It's time for the 2nd blog post about the shuffle readers. Recently, we discovered how Apache Spark fetches the shuffle blocks from local and remote hosts. Today, I would like to share with you the wrapping iterators. Sounds mysterious? It won't be if we start by looking at the iterators participating in the processing of shuffle block files.
Data Engineering Design Patterns
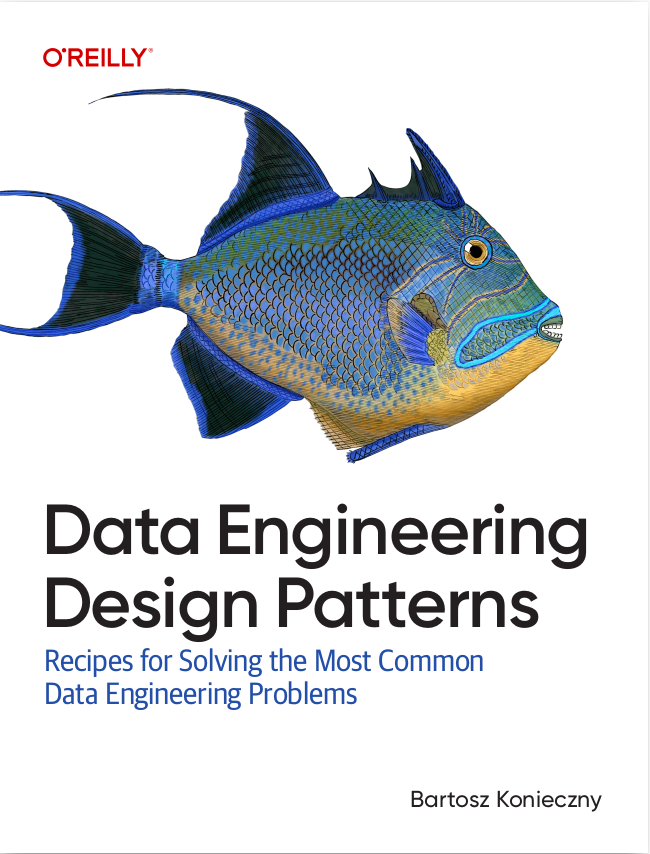
Looking for a book that defines and solves most common data engineering problems? I wrote
one on that topic! You can read it online
on the O'Reilly platform,
or get a print copy on Amazon.
I also help solve your data engineering problems 👉 contact@waitingforcode.com 📩
A high-level view of them looks like that:
private[spark] class BlockStoreShuffleReader[K, C]( // ... ) { override def read(): Iterator[Product2[K, C]] = { val wrappedStreams = new ShuffleBlockFetcherIterator( // ... ).toCompletionIterator val recordIter = wrappedStreams.flatMap { case (blockId, wrappedStream) => serializerInstance.deserializeStream(wrappedStream).asKeyValueIterator } val metricIter = CompletionIterator[(Any, Any), Iterator[(Any, Any)]]( // … val interruptibleIter = new InterruptibleIterator[(Any, Any)](context, metricIter) val resultIter = dep.keyOrdering match { case Some(keyOrd: Ordering[K]) => // ... CompletionIterator[Product2[K, C], Iterator[Product2[K, C]]](sorter.iterator, sorter.stop()) case None => aggregatedIter } ) resultIter match { case _: InterruptibleIterator[Product2[K, C]] => resultIter case _ => new InterruptibleIterator[Product2[K, C]](context, resultIter) }
It makes a lot of different iterators! Let me introduce them in the next section.
Shuffle iterators
The iterators you've seen in the snippets are:
- ShuffleBlockFetcherIterator - the first iterator of the chain is responsible for fetching local and remote shuffle block data. Its next() method returns a pair of (BlockId, InputStream), so a pair of block id and the associated shuffle data.
- CompletionIterator - the shuffle reader that converts the ShuffleBlockFetcherIterator into a CompletionIterator. This iterator simply decorates the fetching process with a ShuffleFetchCompletionListener that will trigger on task completion to free the fetcher resources:
private class ShuffleFetchCompletionListener(var data: ShuffleBlockFetcherIterator) extends TaskCompletionListener { override def onTaskCompletion(context: TaskContext): Unit = { if (data != null) { data.cleanup()locations(blocksByAddress) data = null } } def onComplete(context: TaskContext): Unit = this.onTaskCompletion(context) }
- NextIterator[(Any, Any)] (keyValueIterator) - the 3rd iterator involved in the workflow is a NextIterator. It processes the content of the shuffle blocks and deserializes it with the help of one of the available deserializers: JavaSerializerInstance, KryoSerializerInstance or UnsafeRowSerializerInstance.
