Beginning with new tool and its CLI is never easy. Having a list of useful debugging commands is always helpful. And the rule is not different for Spark on Kubernetes project.
Data Engineering Design Patterns
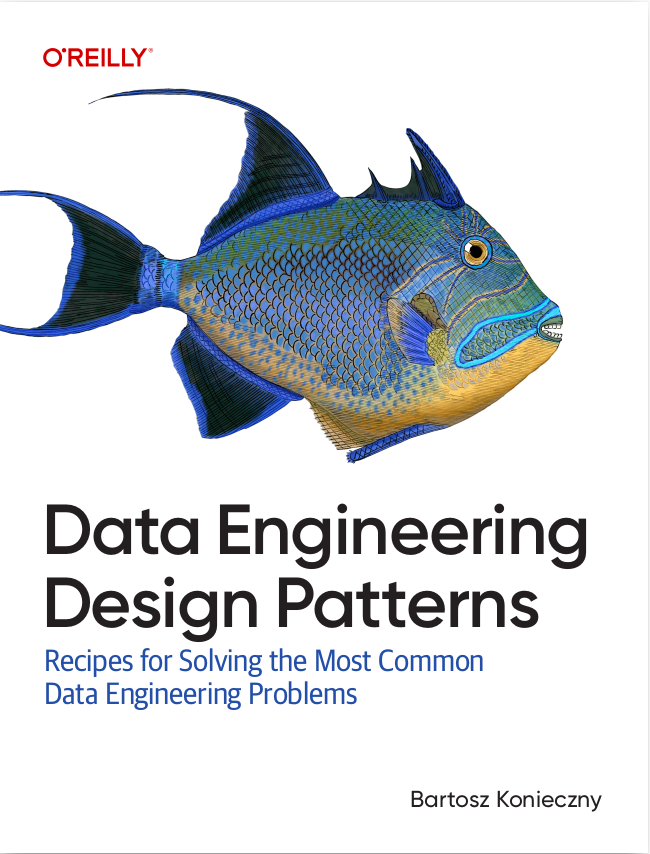
Looking for a book that defines and solves most common data engineering problems? I wrote
one on that topic! You can read it online
on the O'Reilly platform,
or get a print copy on Amazon.
I also help solve your data engineering problems 👉 contact@waitingforcode.com 📩
This post lists some kubectl commands that may be helpful in first contact with Kubernetes CLI. The commands are written in a single list and each of them is composed of a short explanation and generated output.
Among the commands that can help in the firsts contact with Spark on Kubernetes we can distinguish:
- kubectl get pods --watch - generally kubectl's get command is used to retrieve the information about Kubernetes objects. In this example we'll look for more information about pods. The extra --watch flag is used to continuously listening the changes, i.e. everytime a change happens on given object, it's automatically pushed, a little bit like tail -f.
