Few times ago I got an interesting question in the comment about slowly changing dimensions data. Shame on me, but I encountered this term for the first time. After a quick search, I found some basic information and made a decision to document it in this blog post.
Data Engineering Design Patterns
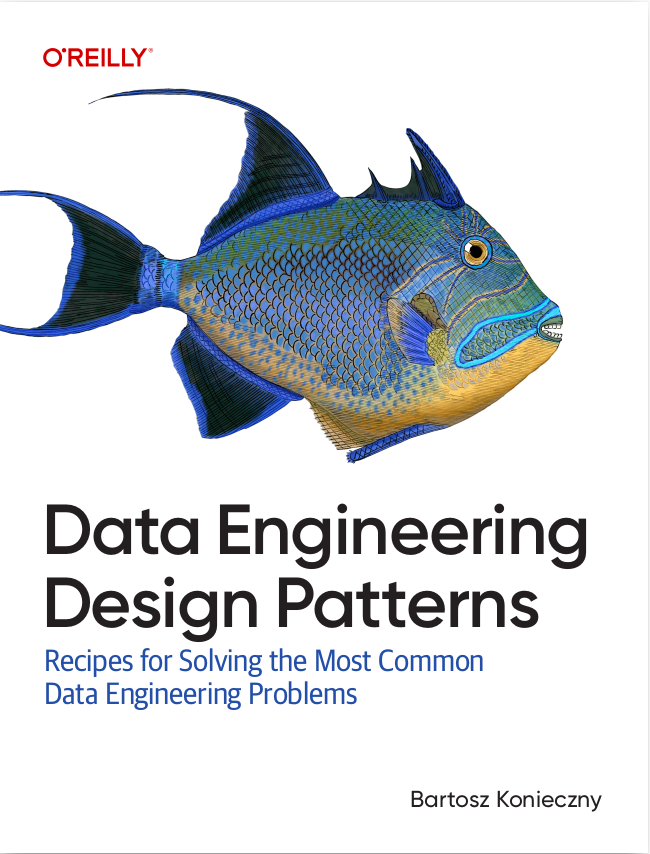
Looking for a book that defines and solves most common data engineering problems? I wrote
one on that topic! You can read it online
on the O'Reilly platform,
or get a print copy on Amazon.
I also help solve your data engineering problems 👉 contact@waitingforcode.com 📩
In the first part of the post I will explain the idea behind slowly changing dimensions. Later, I will show some code and show how we could address each type with Apache Spark SQL.
Definition
In simple terms, slowly changing dimension defines the update strategy on the data. The easiest way to ensure this is by making in-place changes, a little bit like with SQL updates, UPDATE table SET a = "1", b = "2" WHERE id = 30. The problem with these queries is that, despite their simplicity, they decrease the security of your data. Let's imagine how to "rollback" the change and apply different business logic, maybe because we misunderstood the former one. With in-place changes and no raw data to regenerate the dataset from scratch, it can be hard.
That's where slowly changing dimension types came in. You will find different types but I really appreciated the definition from What are Slowly Changing Dimensions? which is quite clear and helps understand the different types pretty well. To summarize these techniques, we can distinguish the following types:
- type 0 - no specific action performed.
- type 1 - data is overwritten in-place, like with my UPDATE...SET example
- type 2 - uses a concept of active/inactive row. When new information is present for a row, it's used to build a completely new row flagged as "active". Previous "active" row passes to "inactive" state. In addition to the state transition, the columns with dates storing the validity period are updated accordingly.
- type 3 - the table has one extra column per updatable field. The currently used values are stored in "current_"-prefixed columns whereas previously used in "previous_"-prefixed columns. The history is then limited by the number of "previous" columns.
- type 4 - the table looks like an append-only log file system. Every change is added at the end of the table. In addition to that, every new row for given key changes the validity period of the previously active row.
- type 6 - the combination of types 1, 2 and 3 (1+2+3 = 6), so you will retrieve a table with: "previous"-prefixed column, validity period and active/inactive flag.
I will now try to implement the types 1, 2, 3 and 4 on Apache Spark SQL.