Of course, Apache Spark SQL will use the UnsafeRowSerializerInstance and it's the single one I'll focus on here. The deserialization method returns a tuple defined as private[this] var rowTuple: (Int, UnsafeRow) = (0, row), where the row is a mutable representation of the currently read row:private[this] var row: UnsafeRow = new UnsafeRow(numFields) override def next(): (Int, UnsafeRow) = { if (rowBuffer.length < rowSize) { rowBuffer = new Array[Byte](rowSize) } ByteStreams.readFully(dIn, rowBuffer, 0, rowSize) row.pointTo(rowBuffer, Platform.BYTE_ARRAY_OFFSET, rowSize) rowSize = readSize() // ...
As you can see then, the tuple id will always be 0. - CompletionIterator - later, shuffle reader will initialize another CompletionIterator. This time, to manage the shuffle metrics with the number of read records.
- InterruptibleIterator - this iterator can be interrupted. Internally, every time it executes the hasNext method, it analyzes the task state and eventually kills the task hosting this iterator. It may happen if the speculative execution is enabled and one of the concurrently running task instances terminates.
- Iterator[(K, C)] or Iterator[(K, Nothing)] - the participation of these iterators is conditional. They'll participate in the shuffle-wrapping-chain only if the ShuffleDependency has an aggregator defined.
This should be never invoked for Apache Spark SQL since the aggregator is only defined in the low-level PairRDDFunctions. It's missing in ShuffleExchangeExec which is the physical node responsible for shuffle management in DataFrame API.
To stay consistent with the key-value type, Apache Spark SQL will cast the InterruptibleIterator instance to interruptibleIter.asInstanceOf[Iterator[Product2[K, C]]]. - CompletionIterator - this CompletionIterator will be sorted if the ShuffleDependency has an ordering expression. As for the aggregation, it won't happen in Apache Spark SQL.
- InterruptibleIterator - if the initial InterruptibleIterator transformed to a non-interruptible version, it's wrapped once again in the final step.
What instead of ShuffleDependency?
As I've mentioned, Apache Spark SQL doesn't use the metadata associated to the ShuffleDependency class to execute a sorting or aggregating post-shuffle operation.But how does it do that? The answer is hidden in the execution plan. Below you can see the plan generated for a groupByKey(...).mapGroups(...) operation. For you, which node is an unusual one?
== Physical Plan == *(2) SerializeFromObject [staticinvoke(class org.apache.spark.unsafe.types.UTF8String, StringType, fromString, input[0, java.lang.String, true], true, false) AS value#15] +- MapGroups org.apache.spark.sql.KeyValueGroupedDataset$$Lambda$1292/1990828041@342beaf6, value#12.toString, createexternalrow(id#7.toString, login#8.toString, StructField(id,StringType,true), StructField(login,StringType,true)), [value#12], [id#7, login#8], obj#14: java.lang.String +- *(1) Sort [value#12 ASC NULLS FIRST], false, 0 +- Exchange hashpartitioning(value#12, 10), ENSURE_REQUIREMENTS, [id=#15] +- AppendColumns com.waitingforcode.AggregatorExample$$$Lambda$1287/131837504@5866731, createexternalrow(id#7.toString, login#8.toString, StructField(id,StringType,true), StructField(login,StringType,true)), [staticinvoke(class org.apache.spark.unsafe.types.UTF8String, StringType, fromString, input[0, java.lang.String, true], true, false) AS value#12] +- LocalTableScan [id#7, login#8]
It's the highlighted Sort. Apache Spark SQL relies on the execution plan to provide a correct input to the post-shuffle operations. And the correct input for mapGroups requires the data to be sorted by the grouping key. It's explained in the comment of GroupedIterator class used in the physical execution:
/** * Iterates over a presorted set of rows, chunking it up by the grouping expression. Each call to * next will return a pair containing the current group and an iterator that will return all the * elements of that group. Iterators for each group are lazily constructed by extracting rows * from the input iterator. As such, full groups are never materialized by this class. * // … */