In Spark on Kubernetes context this command is useful to see what happened with the pods, especially after first unsuccesfull tries:NAME READY STATUS RESTARTS AGE spark-pi-a33b36d1656c31039948a9d74e5f3868-driver 0/1 Error 0 2m spark-pi-ed55e575ad783c4d8997b7224f28c09e-driver 0/1 Pending 0 0s spark-pi-ed55e575ad783c4d8997b7224f28c09e-driver 0/1 Pending 0 0s spark-pi-ed55e575ad783c4d8997b7224f28c09e-driver 0/1 ContainerCreating 0 0s spark-pi-ed55e575ad783c4d8997b7224f28c09e-driver 0/1 Error 0 2s
- kubectl describe pod spark-pi-ee0e0145b94a3dcf94506235bd8c5158-driver - it prints the information about specific Kubernetes object, here Spark's driver pod. It's helpful to: investigate what happened with pod's containers (prints containers state), check if custom configuration was correctly applied (e.g. custom labels), ensure correct resources allocation or simply check object definition prepared by spark-submit client. Output's snippet can look like:
Name: spark-pi-ee0e0145b94a3dcf94506235bd8c5158-driver Namespace: default Node: docker-for-desktop/192.168.65.3 Labels: spark-app-selector=spark-923dc658b26547479570e3834aaae402 spark-role=driver Annotations: spark-app-name=spark-pi Status: Failed Containers: spark-kubernetes-driver: Container ID: docker://f63e19366f6ffae958da175a7cc5925332214318bb86c6dcc5f1b7046d781176 Image: spark:my-tag Image ID: docker://sha256:c9b6f825fbec6319a9337bfb8895e9de7e87af55ae828d9de1c0e67ffa7aebad Port:
Args: driver State: Terminated Reason: Error Exit Code: 1 Ready: False Restart Count: 0 Limits: memory: 1408Mi Requests: cpu: 1 memory: 1Gi Environment: SPARK_DRIVER_MEMORY: 1g SPARK_DRIVER_CLASS: org.apache.spark.examples.SparkPi SPARK_DRIVER_ARGS: 1000 // ... Mounts: /var/run/secrets/kubernetes.io/serviceaccount from default-token-jgd7n (ro) Conditions: Type Status Initialized True Ready False PodScheduled True Volumes: default-token-jgd7n: Type: Secret (a volume populated by a Secret) SecretName: default-token-jgd7n Optional: false QoS Class: Burstable Node-Selectors: Tolerations: node.kubernetes.io/not-ready:NoExecute for 300s node.kubernetes.io/unreachable:NoExecute for 300s Events: - kubectl cluster-info - prints cluster info, as addresses of the master and services with label kubernetes.io/cluster-service=true. In the context of Spark on Kubernetes it's useful to get the address of master required in spark-submit command. An output can look like:
Kubernetes master is running at https://localhost:6445 KubeDNS is running at https://localhost:6445/api/v1/namespaces/kube-system/services/kube-dns:dns/proxy
- kubectl logs spark-pi-ee0e0145b94a3dcf94506235bd8c5158-driver -f - retrieves logs for given Kubernetes resource. -f flag (f for follow) enables or disables logs streaming. Useless to say that this command should be the starting point for all debugging processes:
$ kubectl logs spark-pi-7f56238dc75d3162af4b7196a242392b-driver -f ++ id -u ++ id -u + myuid=0 ++ id -g + mygid=0 ++ getent passwd 0 + uidentry=root:x:0:0:root:/root:/bin/ash + '[' -z root:x:0:0:root:/root:/bin/ash ']' + SPARK_K8S_CMD=driver + '[' -z driver ']' + shift 1 + SPARK_CLASSPATH=':/opt/spark/jars/*' + env + grep SPARK_JAVA_OPT_ + sed 's/[^=]*=\(.