After a break for the Data+AI Summit retrospective, it's time to return to Apache Spark 3.3.0 and see what changed for the DataSource V2 API.
Data Engineering Design Patterns
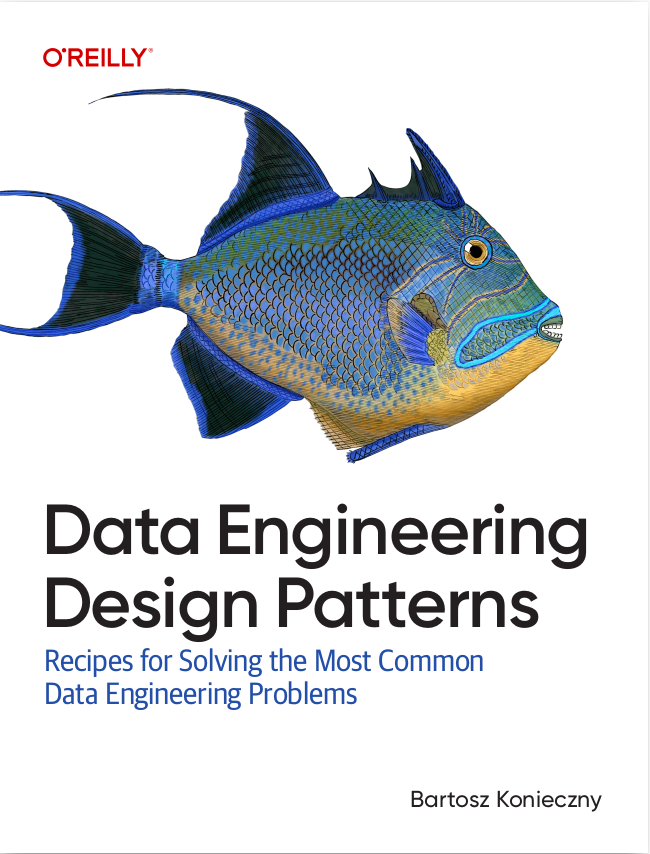
Looking for a book that defines and solves most common data engineering problems? I wrote
one on that topic! You can read it online
on the O'Reilly platform,
or get a print copy on Amazon.
I also help solve your data engineering problems 👉 contact@waitingforcode.com 📩
Index support
In the first feature, Huaxin Gao added a new interface to support the index management. The interface is called SupportsIndex defines the following methods:
public interface SupportsIndex extends Table { void createIndex(String indexName, NamedReference[] columns, Map<NamedReference, Map<String, String>> columnsProperties, Map<String, String> properties) throws IndexAlreadyExistsException; void dropIndex(String indexName) throws NoSuchIndexException; boolean indexExists(String indexName); TableIndex[] listIndexes(); }
The addition of this interface also brings 2 new SQL statements, CREATE INDEX and DROP INDEX, backed respectively by the logical and physical nodes called, CreateIndex/CreateIndexExec and DropIndex/DropIndexExec.
Although the SupportsIndex comes from an Apache Iceberg-related need, it's also implemented natively in Apache Spark JDBCTable data source. And the route is open to add it for any other indexable data source.
