Dealing with a lot of data is a time consuming activity but dealing with a lot of data and ensuring its high value is even more complicated. It's one of the reasons why the data quality should never be neglected. After all, it's one of components providing accurate business insights and facilitating strategic decisions.
Data Engineering Design Patterns
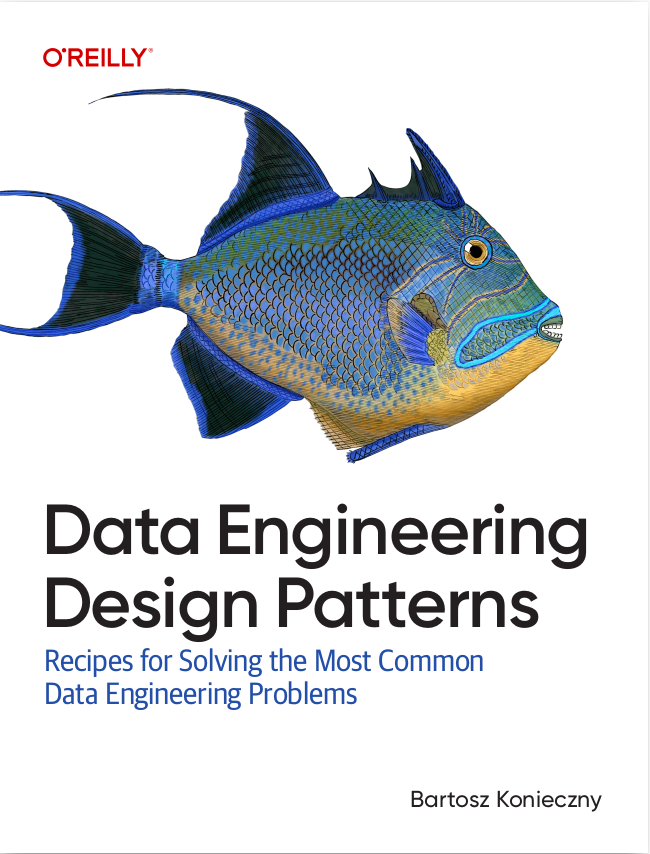
Looking for a book that defines and solves most common data engineering problems? I wrote
one on that topic! You can read it online
on the O'Reilly platform,
or get a print copy on Amazon.
I also help solve your data engineering problems 👉 contact@waitingforcode.com 📩
This post focuses on data quality aspect in data-driven systems. The first part defines the data quality and explains its main axis. The second part shows with what tools the data quality can be provided and at what moment. The last section contains some more important questions that could help to ensure a good data quality in data-driven systems.
Definition
The data quality defines how the data meets the needs of the user requirements. A data of good quality can be used easily. It also respects respecting business requirements. One of its main consequences are more accurate business decisions.
The data quality can be characterized by the following metrics:
- Accuracy - the data must be accurate, i.e. it must be correct. The corectness means here: validity and unambiguity. The data is considered as valid when it fits to a set of accepted values. It's called unambigious when all its occurencies have the same representation (e.g. dates represented with the same format everywhere)
- Integrity - focuses on the data validity accross the relationships. For intance if our database stores orders and customers, it should never happen to have an order without a customer related to it.
- Consistency - this point means that the data must be consistent accross different data sets. An example of incosistent data can be the perception of a customer concept caused by 2 separated definitions. According to one of them, a customer may be somebody with at least 1 realized order. For the other dataset, a customer can be simply someone who registered on our e-commerce store. With such differences it's not difficult to produce discrepancies, especially when both datasets are combined together at a given moment.
- Completeness - all required properties must be defined in order to validate the data. For instance if we're processing orders passed in our e-commerce and some of them miss total order price, then we can consider the dataset as incomplete.
- Validity - the data must be valid from the point of view of business rules. For instance, one rule can define the range of accepted values for hours as [0 - 23]. Thus the end users are not supposed to retrieve time defined in 1PM or 1AM format.
- Timeliness - the data must be available when needed. For instance, if one end user needs the data with 1 day delay (data from tomorrow for today), then the requirement for the data quality will be to provide all needed values at daily basis.