Shuffle reading picture
Sometimes the shuffle data must be materialized in an intermediate stage, as for the mapGroups example presented above. Sometimes it doesn't need to be, and it's the case of accumulative operations like count or sum. A plan for the former one is missing the intermediary node:
== Physical Plan == *(2) HashAggregate(keys=[value#19], functions=[count(1)], output=[key#24, count(1)#23L]) +- Exchange hashpartitioning(value#19, 10), ENSURE_REQUIREMENTS, [id=#50] +- *(1) HashAggregate(keys=[value#19], functions=[partial_count(1)], output=[value#19, count#30L]) +- *(1) Project [value#19] +- AppendColumns com.waitingforcode.AggregatorExample$$$Lambda$2599/1548162287@21f91efa, createexternalrow(id#7.toString, login#8.toString, StructField(id,StringType,true), StructField(login,StringType,true)), [staticinvoke(class org.apache.spark.unsafe.types.UTF8String, StringType, fromString, input[0, java.lang.String, true], true, false) AS value#19] +- LocalTableScan [id#7, login#8]
The fact of how shuffle blocks will be consumed depends then on the operation. The mapGroups will need to materialize them because of the sorting logic using spillable UnsafeExternalSorter:
/* 005 */ // codegenStageId=1 /* 006 */ final class GeneratedIteratorForCodegenStage1 extends org.apache.spark.sql.execution.BufferedRowIterator { /* 007 */ private Object[] references; /* 008 */ private scala.collection.Iterator[] inputs; /* 009 */ private boolean sort_needToSort_0; /* 010 */ private org.apache.spark.sql.execution.UnsafeExternalRowSorter sort_sorter_0; /* 019 */ public void init(int index, scala.collection.Iterator[] inputs) { /* 020 */ partitionIndex = index; /* 021 */ this.inputs = inputs; /* 022 */ sort_needToSort_0 = true; /* 023 */ sort_sorter_0 = ((org.apache.spark.sql.execution.SortExec) references[0] /* plan */).createSorter(); // .. /* 028 */ } /* 030 */ private void sort_addToSorter_0() throws java.io.IOException { /* 031 */ while ( inputadapter_input_0.hasNext()) { /* 032 */ InternalRow inputadapter_row_0 = (InternalRow) inputadapter_input_0.next(); /* 034 */ sort_sorter_0.insertRow((UnsafeRow)inputadapter_row_0); /* 035 */ // shouldStop check is eliminated /* 036 */ } /* 038 */ }
The count aggregation acts more like a fire-and-forget consumer. It processes the pre-shuffle partially aggregated rows and uses them to update the local aggregation buffers in ObjectAggregationIterator. It doesn't need to materialize the shuffle blocks beforehand.
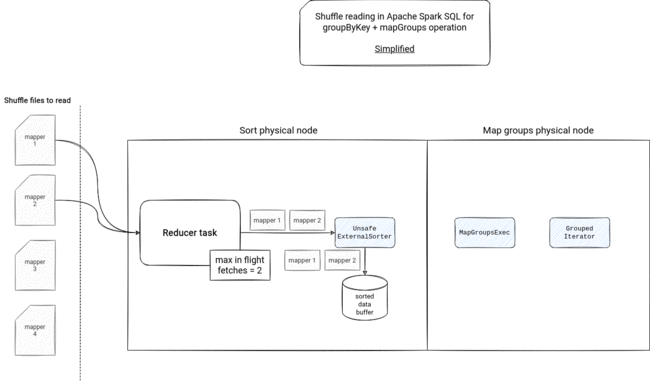
Reading sum-up
The shuffle readers series was quite long and believe me, I haven't covered all topics yet! However, we can already try to summarize the key points of the shuffle readers:
- fetching is not only remote - the instance of ShuffleBlockFetcherIterator class fetches shuffle files from local and remote nodes
- fetching is not an "all at once" action - when it comes to physically fetch the shuffle blocks across the network, the ShuffleBlockFetcherIterator has some limitations. There is a limit regarding pending fetch requests overall and per executor; there is also a limit for the memory size allocated to the shuffle data.
If the reducer task fetches more data than allowed, it will write it into a temporary file. If there is a too big network communication overhead, the fetcher will stop the reading and resume it when it will need the next shuffle block file - the fetching is based on multiple iterators - there are multiple iterators wrapping the initial ShuffleBlockFetcherIterator. Some of them has an important role in the execution, like the InterruptibleIterators used with the speculative execution, some of them seem to be only used in the RDD API because Apache Spark SQL doesn't set the ordering and aggregation values of the ShuffleDependency.
- physical plan instead of ShuffleDependency - instead of ShuffleDependency, Apache Spark creates physical nodes corresponding to the required action. For example, in the group by key + mapGroups operation, it will create a sort node to order the rows by the grouping key, so that they can be consumed by the GroupedIterator.