*\)/\1/g' + readarray -t SPARK_JAVA_OPTS + '[' -n '/opt/spark/jars/spark-examples_2.11-2.3.0.jar;/opt/spark/jars/spark-examples_2.11-2.3.0.jar' ']' + SPARK_CLASSPATH=':/opt/spark/jars/*:/opt/spark/jars/spark-examples_2.11-2.3.0.jar;/opt/spark/jars/spark-examples_2.11-2.3.0.jar' + '[' -n '' ']' + case "$SPARK_K8S_CMD" in + CMD=(${JAVA_HOME}/bin/java "${SPARK_JAVA_OPTS[@]}" -cp "$SPARK_CLASSPATH" -Xms$SPARK_DRIVER_MEMORY -Xmx$SPARK_DRIVER_MEMORY -Dspark.driver.bindAddress=$SPARK_DRIVER_BIND_ADDRESS $SPARK_DRIVER_CLASS $SPARK_DRIVER_ARGS) + exec /sbin/tini -s -- /usr/lib/jvm/java-1.8-openjdk/bin/java -Dspark.driver.port=7078 -Dspark.master=k8s://https://localhost:6445 -Dspark.jars=/opt/spark/jars/spark-examples_2.11-2.3.0.jar,/opt/spark/jars/spark-examples_2.11-2.3.0.jar -Dspark.executor.instances=2 -Dspark.kubernetes.executor.podNamePrefix=spark-pi-b9eba2ce4ee33677853cf13f84119b54 -Dspark.driver.host=spark-pi-b9eba2ce4ee33677853cf13f84119b54-driver-svc.default.svc -Dspark.submit.deployMode=cluster -Dspark.app.name=spark-pi -Dspark.app.id=spark-ce9f9b930aa146559b054db1dcaa256c -Dspark.driver.blockManager.port=7079 -Dspark.kubernetes.driver.pod.name=spark-pi-b9eba2ce4ee33677853cf13f84119b54-driver -Dspark.kubernetes.container.image=spark:latest -cp ':/opt/spark/jars/*:/opt/spark/jars/spark-examples_2.11-2.3.0.jar;/opt/spark/jars/spark-examples_2.11-2.3.0.jar' -Xms1g -Xmx1g -Dspark.driver.bindAddress=0.0.0.0 org.apache.spark.examples.SparkPi 2018-06-24 11:14:02 INFO SparkContext:54 - Running Spark version 2.3.0 2018-06-24 11:14:02 WARN NativeCodeLoader:62 - Unable to load native-hadoop library for your platform... using builtin-java classes where applicable 2018-06-24 11:14:02 INFO SparkContext:54 - Submitted application: Spark Pi 2018-06-24 11:14:02 INFO SecurityManager:54 - Changing view acls to: root 2018-06-24 11:14:02 INFO SecurityManager:54 - Changing modify acls to: root 2018-06-24 11:14:02 INFO SecurityManager:54 - Changing view acls groups to: 2018-06-24 11:14:02 INFO SecurityManager:54 - Changing modify acls groups to: 2018-06-24 11:14:02 INFO SecurityManager:54 - SecurityManager: authentication disabled; ui acls disabled; users with view permissions: Set(root); groups with view permissions: Set(); users with modify permissions: Set(root); groups with modify permissions: Set()
- kubectl create -f driver_template.yaml --validate - if for some reasons (in Apache Spark 2.3 Spark on Kubernetes is still marked as experimental) one of Spark's pods is not deployed correctly, it can be debugged by template manipulations. To do so we need first to get the YAML template created by spark-submit client. It can be done with kubectl get pods spark-pi-ed55e575ad783c4d8997b7224f28c09e-driver -o yaml > driver_template.yaml command.
Later we can manipulate the template and try to validate it with --validate flag of create command. If defined, Kubernetes will use a schema to validate the template before sending it to the scheduler.
For instance, if we remove an mandatory field as container's image, we'll end up with the following message:
$ kubectl create -f /C/tmp/template_test.yaml --validate The Pod "spark-pi-ed55e575ad783c4d8997b7224f28c09e-driver" is invalid: spec.containers[0].image: Required value
- kubectl delete pod spark-pi-7826b7948b3539b8a74ddd909da31da3-driver - as the name points out, this command deletes Kubernetes object (pod in this case). It can be useful if, due of a misconfiguration, a pod remains stuck for too long. The execution of delete gives the following results:
$ kubectl delete pod spark-pi-c0d471fa3f46318a8e8a754cdb9706d6-driver pod "spark-pi-c0d471fa3f46318a8e8a754cdb9706d6-driver" deleted
- kubectl port-forward spark-pi-8663fb7f8d2531b29975461b62ae1cda-driver 4040:4040 - natively Apache Spark UI will be executed locally to the pod. But we can expose it in our localhost by simply forwarding 4040 port from the pod to the host (exactly as for Docker containers). It can be done with port-forward command and the following output should be printed after doing that:
