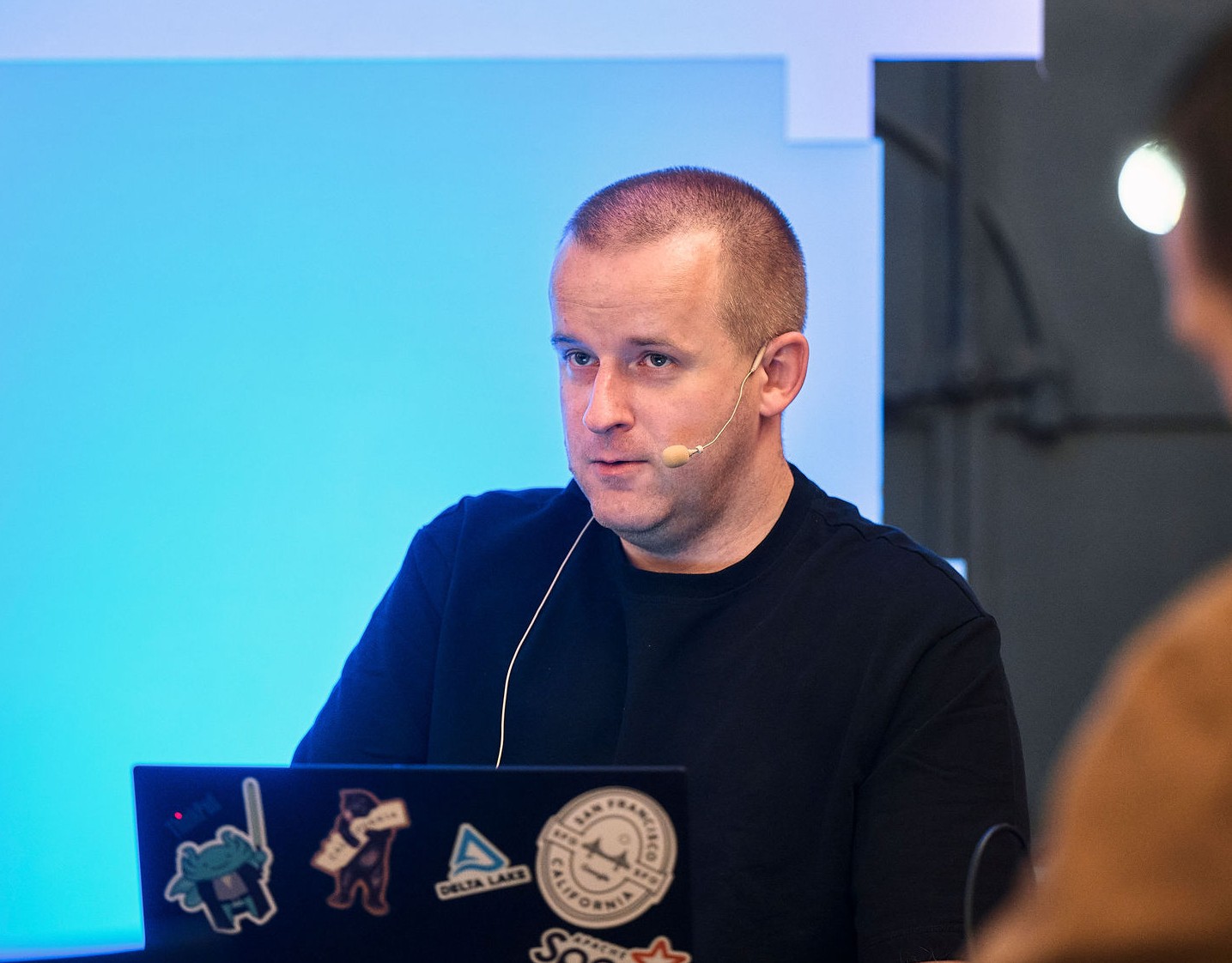
With nearly 16 years of experience, including 8 as data engineer, I offer expert consulting to design and optimize scalable data solutions.
As an O’Reilly author, Data+AI Summit speaker, and blogger, I bring cutting-edge insights to modernize infrastructure, build robust pipelines, and
drive data-driven decision-making. Let's transform your data challenges into opportunities—reach out to elevate your data engineering game today!
👉 contact@waitingforcode.com
đź”— past projects