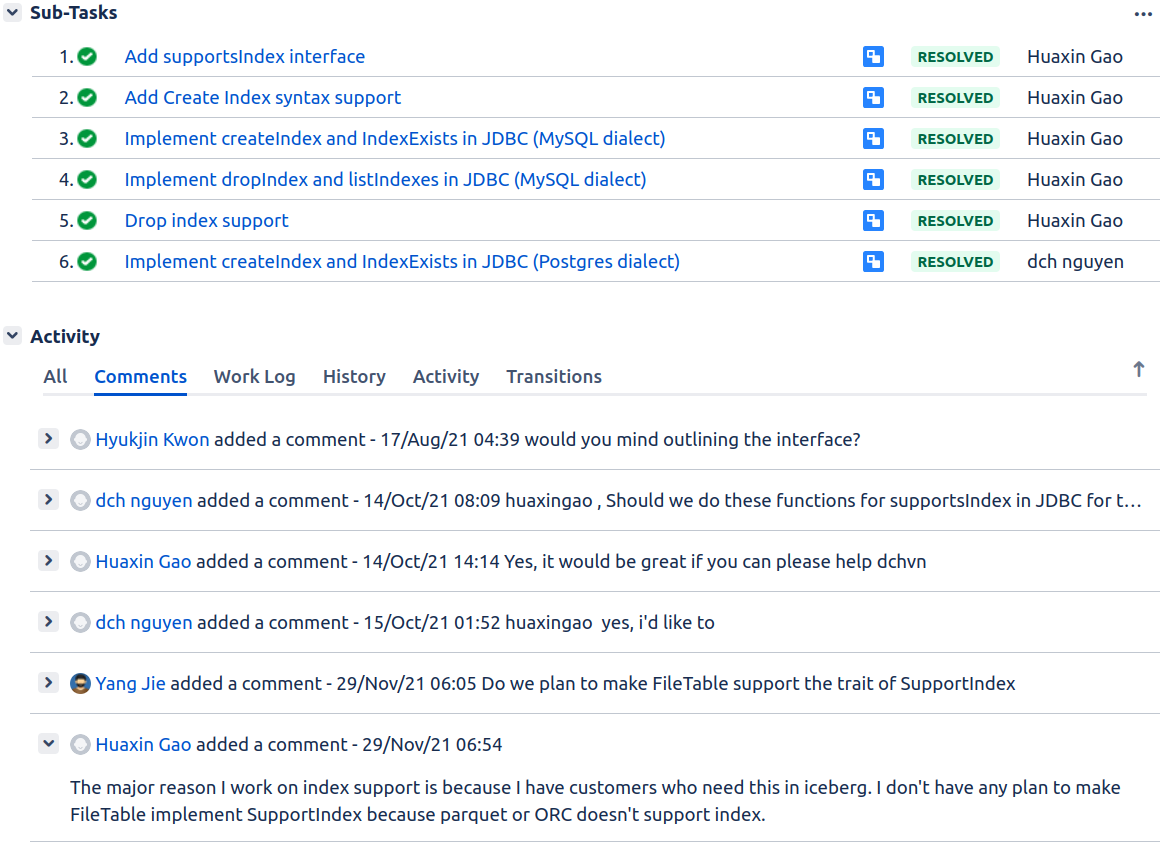
Predicate pushdown support
The second important feature that might not be visible to you as an end-user is the pushdown framework refactoring by Jiaan Geng. The improvement process is still ongoing, but its major part is already in Apache Spark 3.3.0.
Why did this change even happen? Jiaan Geng identified multiple drawbacks in the previous pushdown implementation that can be summarized in 2 major categories:
- Limited support to the filter and aggregate operations. The pushdown is also valid for other scenarios, like a filter or select with SQL functions.
- Correctness for partial pushdown operation. The semantic was incorrect for aggregates without functions or top-n aggregation of a data source with multiple partitions.
Apache Spark manages pushdowns in a logical rule called V2ScanRelationPushDown. It applies to sample, filter, aggregation, limit and scan (data reading) operations. The optimization iteratively optimizes the initial logical plan by adding push downs, starting by transforming the DataSourceV2Relation into a ScanBuilderHolder node:
case class ScanBuilderHolder(output: Seq[AttributeReference], relation: DataSourceV2Relation, builder: ScanBuilder) extends LeafNode { var pushedLimit: Option[Int] = None var sortOrders: Seq[V2SortOrder] = Seq.empty[V2SortOrder] var pushedSample: Option[TableSampleInfo] = None var pushedPredicates: Seq[Predicate] = Seq.empty[Predicate] }
After this initial conversion, the optimization rule updates the ScanBuilderHolder variables. For example, it might add a pushed down predicates:
private def pushDownFilters(plan: LogicalPlan) = plan.transform { case Filter(condition, sHolder: ScanBuilderHolder) => // ... val (pushedFilters, postScanFiltersWithoutSubquery) = PushDownUtils.pushFilters( sHolder.builder, normalizedFiltersWithoutSubquery) val pushedFiltersStr = if (pushedFilters.isLeft) { pushedFilters.left.get.mkString(", ") } else { sHolder.pushedPredicates = pushedFilters.right.get pushedFilters.right.get.mkString(", ") }
Similar rules exist for other pushable components and their detection relies on the SupportsPushDown* interfaces. Below you can find an example of JDBC reader:
case class JDBCScanBuilder( session: SparkSession, schema: StructType, jdbcOptions: JDBCOptions) extends ScanBuilder with SupportsPushDownV2Filters with SupportsPushDownRequiredColumns with SupportsPushDownAggregates with SupportsPushDownLimit with SupportsPushDownTableSample with SupportsPushDownTopN with Logging {
The pushdown changes added in Apache Spark 3.3.0 target mainly the JDBC data source. They consist of getting the JDBC dialect and calling V2ExpressionSQLBuilder to convert Apache Spark Expression into the SQL expression which eventually contains the pushed down element. Among the concrete changes added in Apache Spark 3.3.0 you'll find:
- A new isSupportedFunction(funcName: String) method in the JdbcDialect class that is implemented by each RDBMS dialect supported in Apache Spark (e.g. H2Dialect for the in-memory database, PostgresDialect for PostgreSQL). The function returns true if the function from the parameter is supported by the database and therefore, can be pushed down. Currently, all dialects but H2Dialect don't override it and return false:
private object H2Dialect extends JdbcDialect { private val supportedFunctions = Set("ABS", "COALESCE", "LN", "EXP", "POWER", "SQRT", "FLOOR", "CEIL") override def isSupportedFunction(funcName: String): Boolean = supportedFunctions.contains(funcName)