$ kubectl port-forward spark-pi-8663fb7f8d2531b29975461b62ae1cda-driver 4040:4040 Forwarding from 127.0.0.1:4040 -> 4040 Handling connection for 4040 Handling connection for 4040 Handling connection for 4040 Handling connection for 4040 Handling connection for 4040 Handling connection for 4040
- kubectl get secrets - Spark programs can use secrets to manipulate sensitive configuration as credentials. They can be defined inside spark.kubernetes.driver.secrets.spark-secret and spark.kubernetes.executor.secrets.spark-secret properties. To visualize which secrets are defined for given namespace, get secrets command may be used:
$ kubectl get secrets NAME TYPE DATA AGE default-token-jgd7n kubernetes.io/service-account-token 3 17d
To go even deeper, each secret can be viewed with already presented describe command, like that: kubectl describe secrets/default-token-jgd7n. - kubectl get namespaces - if you intend to test Spark on Kubernetes inside separate namespace, you can check which ones are already defined with get namespaces command. Its execution returns:
$ kubectl get namespaces NAME STATUS AGE default Active 17d docker Active 17d kube-public Active 17d kube-system Active 17d spark-tests Active 12d
Since namespace is also a Kubernetes object, we can also view its properties with describe command:
$ kubectl describe namespace spark-tests Name: spark-tests Labels:
Annotations: Status: Active No resource quota. No resource limits. - kubectl describe nodes - once again another describe version. This time it lets us to see what happens in our cluster's nodes. The command shows pods located in given node as well as used and allocable resources:
Capacity: cpu: 3 memory: 4023128Ki pods: 110 Allocatable: cpu: 3 memory: 3920728Ki pods: 110 System Info: Non-terminated Pods: (9 in total) Namespace Name CPU Requests CPU Limits Memory Requests Memory Limits --------- ---- ------------ ---------- --------------- ------------- docker compose-5d4f4d67b6-xx72m 0 (0%) 0 (0%) 0 (0%) 0 (0%) docker compose-api-7bb7b5968f-twrbp 0 (0%) 0 (0%) 0 (0%) 0 (0%) kube-system etcd-docker-for-desktop 0 (0%) 0 (0%) 0 (0%) 0 (0%) kube-system kube-apiserver-docker-for-desktop 250m (8%) 0 (0%) 0 (0%) 0 (0%) kube-system kube-controller-manager-docker-for-desktop 200m (6%) 0 (0%) 0 (0%) 0 (0%) kube-system kube-dns-6f4fd4bdf-9f7sn 260m (8%) 0 (0%) 110Mi (2%) 170Mi (4%) kube-system kube-proxy-r78gr 0 (0%) 0 (0%) 0 (0%) 0 (0%) kube-system kube-scheduler-docker-for-desktop 100m (3%) 0 (0%) 0 (0%) 0 (0%) kube-system kubernetes-dashboard-5bd6f767c7-cf2jg 0 (0%) 0 (0%) 0 (0%) 0 (0%) Allocated resources: (Total limits may be over 100 percent, i.e., overcommitted.) CPU Requests CPU Limits Memory Requests Memory Limits ------------ ---------- --------------- ------------- 810m (27%) 0 (0%) 110Mi (2%) 170Mi (4%)
It can be useful to check the impact of our Spark application on the cluster at node level. We can also analyze one specific node by defining its name in the command.
The post listed some interesting commands that can help us to start working with Spark on Kubernetes. Among them we can find a lot of kubectl describe examples thanks to which we can easily see what is really executed (e.g. pod specification). We can also see more network-related commands as the one for proxy forwarding letting us to see Spark's driver UI. The last category of commands concerns listing and is executed with kubectl get.