Slowly changing dimensions and Apache Spark
In my examples I will start with the simplest one, type 1. You can do it in many different ways. You can write a map function that will always take the fields from the new dataset, if defined, or from the old one otherwise. I did a similar thing but with CASE - SQL if-else and one mapping method to handle all the cases:
it should "overwrite changes in type 1" in { val currentDataset = Seq( Providers(1L, "provider A", "Big Data"), Providers(2L, "provider B", "cloud"), Providers(3L, "provider C", "software engineering"), Providers(4L, "provider D", "unknown") ).toDF() val newDataset = Seq( Providers(4L, "provider D", "QA") ).toDF() def aliasColumns(prefix: String, schema: StructType): Seq[Column] = { schema.fields.map(field => functions.col(field.name).as(s"${prefix}${field.name}")) } val currentDatasetAliases = currentDataset.select( aliasColumns("current_", currentDataset.schema): _* ) val newDatasetAliased = newDataset.select( aliasColumns("new_", currentDataset.schema): _* ) def generateMapping(schema: StructType): Seq[Column] = { schema.fields.map(field => { functions.when(functions.col(s"new_${field.name}").isNull, functions.col(s"current_${field.name}")) .otherwise(functions.col(s"new_${field.name}")).as(s"${field.name}") }) } // I'm doing the overwrite with a custom SELECT but you can also // do it in .map(...) function with only 1 if-else condition: // `if row.getAs[String]("new_name") != null ==> map to the new // otherwise map to the old. Of course, it will only work when // all columns, even the ones that didn't change, are defined in the // new dataset. val mappedProviders = currentDatasetAliases .join(newDatasetAliased, $"current_id" === $"new_id", "full_outer") .select(generateMapping(currentDataset.schema): _*).as[Providers] .collect() mappedProviders should have size 4 mappedProviders should contain allOf( Providers(1L, "provider A", "Big Data"), Providers(2L, "provider B", "cloud"), Providers(3L, "provider C", "software engineering"), Providers(4L, "provider D", "QA") ) } case class Providers(id: Long, name: String, specialty: String)
The second method, type 2, creates a new additional row and changes the validity period of the previous active row. The easiest way to do so is to use a groupByKey expression and in the flatMapGroups function check whether there is a new value. It works and probably will satisfy 99% of cases. But I tried to do it a smarter way to avoid data materialization. To avoid that, I used this grouping expression:
if (provider.startDate.isEmpty || provider.currentFlag.getOrElse("") == "Y") { s"possible_change-${provider.id}" } else { provider.id.toString }
It simply checks whether we deal with currently active row (...== "Y") or a new value row (startDate.isEmpty). If it's the case, we can group only these 2 rows since only these 2 will need a change. If it's not the case, we can use the id of the entity. Later, in the mapping function, we apply 2 different mapping strategies. The whole example looks like that:
it should "create new active flag for type 2" in { val currentDataset = Seq( ProvidersType2(1L, "provider A", "software", Some(new Timestamp(0L)), Some(new Timestamp(1L)), Some("N")), ProvidersType2(1L, "provider A", "software & Big Data", Some(new Timestamp(1L)), Some(new Timestamp(3L)), Some("N")), ProvidersType2(1L, "provider A", "Big Data", Some(new Timestamp(3L)), None, Some("Y")), ProvidersType2(2L, "provider B", "cloud", Some(new Timestamp(3L)), None, Some("Y")), ProvidersType2(3L, "provider C", "software engineering", Some(new