- The V2ExpressionSQLBuilder#build(Expression expr) method using the isSupportedFunction. Its main responsibility is to convert a single logical expression into a corresponding pushable function. Below you will find an example from JDBCRDD pushed filters:
private[jdbc] class JDBCRDD // ... private val filterWhereClause: String = { val dialect = JdbcDialects.get(url) predicates.flatMap(dialect.compileExpression(_)).map(p => s"($p)").mkString(" AND ") } abstract class JdbcDialect extends Serializable with Logging{ // ... def compileExpression(expr: Expression): Option[String] = { val jdbcSQLBuilder = new JDBCSQLBuilder() try { Some(jdbcSQLBuilder.build(expr)) // ... private[jdbc] class JDBCSQLBuilder extends V2ExpressionSQLBuilder { // ... // #build is not overriden but some functions are, like the visitSQLFunction override def visitSQLFunction(funcName: String, inputs: Array[String]): String = { if (isSupportedFunction(funcName)) { s"""$funcName(${inputs.mkString(", ")})""" } else { // The framework will catch the error and give up the push-down. // Please see `JdbcDialect.compileExpression(expr: Expression)` for more details. throw new UnsupportedOperationException( s"${this.getClass.getSimpleName} does not support function: $funcName") } }
Row-level operations
To finish this blog post, let's introduce the row-level operations support added by Anton Okolnychyi! The SPIP document details the planned work and identifies 2 different types of the row-level operations that should be supported in Apache Spark:
- Group-based, where a whole group of partitions or files must be rewritten.
- Element-based, where only a small part of rows, not necessarily all living on the same physical storage, must be updated.
Additionally, the sources may need different input semantics related to:
- Tracking. It defines how the source identifies the rows to manipulate. The process can use a technical or data row id.
- Granularity. The source may or may not support real in-place changes. For example, a JDBC data source can directly update/delete a single record without worrying about its physical storage. It's not the case with Parquet files, where each in-place operation requires rewriting the entire file.
- Change grouping. The data source may require grouping the operations targeting the same physical storage. It's the case for a sharded database that must group the operations targeting the same physical node before.
- Change ordering. The data source may need to order the changes for efficient processing. For example, a merge-on-read format would require sorted changes for data and delta files to avoid nested loop join and use a streaming merge instead (data file + all delta changes on the row streamed).
To support these in-place operations, Apache Spark API has a new SupportsRowLevelOperations interface that should implement the data sources. It returns an instance of RowLevelOperationBuilder that on its turn, generates a logical representation of the DELETE/UPDATE/MERGE operation as RowLevelOperation.
The RowLevelOperation exposes 2 factory methods returning ScanBuilder and WriteBuilder instances. The ScanBuilder is the data reader that will respect the row-level operation semantic (group- vs. element-based). The WriteBuilder is responsible for writing the data. It might use some information from the ScanBuilder, like the condition used to read the data.
Besides these interfaces, Anton also added a new logical rule called RewriteDeleteFromTable to support delete operations. If the original plan supports the SupportsRowLevelOperations, the rule creates a new RowLevelOperationTable referencing the RowLevelOperation interface, and wraps it around a ReplaceData node that gets executed later by ReplaceDataExec physical node.
