Querying big amounts of data has never been so simple as nowadays. Amazon Redshift and Azure SQL Data Warehouse are one of the solutions. But using them wouldn't be possible without a more global concept known as MPP.
Data Engineering Design Patterns
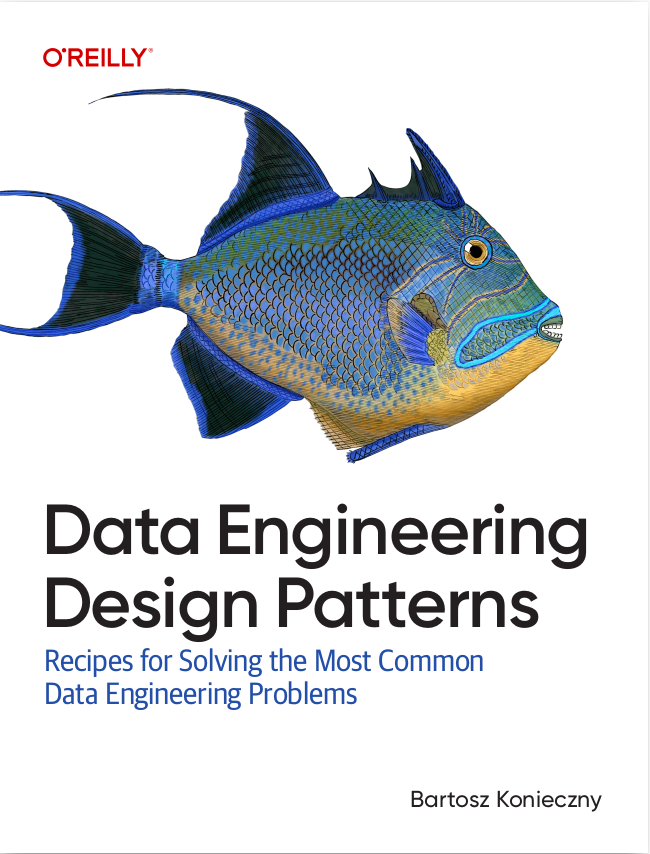
Looking for a book that defines and solves most common data engineering problems? I wrote
one on that topic! You can read it online
on the O'Reilly platform,
or get a print copy on Amazon.
I also help solve your data engineering problems 👉 contact@waitingforcode.com 📩
This post explains the meaning of that mysterious acronym. The first part defines the concepts without delving into details. The second section explains the main components of MPP architecture in the example of Azure SQL Data Warehouse.
Definition
MPP is a concept from parallel processing family. Before we focus on it, we'll see 2 other solutions from this category. The first one is called SMP for symmetric multiprocessors. In this shared-everything architecture multiple processors share memory and I/O. Thus, they're tightly coupled and it makes scaling them difficult. Another family member looks more like MPP and it's called SDC for shared-disk clusters. In this shared-something architecture the processors share disk but scaling them and providing high availability is easier than for SMP. However they require workload synchronization and balancing that makes them worse candidates than MPP.
MPP means Massively Parallel Processing. It's shared-nothing architecture used by popular data warehouse solutions as Amazon Redshift and Azure SQL Data Warehouse. Each processors has its own memory and external I/O. The cluster can be composed of a lot of commodity hardware nodes that makes the scaling cheaper than in 2 previous cases. However, this architecture also has a drawback. It requires pretty efficient communication layer to coordinate the work and communication of processors, e.g. during data shuffling for GROUP BY queries. But as told, scaling is easy thanks to resources separation.
Scalability is also influenced by the data organization. One of 3 strategies can be used:
- shared data and buffer - databases share buffers and data, i.e. the parallelization unit for this solution is the CPU. It's hard to scale.
- shared data - here the data is replicated across different nodes. It's quite good because there is no need to shuffle it but in the other side it's hard to keep it consistent. One of main drawbacks is the need of global locking mechanism ensuring that when one value is updated in given node, all other nodes read updated value.
- partitioning - seems to be the most scalable since it fits to shared-nothing concept. Chunks of dataset are stored in different, physically and logically separated places. Each database is responsible for one or more chunks that don't overlap.
We could summarize MPP in the following schema:
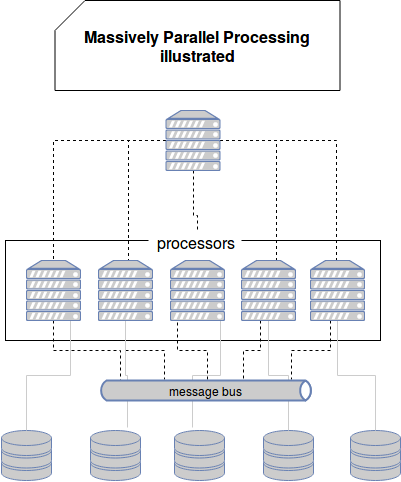
Azure Data Warehouse components
To see MPP implementation in details, let's analyze Azure SQL Data Warehouse architecture:
- control node - it's the entry point for all client requests. This controller receives queries and transforms them to their parallelizable format. The result of this transformation are queries executed by all concerned compute nodes.
- compute nodes - they're processing units. Beside their computation role, they're also responsible for a specific chunk(s) of data from the storage layer. Hence they execute the splitted query only on their subsets.
- communication layer - called Data Movement Service. Its main role consists on providing messaging facility between compute nodes. Sometimes the sharding strategy may not reflect the data needed for the query execution. In these cases some date will be shuffled, i.e. moved across the network. Communication layer facilitates this action.