Timestamp(3L)), None, Some("Y")), ProvidersType2(4L, "provider D", "unknown", Some(new Timestamp(3L)), None, Some("Y")) ).toDF() val newDataset = Seq( ProvidersType2(4L, "provider D", "QA"), ProvidersType2(5L, "provider E", "data science") ).toDF() val changeDateMillis = 5L val broadcastedChangeDateMillis = testSparkSession.sparkContext.broadcast(changeDateMillis) val mappedProviders = currentDataset.union(newDataset).as[ProvidersType2] .groupByKey(provider => { if (provider.startDate.isEmpty || provider.currentFlag.getOrElse("") == "Y") { s"possible_change-${provider.id}" } else { provider.id.toString } }) .flatMapGroups { case (key, records) => { if (key.startsWith("possible_change")) { val mappedRecords = new mutable.ListBuffer[ProvidersType2]() var shouldOverwrite = false while (records.hasNext) { // The logic behind this is straightforward. // We suppose that we'll have at most 2 records in this group because // at a given moment we can only have 1 active row and, eventually, // 1 candidate row. If the latter happens, the `shouldOverwrite` will be true // and therefore, we'll need to change the state of every row accordingly // Another solution would be to group by the `id` property and materialize the whole // dataset at once but I tried to do it "smart way", without the data materialization val recordToMap = records.next() shouldOverwrite = (shouldOverwrite || records.hasNext) if (shouldOverwrite) { mappedRecords.append(recordToMap.transitToNewState(broadcastedChangeDateMillis.value)) } else { // If we have only 1 row here, it means either that it didn't change or // that it's the new row. `enableIfDisabled` will do nothing // for the former case. It will only promote the new row // as the currently used. mappedRecords.append(recordToMap.enableIfDisabled(broadcastedChangeDateMillis.value)) } } mappedRecords } else { records } } }.collect() mappedProviders should have size 8 mappedProviders should contain allOf( ProvidersType2(1L, "provider A", "software", Some(new Timestamp(0L)), Some(new Timestamp(1L)), Some("N")), ProvidersType2(1L, "provider A", "software & Big Data", Some(new Timestamp(1L)), Some(new Timestamp(3L)), Some("N")), ProvidersType2(1L, "provider A", "Big Data", Some(new Timestamp(3L)), None, Some("Y")), ProvidersType2(2L, "provider B", "cloud", Some(new Timestamp(3L)), None, Some("Y")), ProvidersType2(3L, "provider C", "software engineering", Some(new Timestamp(3L)), None, Some("Y")), ProvidersType2(4L, "provider D", "unknown", Some(new Timestamp(3L)), Some(new Timestamp(5L)), Some("N")), ProvidersType2(4L, "provider D", "QA", Some(new Timestamp(5L)), None, Some("Y")), ProvidersType2(5L, "provider E", "data science", Some(new Timestamp(5L)), None, Some("Y")) ) } case class ProvidersType2(id: Long, name: String, specialty: String, startDate: Option[Timestamp] = None, endDate: Option[Timestamp] = None, currentFlag: Option[String] = None) { def transitToNewState(dateTimeMillis: Long): ProvidersType2 = { if (currentFlag == ProvidersType2.EnabledFlag) { disable(dateTimeMillis) } else { enable(dateTimeMillis) } } def disable(dateTimeMillis: Long): ProvidersType2 = { this.copy(endDate = Some(new Timestamp(dateTimeMillis)), currentFlag = ProvidersType2.DisabledFlag) } def enable(dateTimeMillis: Long): ProvidersType2 = { this.copy(startDate = Some(new Timestamp(dateTimeMillis)), currentFlag = ProvidersType2.EnabledFlag) } def enableIfDisabled(dateTimeMillis: Long): ProvidersType2 = { if (currentFlag.isEmpty) { this.copy(startDate = Some(new Timestamp(dateTimeMillis)), currentFlag = ProvidersType2.EnabledFlag) } else { this } } } object ProvidersType2 { val EnabledFlag = Some("Y") val DisabledFlag = Some("N") }