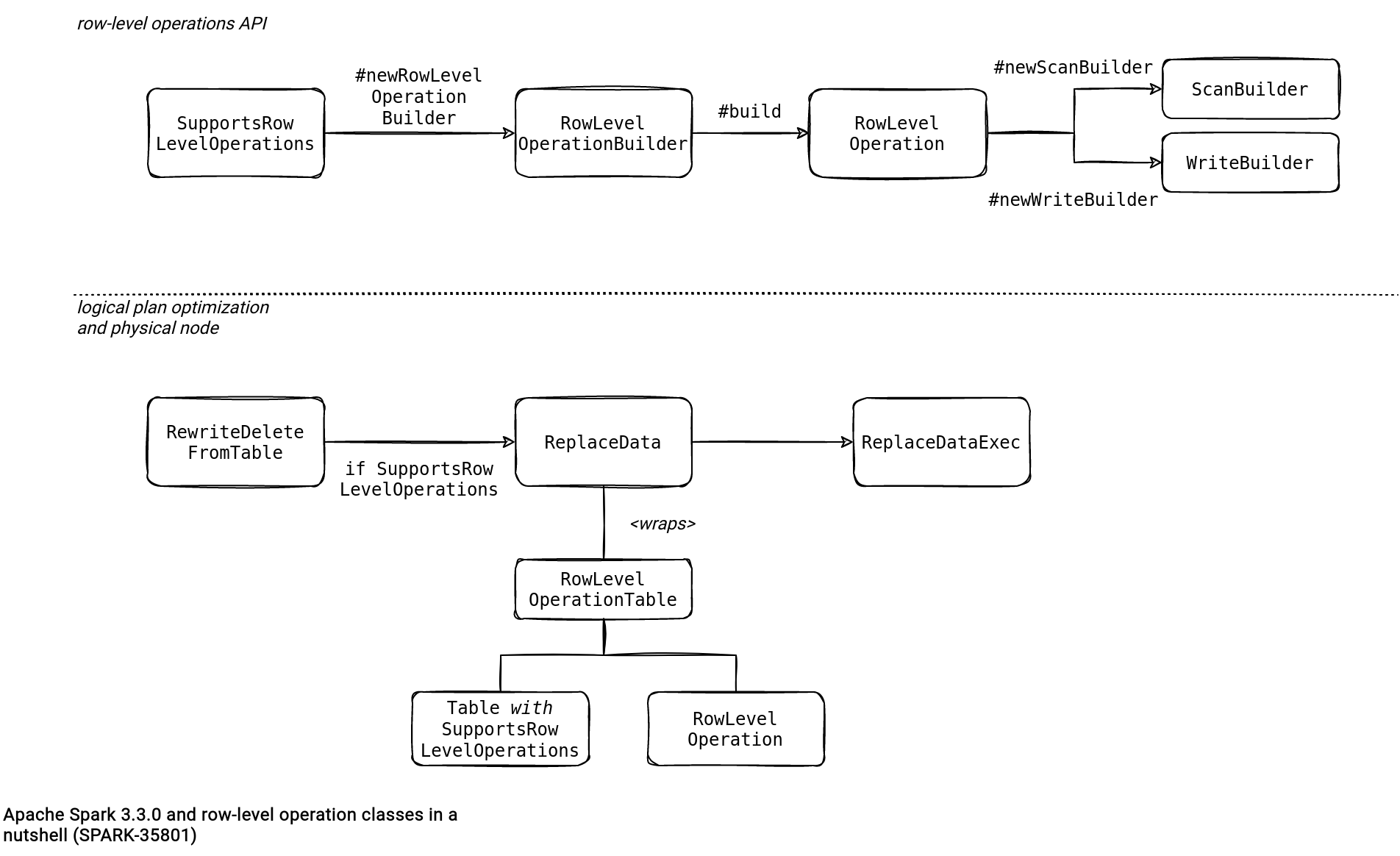
It's great to see all these innovations in the DataSource V2 API! Indexes, predicate pushdown and optimized row-level operations should help improve the performance of Apache Spark workloads. Yet, it's only the API and now it's time for the databases to implement the new interfaces!