- Accessibility - the data must be easily accessible, e.g. through a web UI dedicated for the end users. Moreover, this point brings also the trait related to data understandig. The users should be able to retrieve the documentation related to the data, for instance a document defining the meaning of all fields for a given table.
Guaranteeing data quality
As you can see, the list is quite long - even though it's not described in details. All of above points can be guaranteed with the use of different methods, at different stages of the processing pipeline, for instance:
- at development level - the engineers ensure that their code fits for data quality requirements by writing the unit tests. They validate the data processing pipeline at the smallest level
- integration tests - this level concerns the whole data processing pipeline and it helps to ensure that the data is correctly processed from the beginning to the end
- data validation - executed on the data already stored in the database. This step is very often realized with ad-hoc queries in order to ensure: data copleteness and accuracy. It should be done by a person coming from business side, or at least with his help
- user acceptance - the end users validate that the provided data fits to their needs
- performance tests - this layer is responsible to ensure that the data processing pipelines scales with current data load and will scale with an increased load
- regression tests - as in the case of all software, this step ensures that the new development doesn't break already existent one
Data quality checklist
Data quality is not a young domain and it's why we can find some interesting resources pointing out the most important concepts. Below list contains some of more frequent questions to answer in order to improve the data quality, especially from the engineering point of view:
- Does the data contain duplicates and redundancy ? An example: 2 different records mean the same thing (redundancy) or 2 or more the same events are injected to the system (duplicates). If it happens, do the writes on the database will be idempotent (deduplication) or not (append) ?
- Is a business unit involved in the data quality management ? An example: data engineering team underestimated the importance of some indicators and the company decisional capacity decreased because of that. The business unit implication can help to ensure that the data comply to the business rules.
- Does the data have the same representation over datasets ? The consistency is a king. For instance if in one dataset the countries are represented as ISO codes and in the other as English fullnames, it'll bring maintenance problems soon or later. And if each particular values are supposed to have the same representation, are they defined in a documentation ?
- The data must be standardized ? An example: one property comes from different external data providers. In order to guarantee the data consistency, we'll need to translate them to the values belonging to a single and shared dictionary (point related to data representation over dataset).
- Is the data correct ? An example: we're running a real-time pipeline and we receive the events which event time is older than 1 month. This question brings another: Is the data correct within the aggregation unit ? An example: the system tracking user's behavior on a website with the cookie. We suppose to have the cookie defined for every event but sometimes it's missing (within the same session).
- What is the type of storage - immutable or mutable ? If the storage is mutable, then it's important to know what is the procedure of recovery in case of data generation problems ?
- What is the data freshness ? An example: the real-time pipeline should be able to provide even an approximative insight in near real-time. It's less true for batch pipelines executed for instance at the end of a day to process the accumulated data at once.
- What happens with the error data ? This data is monitored and fixed ? Do the impacted business units are aware of the problems ?
- Is the data enriched ? An example: tracking events about the user's activity on a website can be enriched with a lot of information describing the user, his previous activity, the context of its visit (A/B testing case) and so on.
- Does the data quality is measured ? If yes, how often it's measured and who defined the measures ?
- Does the data pipeline scale out ? An example: a successful marketing campaign (who would complaint about it?) and the data pipeline unable to absorb the increased load - in consequence, slower business decision process or even worse, some data lost.
- How the data processing pipeline is monitored ? An example: automatic validation metrics (e.g. when no data during the last X minutes) sent to support/engeenering team or visualization tools.
This posts gave some indicators emphasizing the data quality importance. We could summarize the data quality as a process involving business units and engineering team. The business unit is needed to define the rules helping to understand the data and its important values. The engineering team is there to ensure the reliability, the implementation of business rules, the scaling and the monitoring of the processing pipeline. The last section provided some non exhaustive mlist of questions that should help in the data quality management process.