I hope that thanks to the recent blog posts, the Apache Spark shuffle part has less mysteries, but I also hope you have some questions to share that will maybe help to write the next blog posts of the series!
Consulting
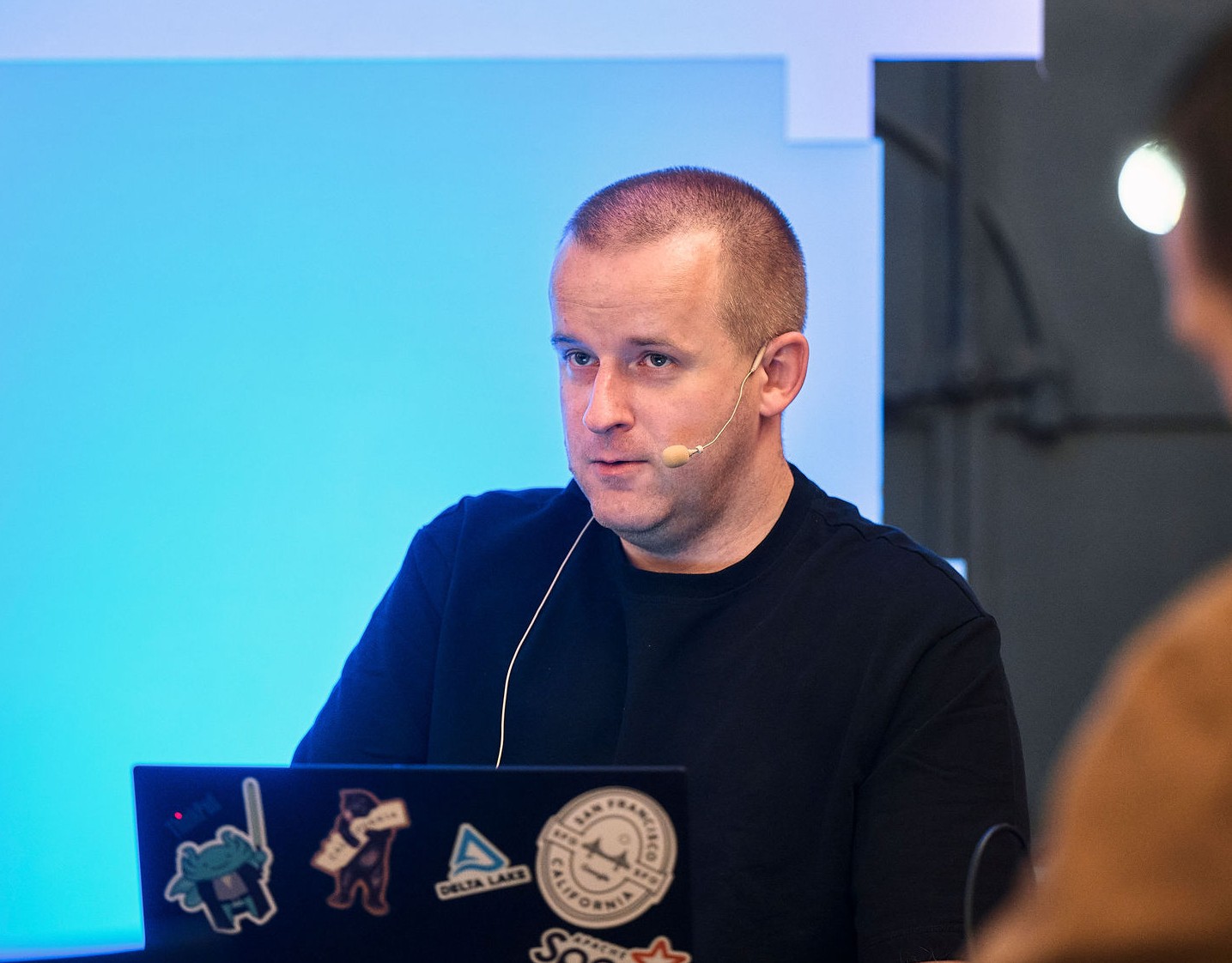
With nearly 16 years of experience, including 8 as data engineer, I offer expert consulting to design and optimize scalable data solutions.
As an O’Reilly author, Data+AI Summit speaker, and blogger, I bring cutting-edge insights to modernize infrastructure, build robust pipelines, and
drive data-driven decision-making. Let's transform your data challenges into opportunities—reach out to elevate your data engineering game today!
👉 contact@waitingforcode.com
đź”— past projects