To implement the update method for type 3, I used a similar approach to type 1, ie. with dynamic if-else cases. The drawback is that I didn't find a smart way to not repeat the schema in the generateMapping function. Also, I'm simply verifying whether the columns are not empty but we could (should?) enforce this and compare the values between columns (current and previous) to not update the previous one like in this example (provider D):
it should "switch new/old columns for type 3" in { val currentDataset = Seq( ProvidersType3(1L, "provider A", None, "Big Data", None), ProvidersType3(2L, "provider B", None, "cloud", None), ProvidersType3(3L, "provider C", None, "software engineering", None), ProvidersType3(4L, "provider D", None, "unknown", None) ).toDF() val newDataset = Seq( ProvidersType3(4L, "provider D", None, "QA", None), ProvidersType3(5L, "provider E", None, "DevOps", None) // This will be our new row ).toDF() def aliasColumns(prefix: String, schema: StructType): Seq[Column] = { schema.fields.map(field => functions.col(field.name).as(s"${prefix}${field.name}")) } val currentDatasetAliases = currentDataset.select( aliasColumns("current_", currentDataset.schema): _* ) val newDatasetAliased = newDataset.select( aliasColumns("new_", currentDataset.schema): _* ) def generateMapping(staticColumns: Seq[String], columnsToSwitch: Seq[String]): Seq[Column] = { val staticColumnNames = staticColumns.map(field => { functions.when(functions.col(s"new_${field}").isNull, functions.col(s"current_${field}")) .otherwise(functions.col(s"new_${field}")).as(s"${field}") }) val currentNames = columnsToSwitch.map(field => { functions .when(functions.col(s"new_${field}").isNotNull, functions.col(s"new_${field}")) .otherwise(functions.col(s"current_${field}")).as(s"${field}") }) val previousNames = columnsToSwitch.map(field => { functions .when(functions.col(s"new_${field}").isNotNull, functions.col(s"current_${field}")) .otherwise(functions.col(s"current_previous${field.capitalize}")).as(s"previous${field.capitalize}") }) staticColumnNames ++ currentNames ++ previousNames } val mappedProviders = currentDatasetAliases .join(newDatasetAliased, $"current_id" === $"new_id", "full_outer") .select( generateMapping(Seq("id"), Seq("name", "specialty")): _*).as[ProvidersType3] .collect() mappedProviders should have size 5 mappedProviders should contain allOf( ProvidersType3(1, "provider A", None, "Big Data",None), ProvidersType3(2, "provider B", None, "cloud",None), ProvidersType3(3, "provider C", None, "software engineering", None), ProvidersType3(4, "provider D", Some("provider D"), "QA" , Some("unknown")), ProvidersType3(5, "provider E", None, "DevOps", None) ) } case class ProvidersType3(id: Long, name: String, previousName: Option[String] = None, specialty: String, previousSpecialty: Option[String] = None)
Compared to the previous type, type 4 is relatively easy since we don't need to switch columns. The only thing to do is to check whether we should modify the validity period. And for that, we can slightly modify the type 2's solution:
it should "add new row and update validity period for type 4" in { val currentDataset = Seq( ProvidersType4(1L, "provider A", "software", Some(new Timestamp(0L)), Some(new Timestamp(1L))), ProvidersType4(1L, "provider A", "software & Big Data", Some(new Timestamp(1L)), Some(new Timestamp(3L))), ProvidersType4(1L, "provider A", "Big Data", Some(new Timestamp(3L)), None), ProvidersType4(2L, "provider B", "cloud", Some(new Timestamp(3L)), None), ProvidersType4(3L, "provider C", "software engineering", Some(new Timestamp(3L)), None), ProvidersType4(4L, "provider D", "unknown", Some(new Timestamp(3L)), None) ).toDF() val newDataset = Seq( ProvidersType4(4L, "provider D", "QA"), ProvidersType4(5L, "provider E", "backend engineering") ).toDF() val changeDateMillis = 5L val broadcastedChangeDateMillis = testSparkSession.sparkContext.broadcast(changeDateMillis) val mappedProviders = currentDataset.union(newDataset).as[ProvidersType4] .groupByKey(provider => { // Handles both, new and old active row if (provider.endDate.isEmpty) { s"possible_change-${provider.id}" } else { provider.id.toString } }) .flatMapGroups { case (key, records) => { if (key.startsWith("possible_change")) { val mappedRecords = new mutable.ListBuffer[ProvidersType4]() var shouldOverwrite = false while (records.hasNext) { val recordToMap = records.next() shouldOverwrite = (shouldOverwrite || records.hasNext) if (shouldOverwrite) { mappedRecords.append(recordToMap.transitToNewState(broadcastedChangeDateMillis.value)) } else { mappedRecords.append(recordToMap.enableIfDisabled(broadcastedChangeDateMillis.value)) } } mappedRecords } else { records } } }.collect() mappedProviders should have size 8 mappedProviders should contain allOf( ProvidersType4(1L, "provider A", "software", Some(new Timestamp(0L)), Some(new Timestamp(1L))), ProvidersType4(1L, "provider A", "software & Big Data", Some(new Timestamp(1L)), Some(new Timestamp(3L))), ProvidersType4(1L, "provider A", "Big Data", Some(new Timestamp(3L)), None), ProvidersType4(2L, "provider B", "cloud", Some(new Timestamp(3L)), None), ProvidersType4(3L, "provider C", "software engineering", Some(new Timestamp(3L)), None), ProvidersType4(4L, "provider D", "unknown", Some(new Timestamp(3L)), Some(new Timestamp(5L))), ProvidersType4(4L, "provider D", "QA", Some(new Timestamp(5L)), None), ProvidersType4(5L, "provider E", "backend engineering", Some(new Timestamp(5L)), None) ) } case class ProvidersType4(id: Long, name: String, specialty: String, startDate: Option[Timestamp] = None, endDate: Option[Timestamp] = None) { def transitToNewState(dateTimeMillis: Long): ProvidersType4 = { if (startDate.nonEmpty && endDate.isEmpty) { disable(dateTimeMillis) } else { enable(dateTimeMillis) } } def disable(dateTimeMillis: Long): ProvidersType4 = { this.copy(endDate = Some(new Timestamp(dateTimeMillis))) } def enable(dateTimeMillis: Long): ProvidersType4 = { this.copy(startDate = Some(new Timestamp(dateTimeMillis))) } def enableIfDisabled(dateTimeMillis: Long): ProvidersType4 = { if (startDate.isEmpty) { this.copy(startDate = Some(new Timestamp(dateTimeMillis))) } else { this } } }
After these few quick exercises, I was able to understand the concept of the slowly changing dimensions a little bit better. When I first saw the different types, I found that unconsciously I used the type 4 a few times and never saw its official name :P Nonetheless, all described types are worth to know since as for everything, the "story of trade-offs" can make you chose one or another ;)
Consulting
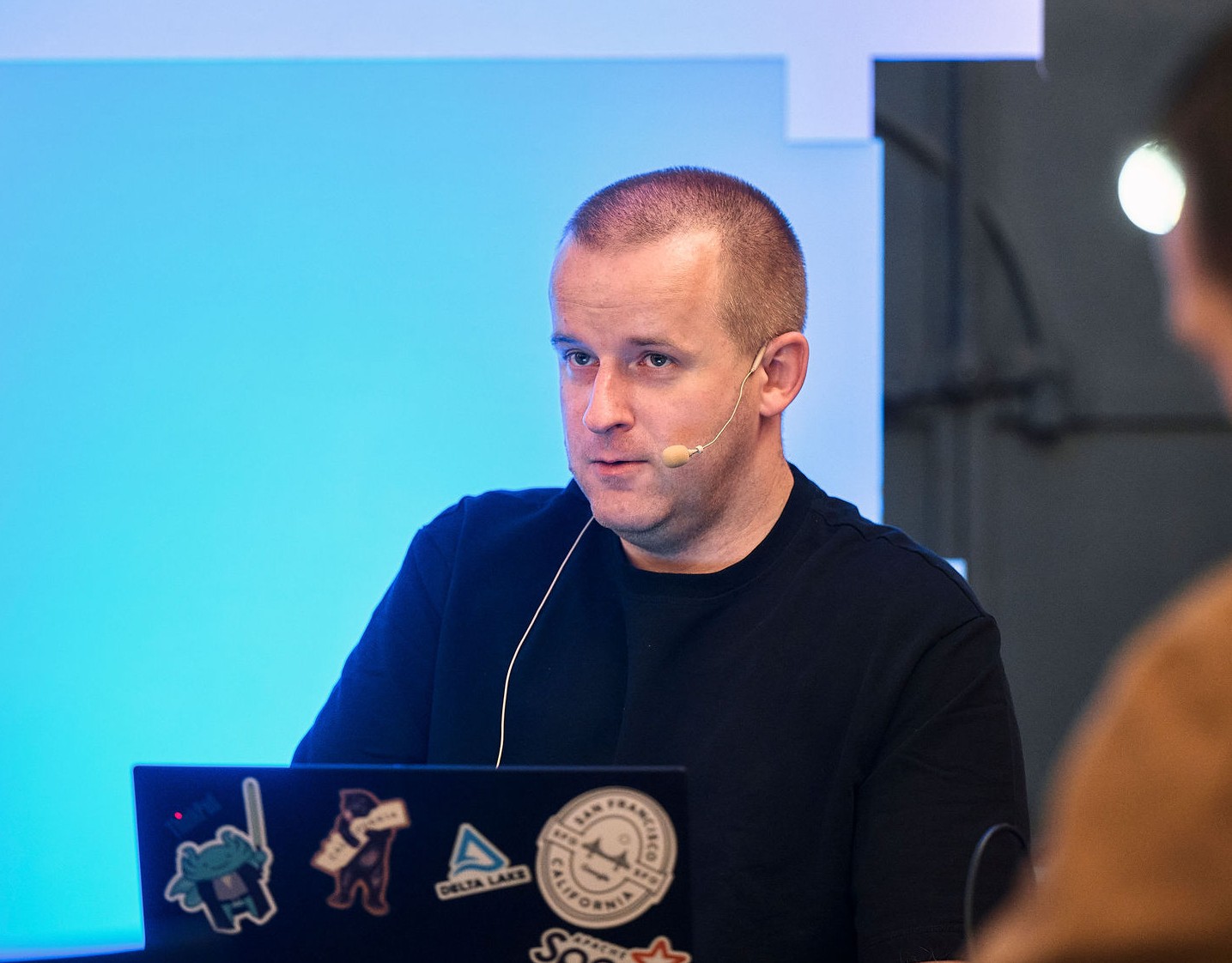
With nearly 16 years of experience, including 8 as data engineer, I offer expert consulting to design and optimize scalable data solutions.
As an O’Reilly author, Data+AI Summit speaker, and blogger, I bring cutting-edge insights to modernize infrastructure, build robust pipelines, and
drive data-driven decision-making. Let's transform your data challenges into opportunities—reach out to elevate your data engineering game today!
👉 contact@waitingforcode.com
đź”— past projects