Consulting
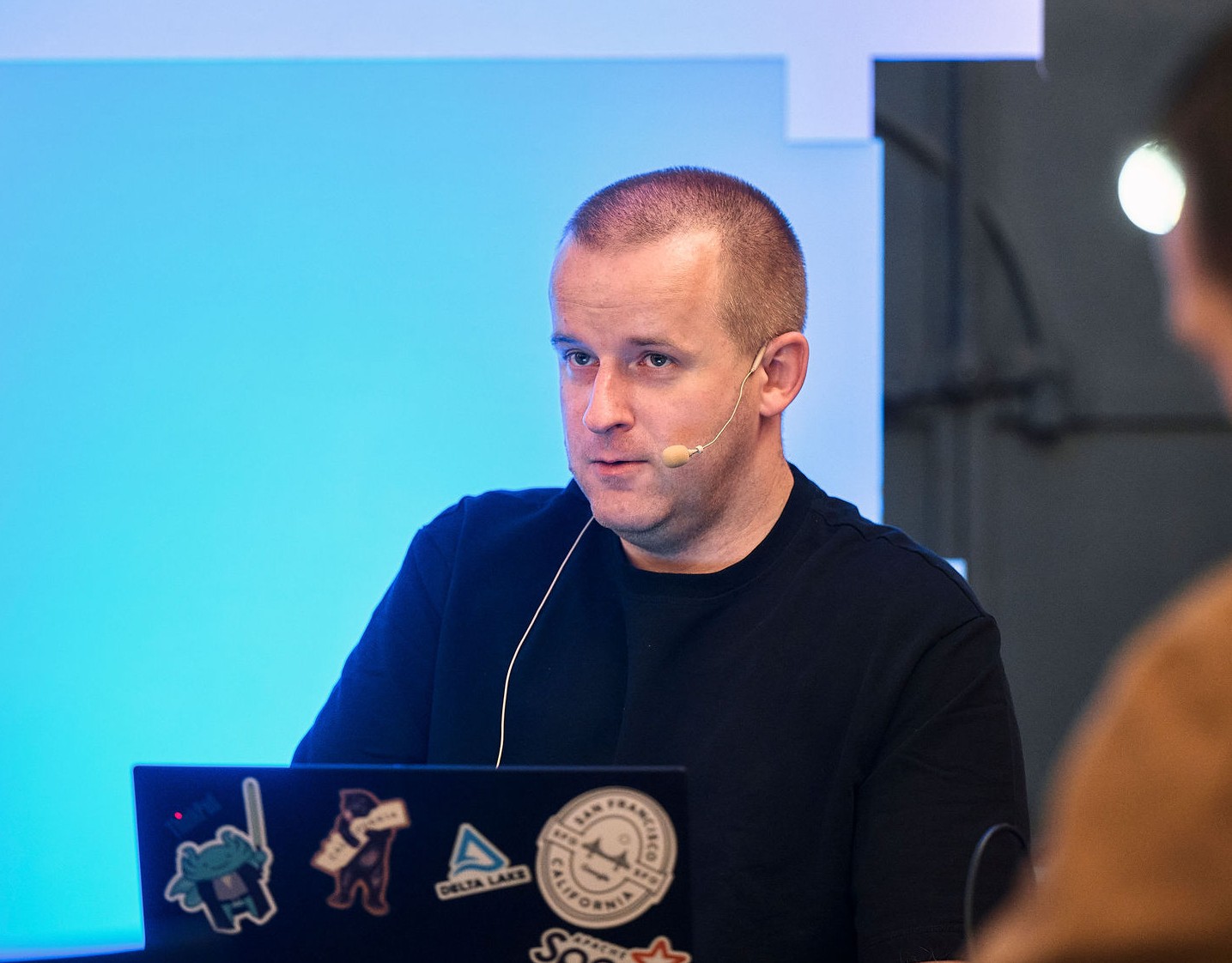
With nearly 16 years of experience, including 8 as data engineer, I offer expert consulting to design and optimize scalable data solutions.
As an O’Reilly author, Data+AI Summit speaker, and blogger, I bring cutting-edge insights to modernize infrastructure, build robust pipelines, and
drive data-driven decision-making. Let's transform your data challenges into opportunities—reach out to elevate your data engineering game today!
👉 contact@waitingforcode.com
đź”— past projects