Consulting
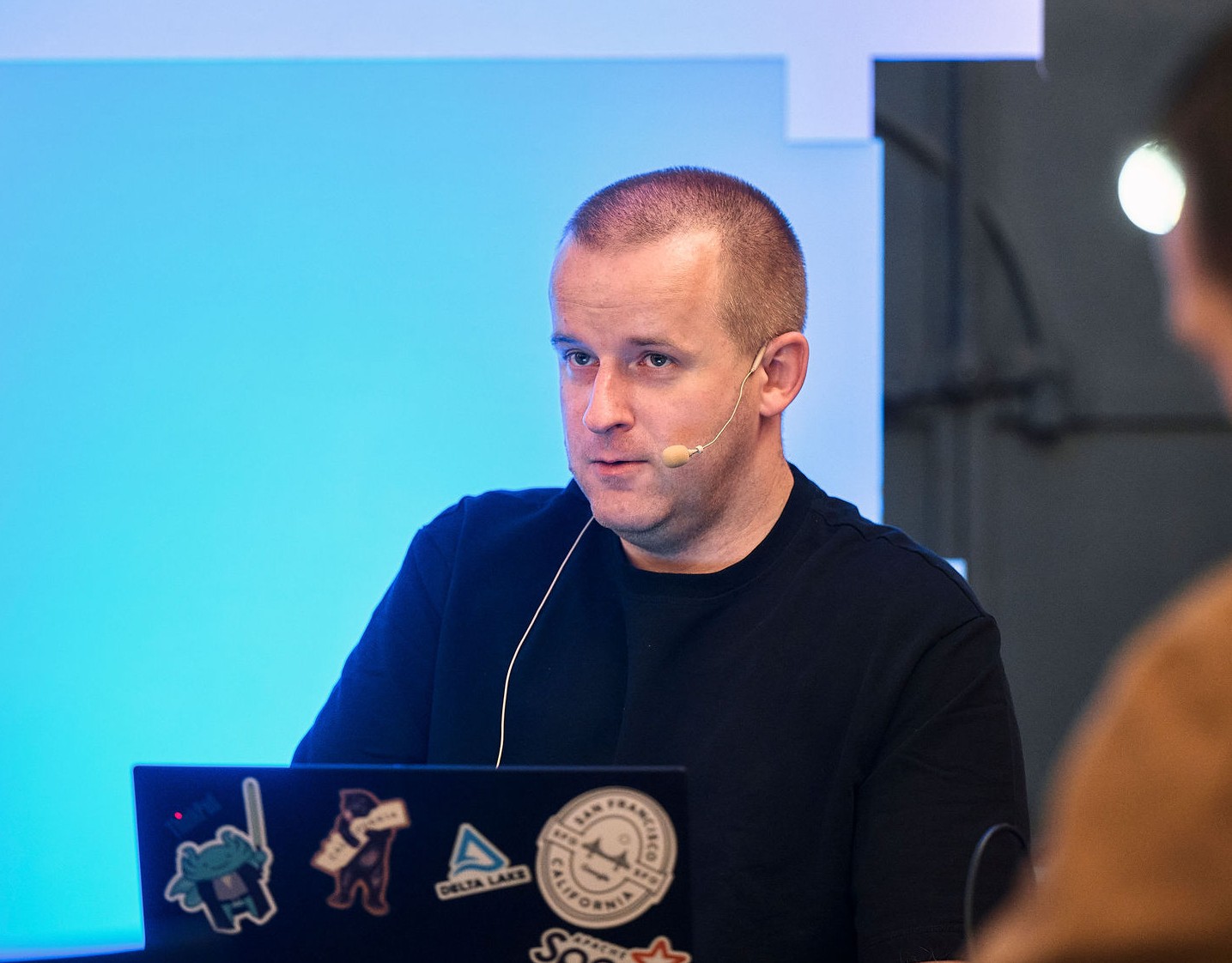
With nearly 16 years of experience, including 8 as data engineer, I offer expert consulting to design and optimize scalable data solutions.
As an O’Reilly author, Data+AI Summit speaker, and blogger, I bring cutting-edge insights to modernize infrastructure, build robust pipelines, and
drive data-driven decision-making. Let's transform your data challenges into opportunities—reach out to elevate your data engineering game today!
👉 contact@waitingforcode.com
đź”— past projects