- storage - as we've seen, data in the storage part is distributed in different shards. When compute nodes execute the query they all work on different shard. Thanks to that the storage is not anymore a contention point in the execution. Data in this storage layer can be organized according to different policies, based on:
- hashes - items are dispatched according to their computed hashes. The hash is computed from a distribution column(s). But not every column is a good candidate for the distribution and it should be chosen according to: distinctness, data skew, and the types of queries that run on the system. Otherwise data can be unevenly distributed and it can require shuffling and longer execution time.
- round-robin algorithm - data is distributed in round-robin manner, i.e. the first items goes to the 1st node, the 2nd to the 2nd, the 3rd to the 3rd and so on. When the last node receives the data, the distribution starts again with the 1st node in the storage part. It guarantees even data distribution but in the case of joins, it requires data shuffling that can slow down query execution.
- replication - here whole dataset is cached on each compute node, so the data doesn't need to be moved from storage layer to the compute nodes. However not every dataset is a good candidate for it. This strategy fits good for small tables that can be easily cached..
For the years divide-and-conquer strategy was one of solutions to speed up programs execution. It's especially true for Java world and ForkJoinPool. But with the arrival of Big Data this concept also made its proofs. One of the examples is Massively Parallel Processing architecture shown in this post. As described in the first section, it's based on a parallel execution of small chunks of a bigger query by processor units (commodity hardware nodes). The second part shown how this architecture is implemented in Azure cloud. We could clearly discover that separation of compute and storage helps to scale both layers independently and more efficiently in the case of growing load. However, this lack of coupling requires the existence of an extra layer to handle the communication between nodes, which adds some additional complexity to the whole architecture.
Consulting
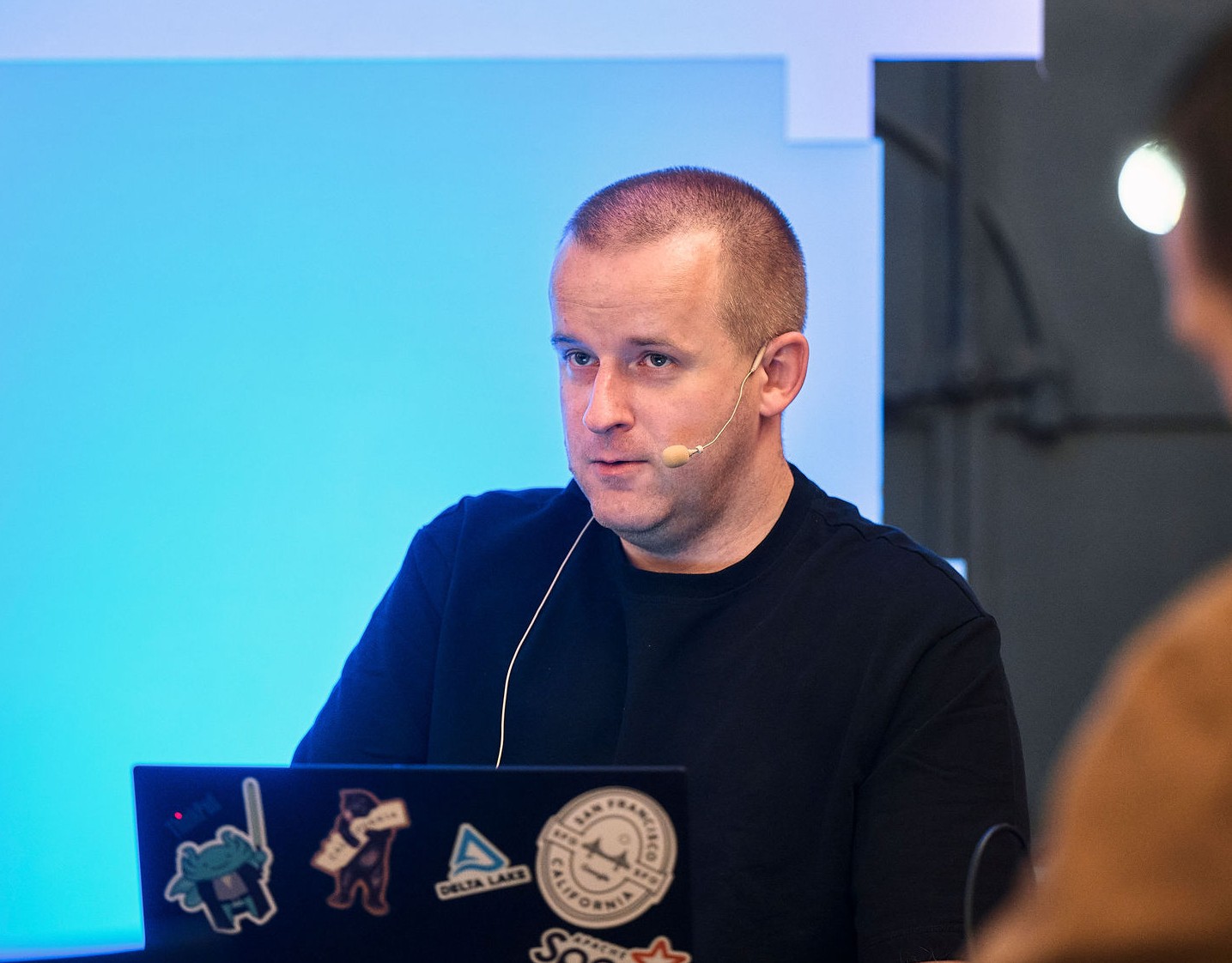
With nearly 16 years of experience, including 8 as data engineer, I offer expert consulting to design and optimize scalable data solutions.
As an O’Reilly author, Data+AI Summit speaker, and blogger, I bring cutting-edge insights to modernize infrastructure, build robust pipelines, and
drive data-driven decision-making. Let's transform your data challenges into opportunities—reach out to elevate your data engineering game today!
👉 contact@waitingforcode.com
đź”— past projects