Consulting
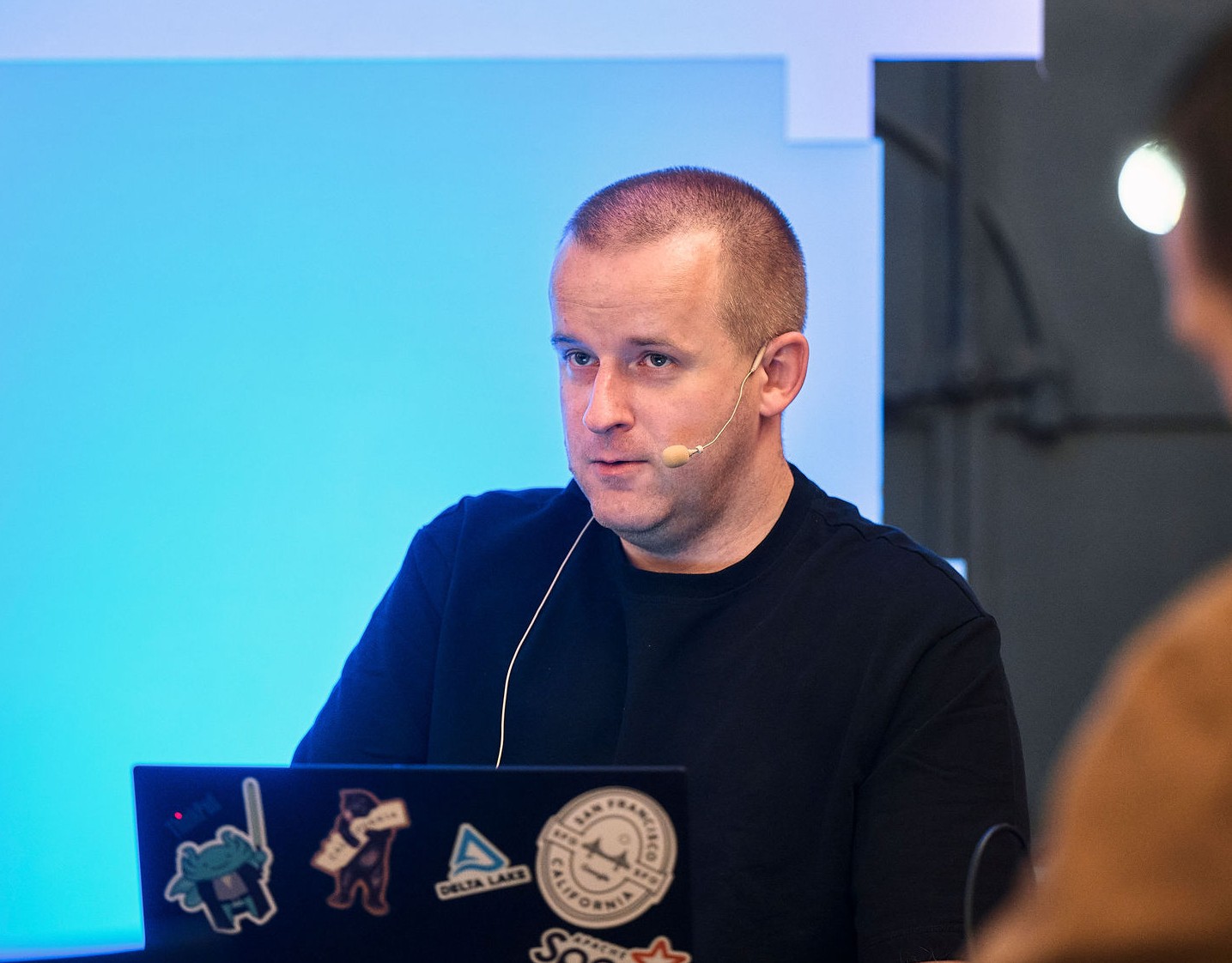
With nearly 16 years of experience, including 8 as data engineer, I offer expert consulting to design and optimize scalable data solutions.
As an O’Reilly author, Data+AI Summit speaker, and blogger, I bring cutting-edge insights to modernize infrastructure, build robust pipelines, and
drive data-driven decision-making. Let's transform your data challenges into opportunities—reach out to elevate your data engineering game today!
👉 contact@waitingforcode.com
đź”— past projects